A TV regularisation sparse light field reconstruction model based on guided-filtering
Signal Processing: Image Communication(2022)
摘要
Obtaining and representing the 4D light field is important for a number of computer vision applications. Due to the high dimensionality, acquiring the light field directly is costly. One way to overcome this deficiency is to reconstruct the light field from a limited number of measurements. Existing approaches involve either a depth estimation process or require a large number of measurements to obtain high-quality reconstructed results. In this paper, we propose a total variation (TV) regularisation sparse model with the alternating direction method of multipliers (ADMM) based on guided filtering, which addresses this depth-dependence problem with only a few measurements. As one of the sparse optimisation methods, TV regularisation based on ADMM is well suited to solve ill-posed problems such as this. Moreover, guided filtering has good edge-preserving smoothing properties, which can be incorporated into the light field reconstruction process. Therefore, high precision light field reconstruction is established with our model. Specifically, the updated image in the iteration step contains the guidance image, and an initialiser for the least squares method using a QR factorisation (LSQR) algorithm is involved in one of the subproblems. The model outperforms other methods in both visual assessments and objective metrics – in simulation experiments from synthetic data and photographic data using produced focal stacks from light field contents – and it works well in experiments using captured focal stacks. We also show a further application for arbitrary refocusing by using the reconstructed light field.
更多查看译文
关键词
Light field reconstruction,Total variation regularisation,The guided filter
AI 理解论文
溯源树
样例
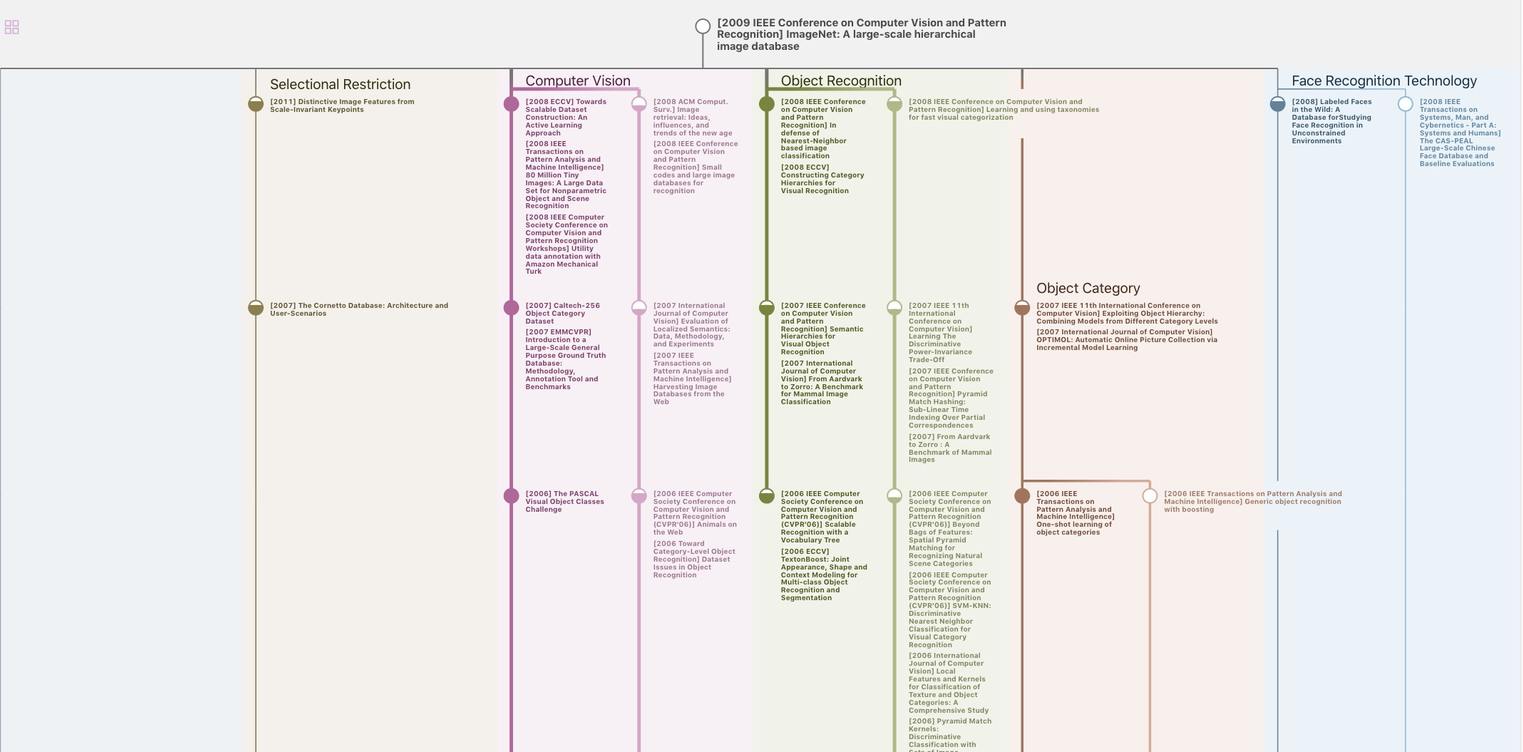
生成溯源树,研究论文发展脉络
Chat Paper
正在生成论文摘要