Synthetic Ground Truth Generation of an Electricity Consumption Dataset
2022 International Conference on Smart Energy Systems and Technologies (SEST)(2022)
摘要
The training of supervised Machine Learning (ML) and Artificial Intelligence (AI) algorithms is strongly affected by the goodness of the input data. To this end, this paper proposes an innovative synthetic ground truth generation algorithm. The methodology is based on applying a data reduction with Symbolic Aggregate Approximation (SAX). In addition, a Classification And Regression Tree (CART) is employed to identify the best granularity of the data reduction. The proposed algorithm has been applied to telecommunication (TLC) sites dataset by analyzing their electricity consumption patterns. The presented approach substantially reduced the dispersion of the dataset compared to the raw dataset, thus reducing the effort required to train the supervised algorithms.
更多查看译文
关键词
Approximation,Classification,Clustering,Symbols Aggregated,Synthetic Ground Truth
AI 理解论文
溯源树
样例
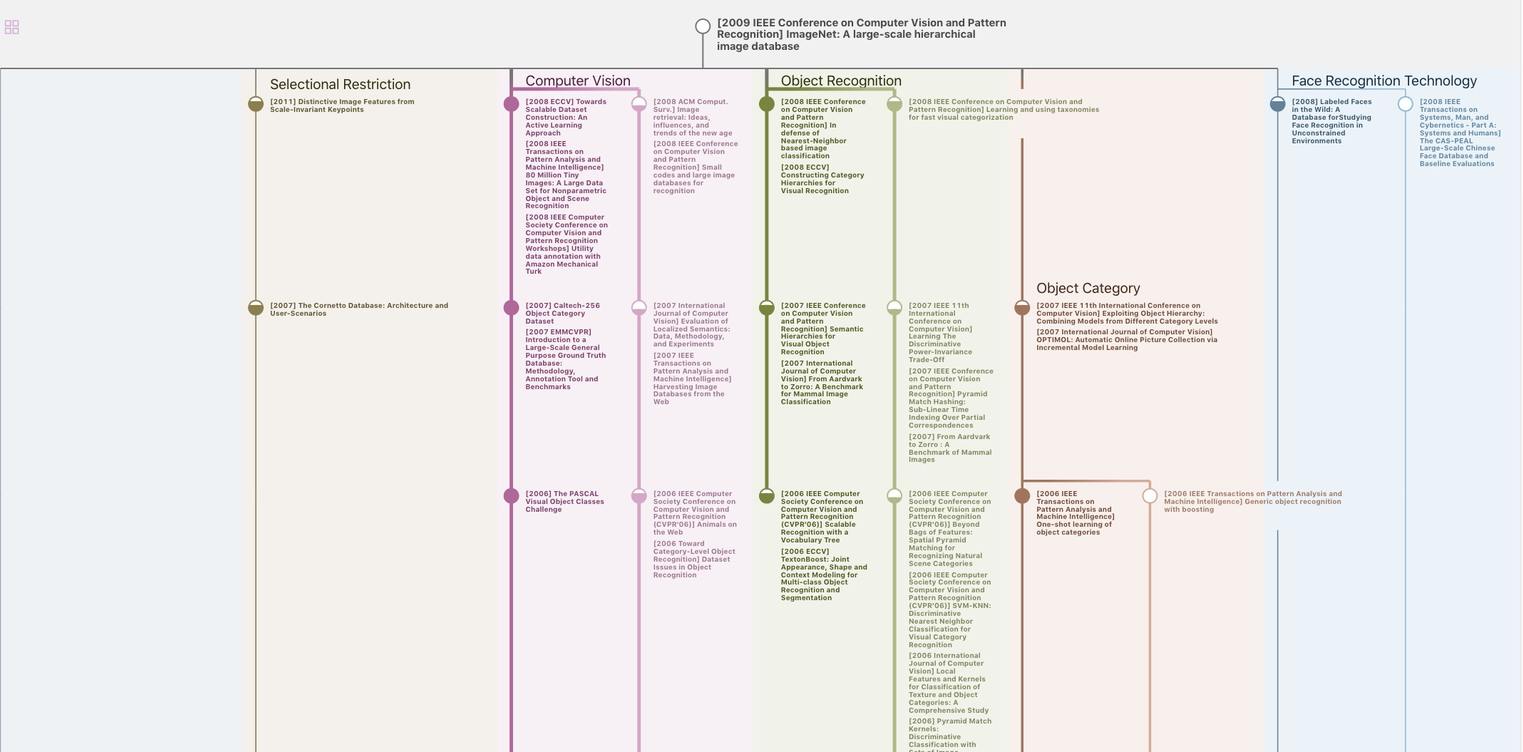
生成溯源树,研究论文发展脉络
Chat Paper
正在生成论文摘要