Non-intrusive Speech Quality Assessment based on Tucker Decomposition and Deep Neural Network
2019 IEEE International Conference on Signal, Information and Data Processing (ICSIDP)(2019)
摘要
In this paper, we present a new speech quality assessment method to estimate the quality of degraded speech without the reference speech. The traditional non-intrusive assessment methods cannot meet the requirement of high consistency with subjective results owing to the lack of original reference signals. To solve these issues, deep belief network is trained to produce pseudo-reference speech signal of degraded speech. Then the pseudo-reference speech and the degraded speech are modeled by tensor analysis to obtained features which is used to calculate feature differences. The feature differences are mapped to speech quality score using support vector regression. Experiments are conducted in a wideband dataset containing various degraded speech signals and subjective listening scores. When compared with the Gaussian Mixture Model method and deep belief network method, the proposed method brings about a higher correlation coefficient between predicted scores and subjective scores.
更多查看译文
关键词
non-intrusive speech quality assessment,deep belief network,tensor analysis,support vector regression
AI 理解论文
溯源树
样例
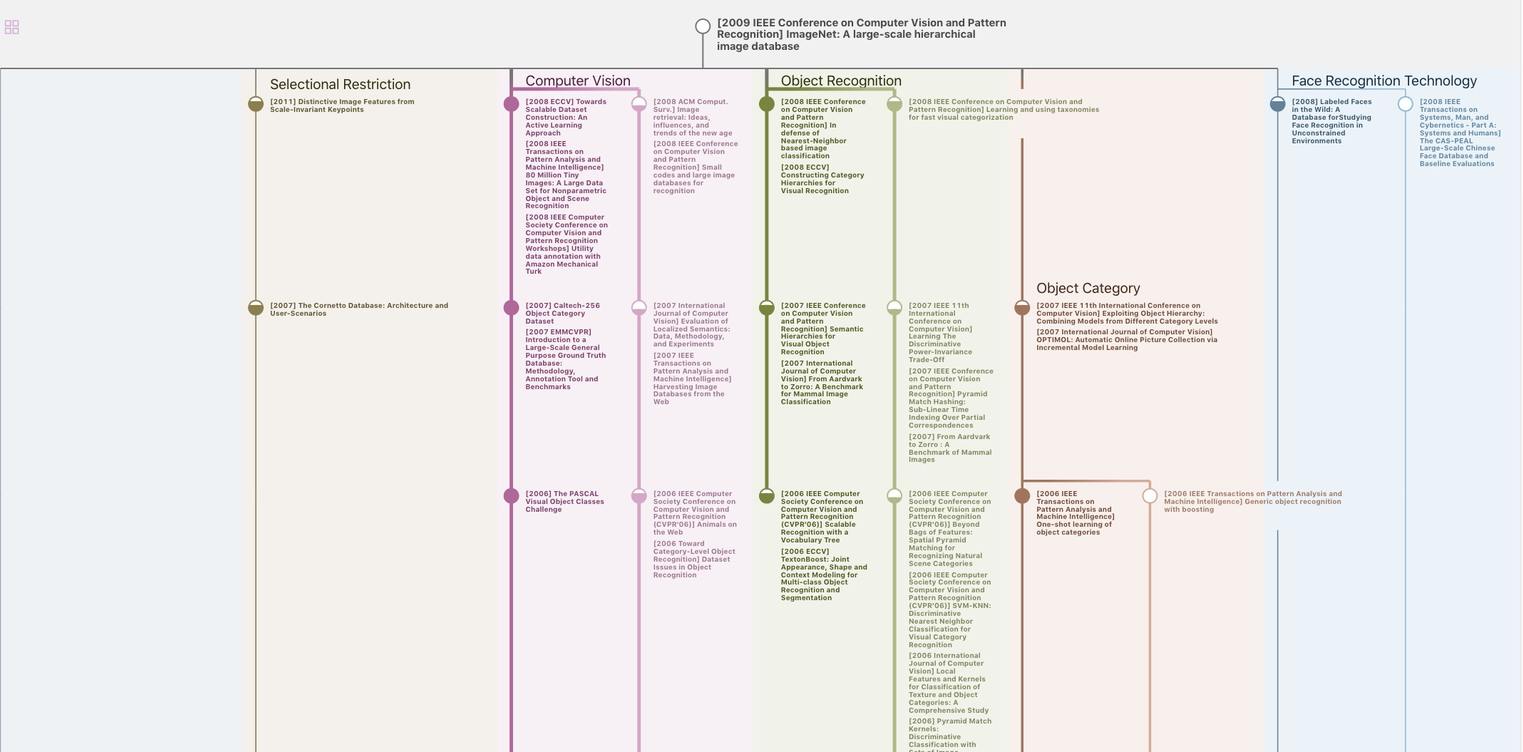
生成溯源树,研究论文发展脉络
Chat Paper
正在生成论文摘要