RA-Swin: A RefineNet Based Adaptive Model Using Swin Transformer for Monocular Depth Estimation
2022 8th International Conference on Virtual Reality (ICVR)(2022)
摘要
Transformer-based deep learning networks have achieved extraordinary success in the field of natural language processing (NLP) in recent years. However, Transformer faces practical challenges due to the differences in the fields of NLP and visual dense prediction. This paper employs a layered Transformer as a feature extraction encoder for monocular depth estimation to overcome these differences. The encoder takes the original image size as input and performs self-attention computation on non-overlapping local regions of the feature map by shifting the window. It enables the cross-window information to interact. Different variants of the encoder are followed by an adaptable decoder based on the spatial resampling module and Refine Net. The adaptable decoder can better fuse the multi-scale output features of the encoder while keeping the number of parameters low, combined with skip connections. Experiments show that the encoder-decoder structure in this paper, fine-tuned on the NYU Depth v2 dataset, can also yield substantial improvements for monocular depth estimation. The experimental results show that compared with the current advanced Transformer model DPT -Hybrid, the root mean square error (RMS) of the Swin-B and Swin-L based models are reduced by 1.12% and 2.97%, achieving better depth estimation results.
更多查看译文
关键词
Swin transformer,monocular vision,depth estimation,transfer learning
AI 理解论文
溯源树
样例
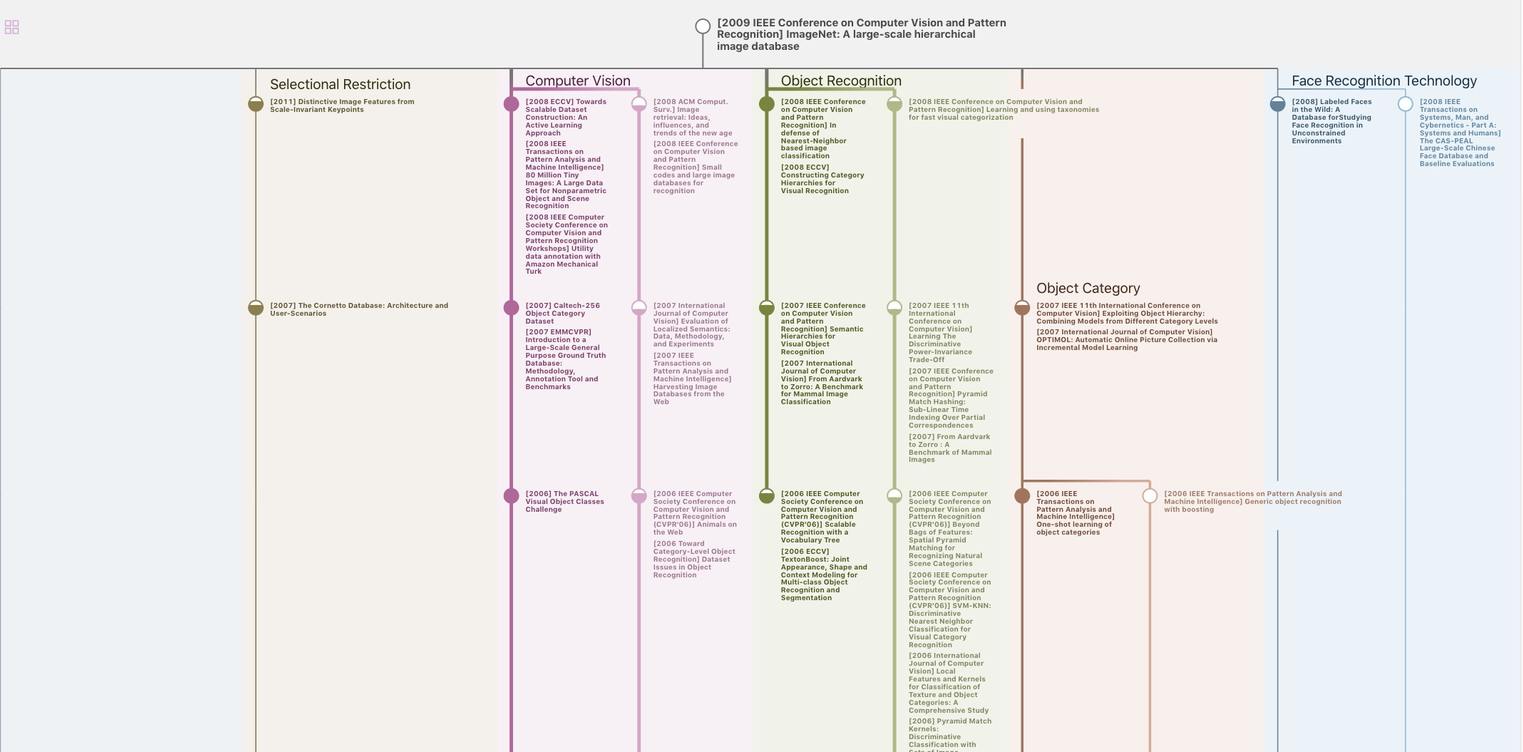
生成溯源树,研究论文发展脉络
Chat Paper
正在生成论文摘要