Framework and experimental analysis of generalised surrogate-assisted particle swarm optimisation
International Journal of Computing Science and Mathematics(2022)
摘要
The paper develops a framework of generalised surrogate-assisted particle swarm optimisation (GS-PSO) to solve computationally expensive problems. To ensure the generalisation ability and optimisation accuracy of the algorithm, some researches about the factors of GS-PSO and selection of surrogates have been done. Some statistics indexes such as accuracy, robustness, and scalability are formulated to evaluate six popular metamodels, which can help to choose the proper surrogates for GS-PSO during the optimisation process. A series of simulation experiments are conducted based on some notable benchmark functions. The results show that GS-PSO with RBF is a robust surrogate-assisted algorithm for computationally expensive problems. Meanwhile, a proper combination of optimisers and surrogates can contribute to an improvement of GS-PSO for different optimisation problems.
更多查看译文
关键词
SAEAs, surrogate models, optimisation algorithms, performance indexes
AI 理解论文
溯源树
样例
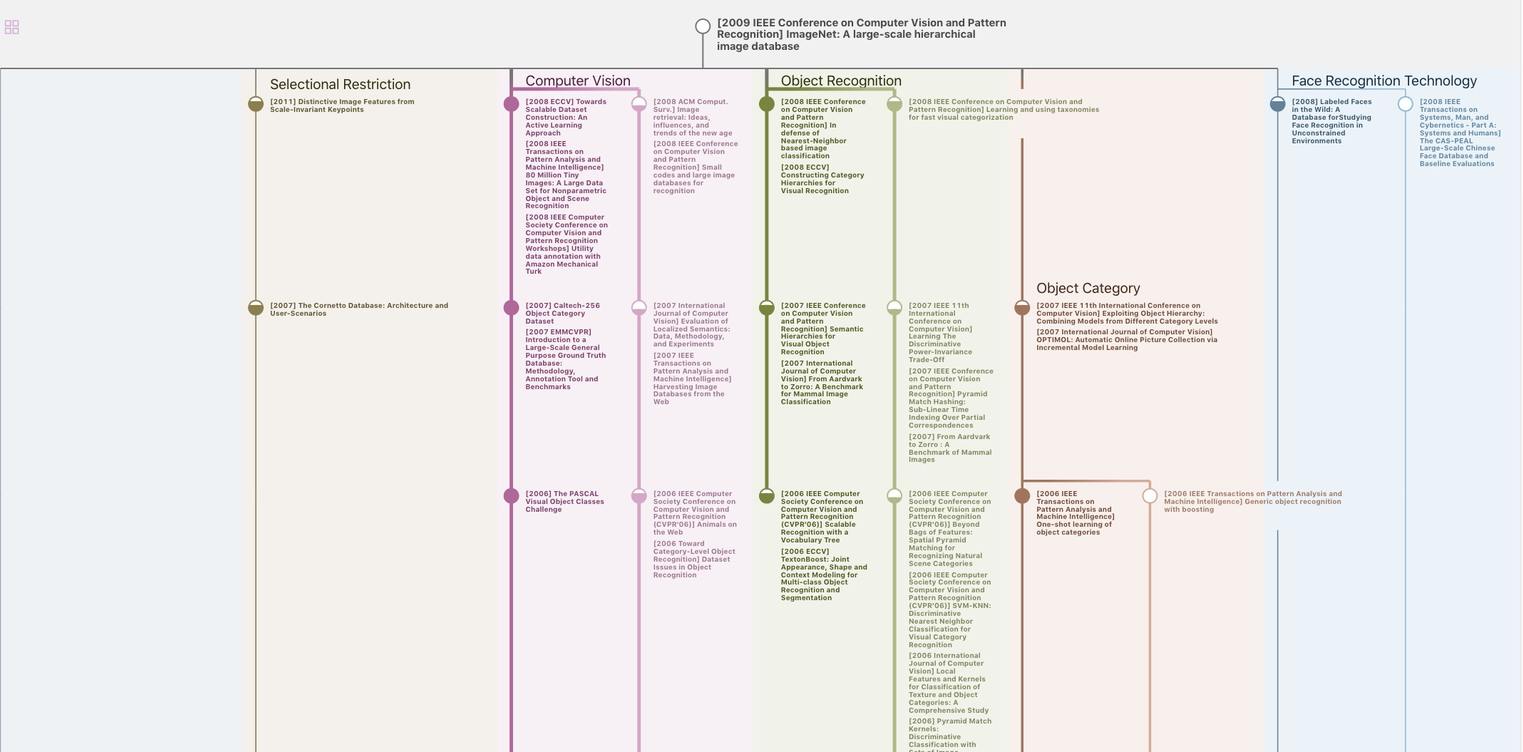
生成溯源树,研究论文发展脉络
Chat Paper
正在生成论文摘要