RAP: Risk-Aware Prediction for Robust Planning
arxiv(2023)
摘要
Robust planning in interactive scenarios requires predicting the uncertain future to make risk-aware decisions. Unfortunately, due to long-tail safety-critical events, the risk is often under-estimated by finite-sampling approximations of probabilistic motion forecasts. This can lead to overconfident and unsafe robot behavior, even with robust planners. Instead of assuming full prediction coverage that robust planners require, we propose to make prediction itself risk-aware. We introduce a new prediction objective to learn a risk-biased distribution over trajectories, so that risk evaluation simplifies to an expected cost estimation under this biased distribution. This reduces the sample complexity of the risk estimation during online planning, which is needed for safe real-time performance. Evaluation results in a didactic simulation environment and on a real-world dataset demonstrate the effectiveness of our approach. The code and a demo are available.
更多查看译文
关键词
planning,prediction,risk-aware
AI 理解论文
溯源树
样例
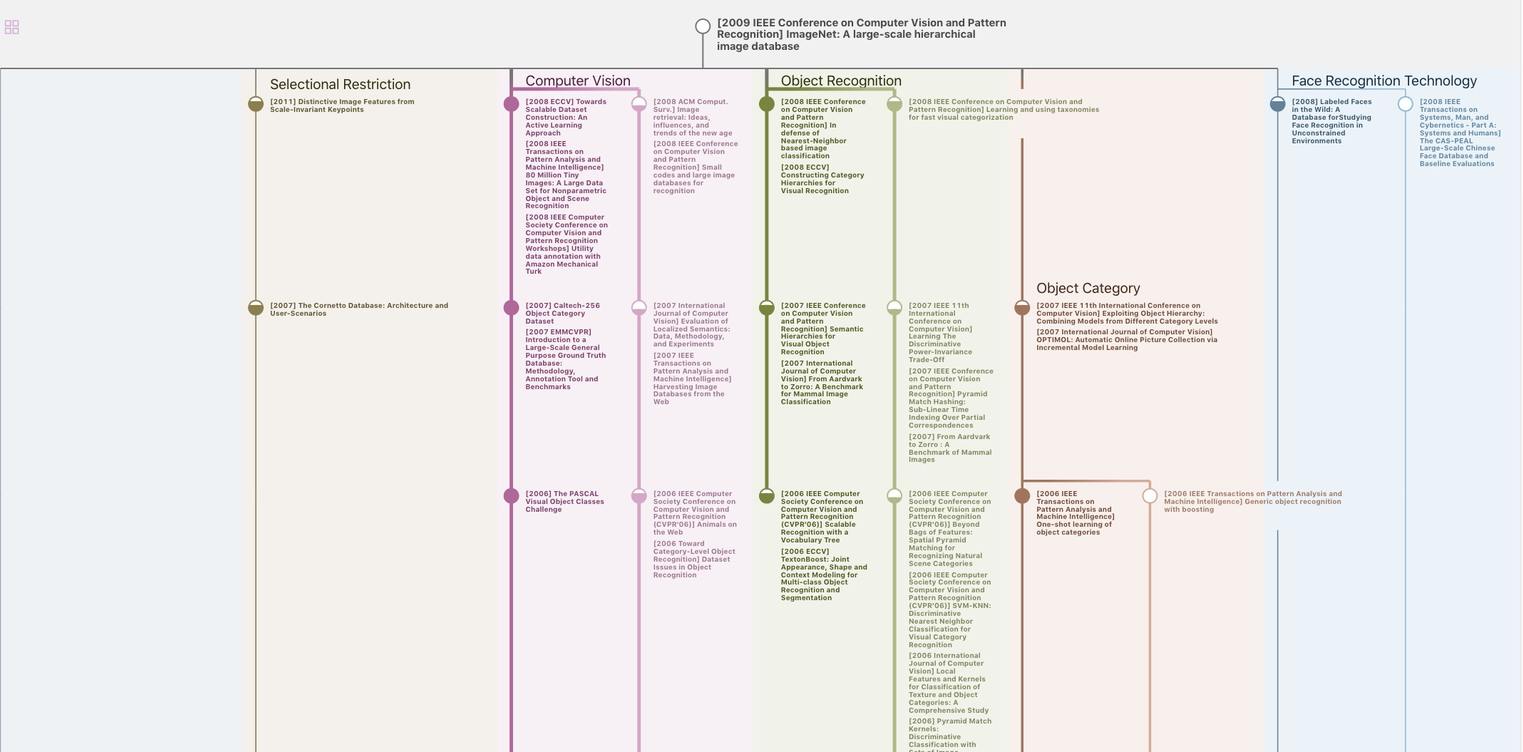
生成溯源树,研究论文发展脉络
Chat Paper
正在生成论文摘要