A deep neural network and Bayesian method based framework for all-terminal network reliability estimation considering degradation.
Reliab. Eng. Syst. Saf.(2023)
摘要
Most research on network reliability has considered links to have a binary state, i.e., functioning or failed, whereas nodes are considered flawless. In a more realistic scenario, both links and nodes might fail or may exhibit degradation behavior before failing. This study develops a framework to estimate the all-terminal reliability of a network that considers the degradation and probability of failure of all nodes and links in a network. Unlike previous works on network reliability that considered constant reliability for links, this paper considers the reliability of links, nodes, and the network as functions of time. In the proposed framework, the Bayesian methods (BM) are employed to estimate the reliability of links and nodes as functions of time considering degradation data. Due to the complexity of the all-terminal reliability problem, and to get fast estimations of the reliability of a network, an integration of Monte Carlo (MC) and Deep Neural Networks (DNNs) is proposed. The proposed MC algorithm can estimate the network reliability for given nodes and links reliability values. To speed up the calculation, a DNN model is integrated into the framework, thus enabling accurate and fast estimation of network reliability for given link and node reliability values. The DNN accuracy, based on the RMSE (0.01460), outperforms previous traditional artificial neural network (ANN) approaches. Moreover, the DNN model takes 0.3 ms to compute the reliability for any given links and reliability values. The proposed framework can provide not only reliability point estimates but also credible intervals. Finally, we take advantage of Bayesian methods to integrate new data into the framework as they become available. The framework uses the new data to refine and further update the degradation model parameters and the prediction of the reliability of links, nodes, and the network. The proposed methodology has been demonstrated with the real-world network topology Ion (125 nodes, 150 links) with actual degradation data.
更多查看译文
关键词
All-terminal network reliability,Bayesian methods,Credible intervals,Degradation,Monte Carlo,Deep neural networks
AI 理解论文
溯源树
样例
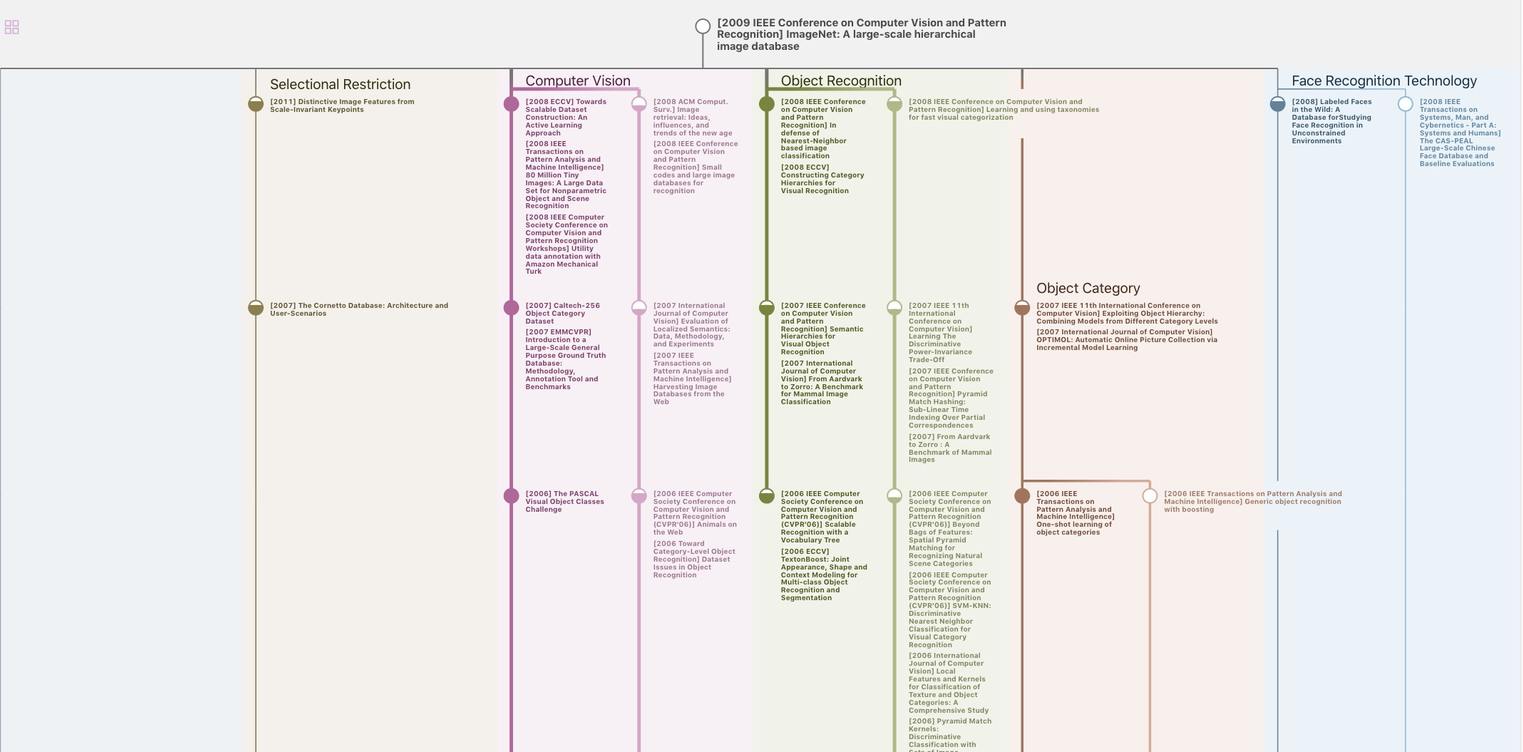
生成溯源树,研究论文发展脉络
Chat Paper
正在生成论文摘要