Deep-learning informed Kalman filtering for priori-free and real-time hemodynamics extraction in functional near-infrared spectroscopy
BIOMEDICAL OPTICS EXPRESS(2022)
摘要
Separation of the physiological interferences and the neural hemodynamics has been a vitally important task in the realistic implementation of functional near-infrared spectroscopy (fNIRS). Although many efforts have been devoted, the established solutions to this issue additionally rely on priori information on the interferences and activation responses, such as time-frequency characteristics and spatial patterns, etc., also hindering the realization of real-time. To tackle the adversity, we herein propose a novel priori-free scheme for real-time physiological interference suppression. This method combines the robustness of deep-leaning -based interference characterization and adaptivity of Kalman filtering: a long short-term memory (LSTM) network is trained with the time-courses of the absorption perturbation baseline for interferences profiling, and successively, a Kalman filtering process is applied with reference to the noise prediction for real-time activation extraction. The proposed method is validated using both simulated dynamic data and in-vivo experiments, showing the comprehensively improved performance and promisingly appended superiority achieved in the purely data-driven way.(c) 2022 Optica Publishing Group under the terms of the Optica Open Access Publishing Agreement
更多查看译文
关键词
spectroscopy,kalman filtering,deep-learning deep-learning,priori-free,real-time,near-infrared
AI 理解论文
溯源树
样例
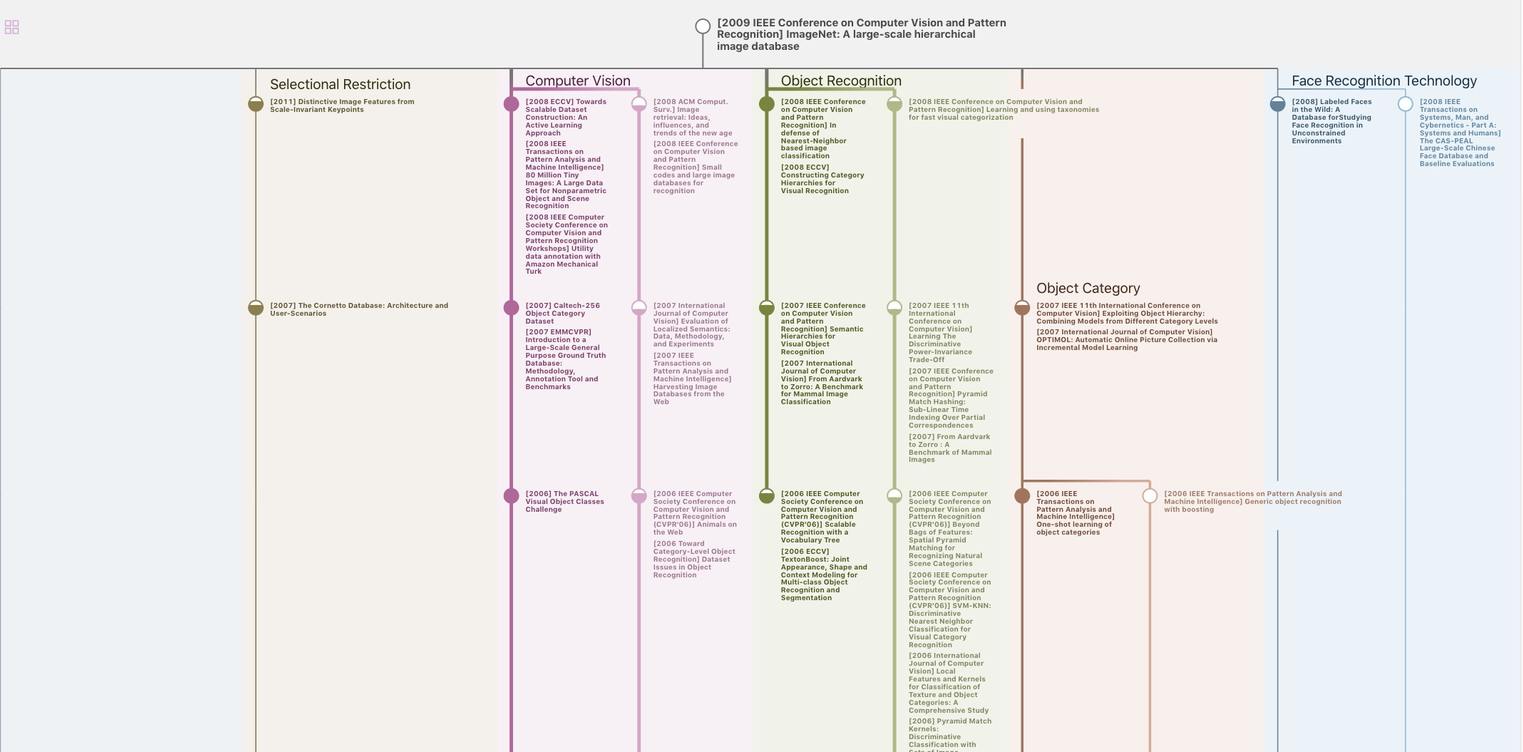
生成溯源树,研究论文发展脉络
Chat Paper
正在生成论文摘要