A Partially Functional Linear Modeling Framework for Integrating Genetic, Imaging, and Clinical Data
arxiv(2023)
摘要
This paper is motivated by the joint analysis of genetic, imaging, and clinical (GIC) data collected in the Alzheimer's Disease Neuroimaging Initiative (ADNI) study. We propose a regression framework based on partially functional linear regression models to map high-dimensional GIC-related pathways for Alzheimer's Disease (AD). We develop a joint model selection and estimation procedure by embedding imaging data in the reproducing kernel Hilbert space and imposing the L0 penalty for the coefficients of genetic variables. We apply the proposed method to the ADNI dataset to identify important features from tens of thousands of genetic polymorphisms (reduced from millions using a preprocessing step) and study the effects of a certain set of informative genetic variants and the baseline hippocampus surface on thirteen future cognitive scores measuring different aspects of cognitive function. We explore the shared and different heritability patterns of these cognitive scores. Analysis results suggest that both the hippocampal and genetic data have heterogeneous effects on different scores, with the trend that the value of both hippocampi is negatively associated with the severity of cognition deficits. Polygenic effects are observed for all thirteen cognitive scores. The well-known APOE4 genotype only explains a small part of cognitive function. Shared genetic etiology exists, however, greater genetic heterogeneity exists within disease classifications after accounting for the baseline diagnosis status. These analyses are useful in further investigation of functional mechanisms for AD evolution.
更多查看译文
关键词
functional linear modeling framework,genetic,imaging
AI 理解论文
溯源树
样例
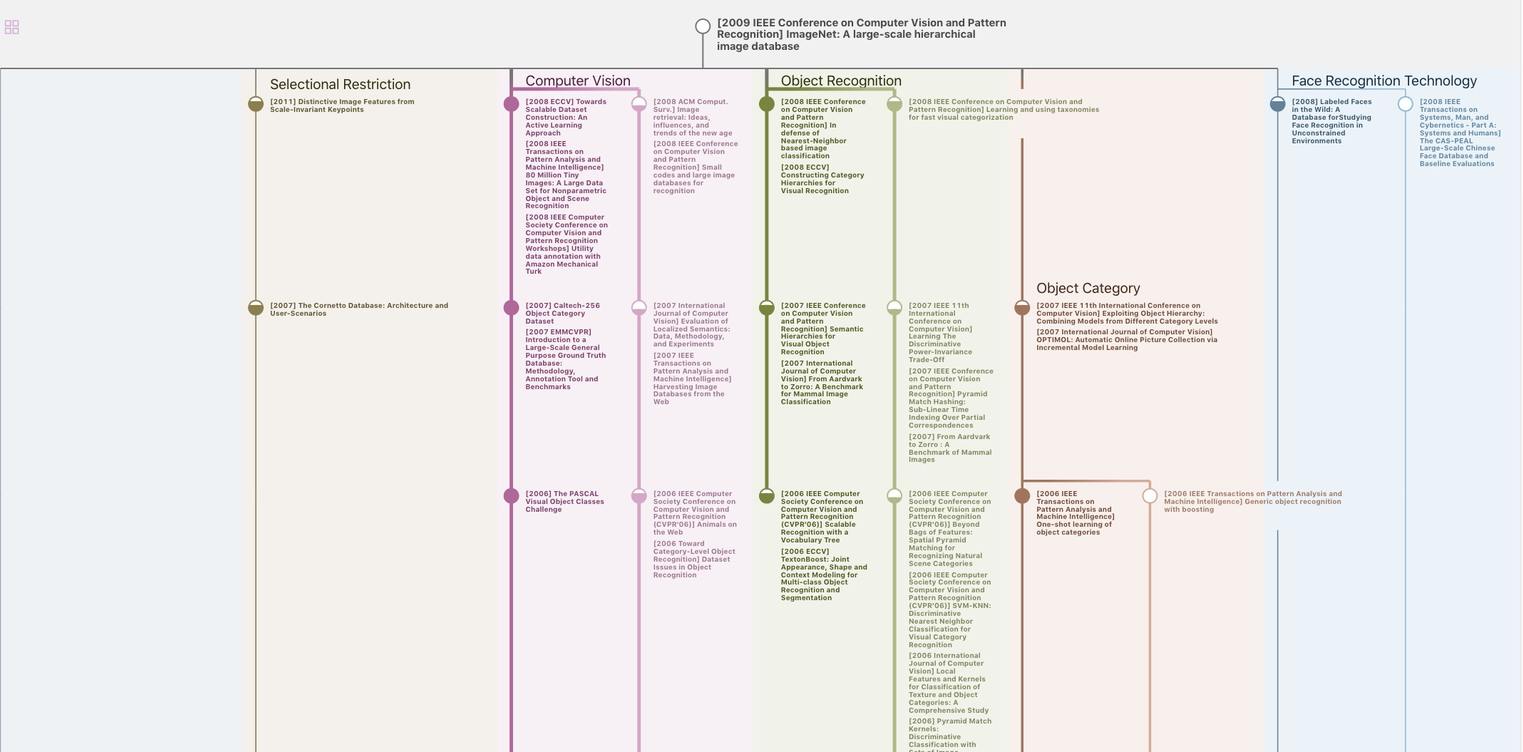
生成溯源树,研究论文发展脉络
Chat Paper
正在生成论文摘要