Explanation-by-Example Based on Item Response Theory.
BRACIS (1)(2022)
摘要
Intelligent systems that use Machine Learning classification algorithms are increasingly common in everyday society. However, many systems use black-box models that do not have characteristics that allow for self-explanation of their predictions. This situation leads researchers in the field and society to the following question: How can I trust the prediction of a model I cannot understand? In this sense, XAI emerges as a field of AI that aims to create techniques capable of explaining the decisions of the classifier to the end-user. As a result, several techniques have emerged, such as Explanation-by-Example, which has a few initiatives consolidated by the community currently working with XAI. This research explores the Item Response Theory (IRT) as a tool to explaining the models and measuring the level of reliability of the Explanation-by-Example approach. To this end, four datasets with different levels of complexity were used, and the Random Forest model was used as a hypothesis test. From the test set, 83.8% of the errors are from instances in which the IRT points out the model as unreliable.
更多查看译文
关键词
response,theory,explanation-by-example
AI 理解论文
溯源树
样例
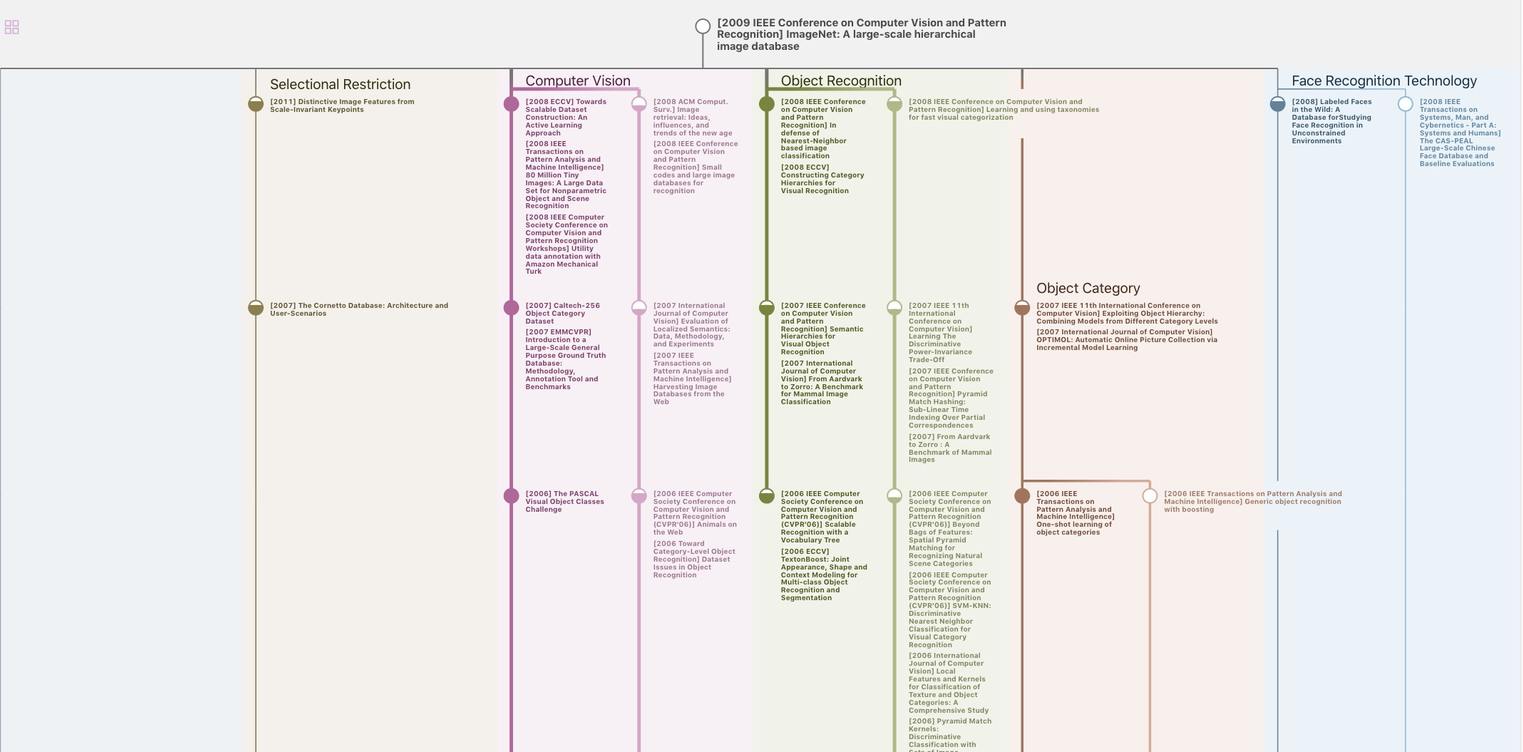
生成溯源树,研究论文发展脉络
Chat Paper
正在生成论文摘要