Learning over time using a neuromorphic adaptive control algorithm for robotic arms
arxiv(2022)
摘要
In this paper, we explore the ability of a robot arm to learn the underlying operation space defined by the positions (x, y, z) that the arm's end-effector can reach, including disturbances, by deploying and thoroughly evaluating a Spiking Neural Network SNN-based adaptive control algorithm. While traditional control algorithms for robotics have limitations in both adapting to new and dynamic environments, we show that the robot arm can learn the operational space and complete tasks faster over time. We also demonstrate that the adaptive robot control algorithm based on SNNs enables a fast response while maintaining energy efficiency. We obtained these results by performing an extensive search of the adaptive algorithm parameter space, and evaluating algorithm performance for different SNN network sizes, learning rates, dynamic robot arm trajectories, and response times. We show that the robot arm learns to complete tasks 15% faster in specific experiment scenarios such as scenarios with six or nine random target points.
更多查看译文
关键词
neuromorphic adaptive control algorithm,adaptive control,learning,arms
AI 理解论文
溯源树
样例
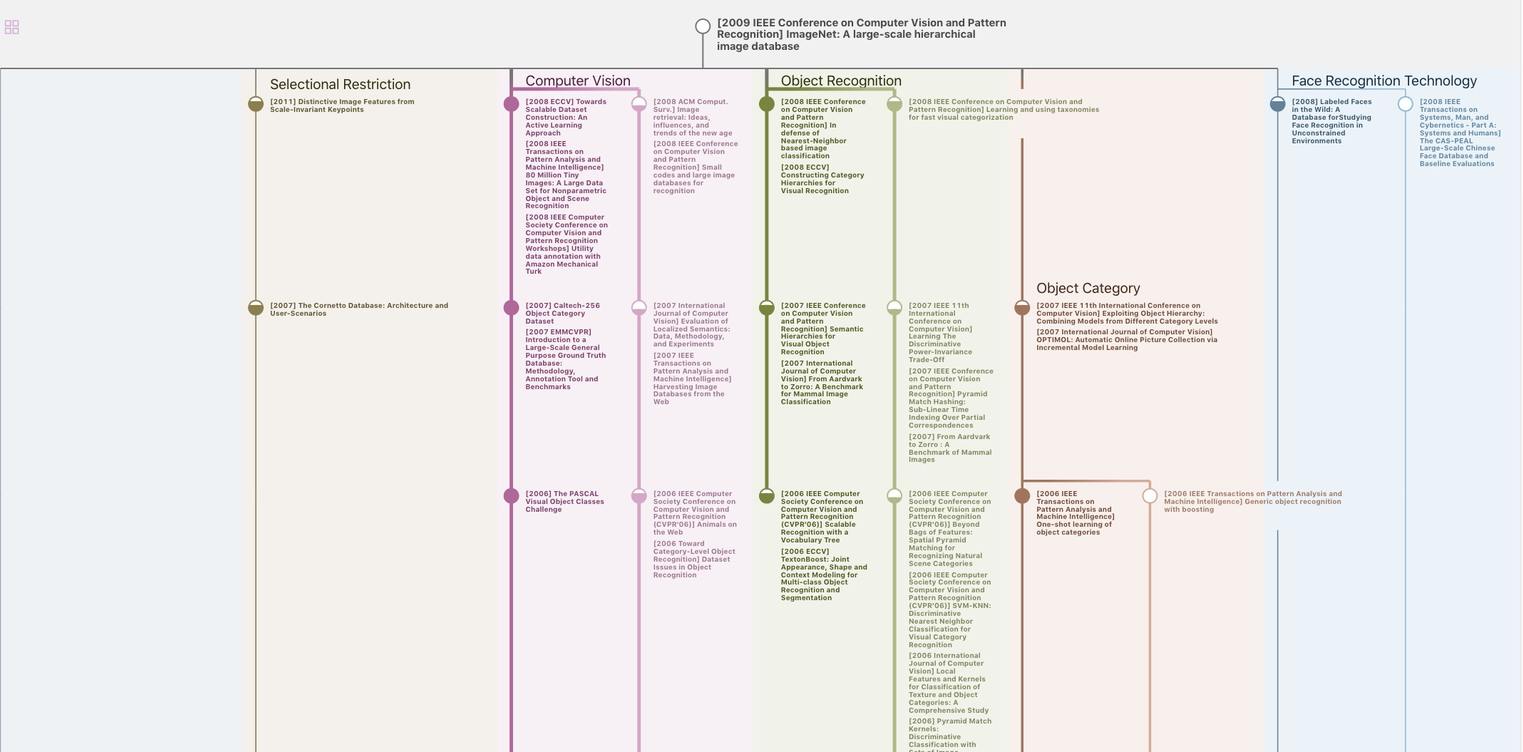
生成溯源树,研究论文发展脉络
Chat Paper
正在生成论文摘要