Selective Kernel and Spatial Grouping Attention Network for Occluded Pedestrian Detection
2022 8th International Conference on Virtual Reality (ICVR)(2022)
摘要
Pedestrian detection has achieved significant progress on computer vision tasks in recent years. Most pedestrian detection methods employ deep convolutional neural networks to extract abstract features. However, convolution is a local operation that relies on down-sampling to obtain high-level semantic features, which cannot extract global image information or selectively focus on the input features. Furthermore, since the majority of the pedestrian's body is invisible under severe occlusion, the performance of existing pedestrian detectors remains further improvement. To this end, we propose a novel network with selective kernel and spatial grouping attention, i.e., SKGNet, for the occluded pedestrian detection task. Specifically, we first introduce a lightweight attention module, selective kernel and spatial grouping attention (SKG), which is embedded in the SKGNet's feature extraction backbone. The SKG module combines the properties of the selective kernel (SK) and spatial grouping enhancement (SGE) mechanisms to extract more critical features and improve the expressive ability of feature maps, ultimately improving the detection performance of the network. Moreover, we propose a mask-guided (MG) module to modulate full-body features, which can highlight the visible part of pedestrians while suppressing the occlusion part, thereby significantly improving occlusion detection performance. Extensive experiments show that SKGNet outperforms the existing advanced methods on the CityPersons dataset without excessive extra parameters and computations.
更多查看译文
关键词
deep learning,convolutional neural network,pedestrian detection,attention mechanism
AI 理解论文
溯源树
样例
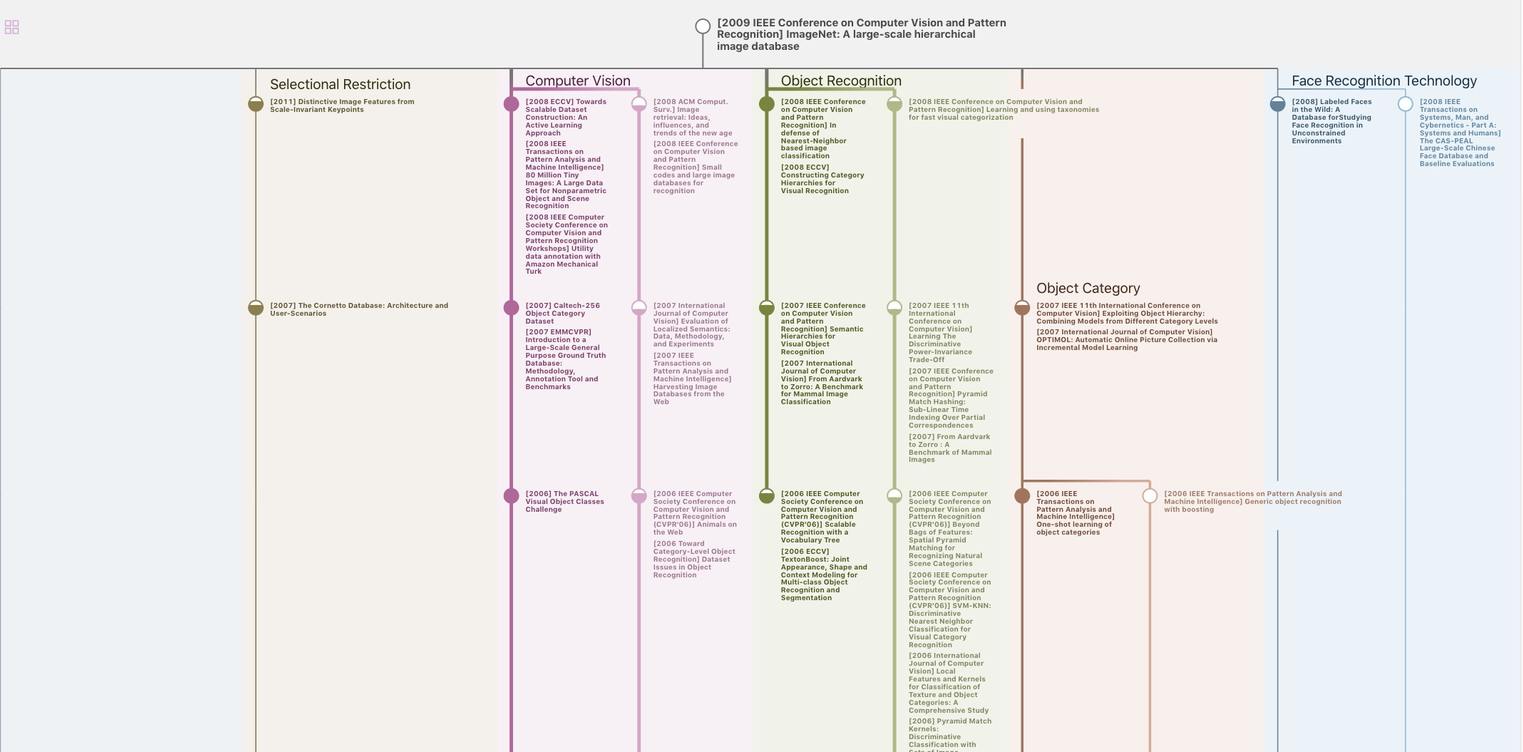
生成溯源树,研究论文发展脉络
Chat Paper
正在生成论文摘要