Local Intrinsic Density Based Community Detection Using Branch-and-Bound and Minimum Spanning Tree
Systems and Soft Computing(2022)
摘要
Community detection in complex networks has emerged as a fundamental research area during the last decade. Traditional community detection is primarily based on static techniques, which are neither practicable regarding limited resources nor appropriate for the evolving complex networks. Dynamically updating the community structures, getting accurate and real-time results are vital constraints for analyzing evolving networks, hence in our research, we take these possibilities into consideration. Our work proposes a novel community detection approach BBmst inspired by the original branch-and-bound (BB) concept for label propagation and a Minimum Spanning Tree (MST) to measure the dissimilarity between nodes in graphs. These proposed methods consist of four phases: finding of the local intrinsic density called core or granule of the community, label propagation with BB, an MST is employed to segregate the detected communities and finally a modularity-based community merging is performed. The performance of our proposed method is assessed against a few well-known community detectors on real-world social networks concerning various validity measures. Results demonstrated our proposed method superiority over current community detectors and showed a considerable trade-off between cluster accuracy and quality.
更多查看译文
关键词
Minimum spanning tree,Branch-and-bound,Community detection,Local density,Tree structure,Dynamic social networks,Modularity
AI 理解论文
溯源树
样例
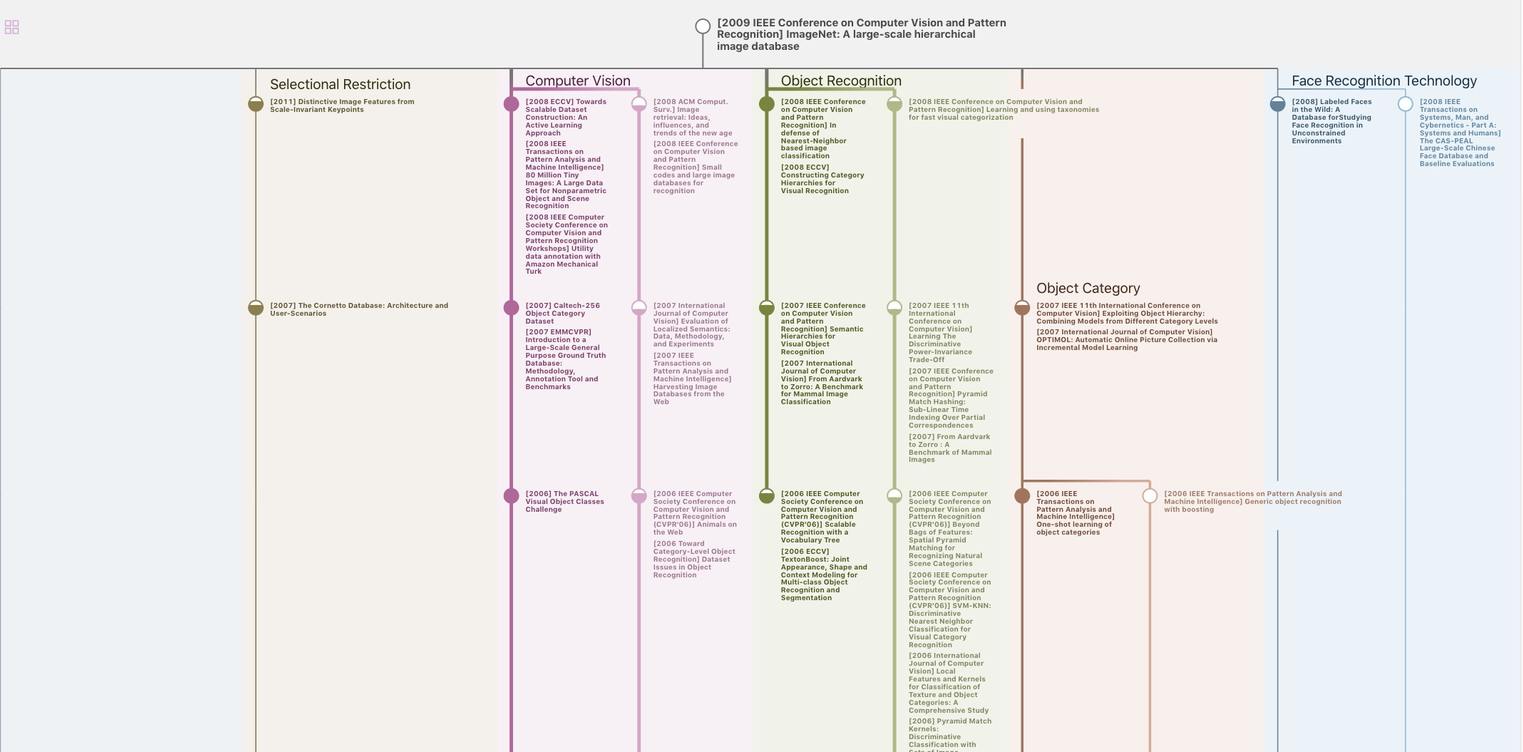
生成溯源树,研究论文发展脉络
Chat Paper
正在生成论文摘要