Active fault detection using time and frequency diagnostic features for electrical machine
11th International Conference on Power Electronics, Machines and Drives (PEMD 2022)(2022)
摘要
Accurate fault detection in electrical motors is essential for ensuring system reliability and safety. This paper presents an effective diagnosis method for the fault detection of permanent magnet synchronous motors (PMSMs) operating at three different faults over a wide speed and load range. The proposed fault-detection method is based on the extracted features of stator currents from the time and frequency domains. The extracted features are then fed into an ensemble subspace discriminant tree machine-learning algorithm to classify the different types of faults. To validate the efficiency of the proposed approach, experimental tests were conducted on PMSMs operating under different speeds, loads, and fault conditions. The proposed method achieved highly accurate prediction results of 99.6% and could classify five different motor states, including two interturn short-circuit fault states.
更多查看译文
关键词
fault conditions,highly accurate prediction results,different motor states,interturn short-circuit fault states,active fault detection,frequency diagnostic features,electrical machine,accurate fault detection,electrical motors,system reliability,effective diagnosis method,permanent magnet synchronous motors,wide speed,load range,fault-detection method,stator currents,frequency domains,machine-learning algorithm,PMSM,time diagnostic features,extracted features,short-circuit fault states,subspace discriminant tree
AI 理解论文
溯源树
样例
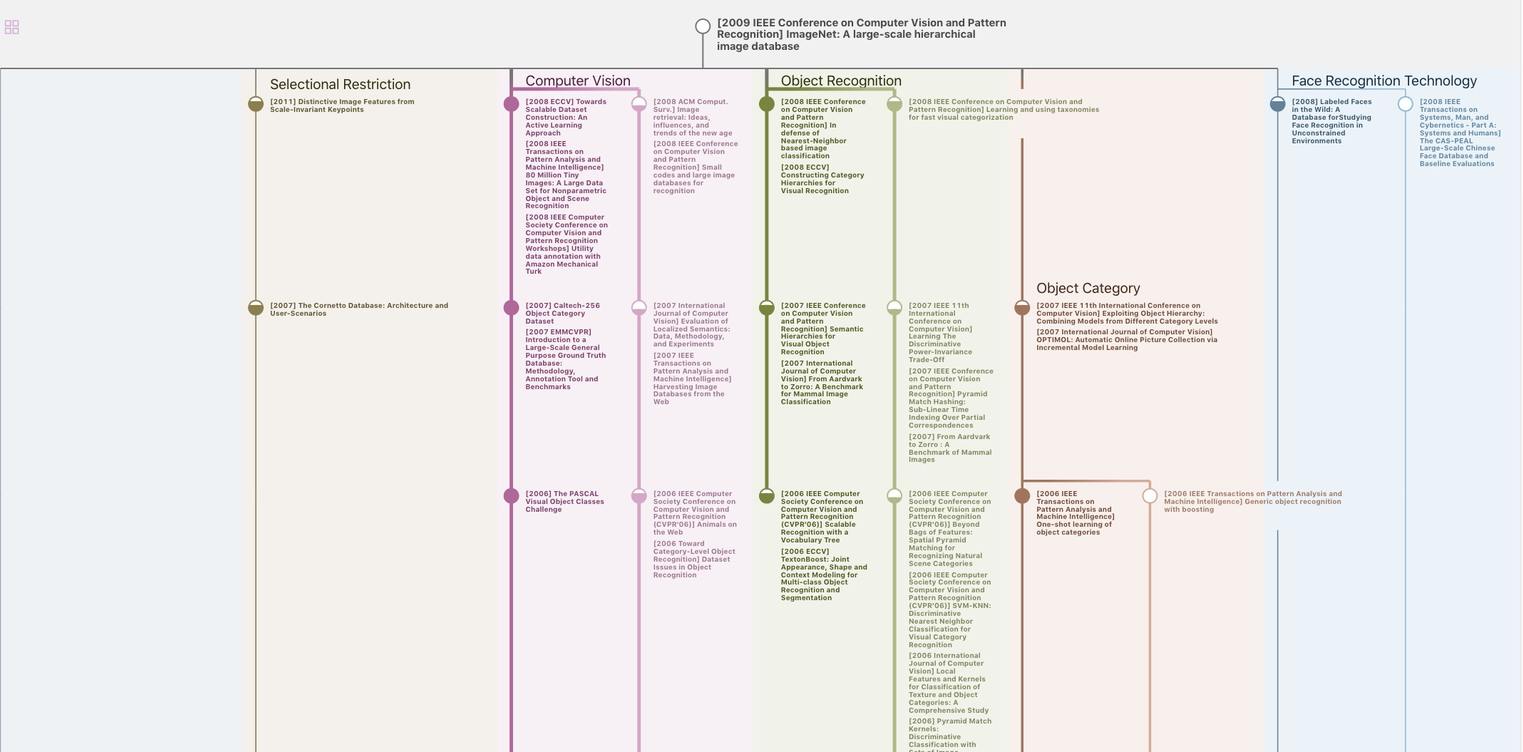
生成溯源树,研究论文发展脉络
Chat Paper
正在生成论文摘要