Understanding Influence Functions and Datamodels via Harmonic Analysis
ICLR 2023(2022)
摘要
Influence functions estimate effect of individual data points on predictions of the model on test data and were adapted to deep learning in Koh and Liang [2017]. They have been used for detecting data poisoning, detecting helpful and harmful examples, influence of groups of datapoints, etc. Recently, Ilyas et al. [2022] introduced a linear regression method they termed datamodels to predict the effect of training points on outputs on test data. The current paper seeks to provide a better theoretical understanding of such interesting empirical phenomena. The primary tool is harmonic analysis and the idea of noise stability. Contributions include: (a) Exact characterization of the learnt datamodel in terms of Fourier coefficients. (b) An efficient method to estimate the residual error and quality of the optimum linear datamodel without having to train the datamodel. (c) New insights into when influences of groups of datapoints may or may not add up linearly.
更多查看译文
关键词
theory,datamodels,influence functions,fourier analysis,harmonic analysis
AI 理解论文
溯源树
样例
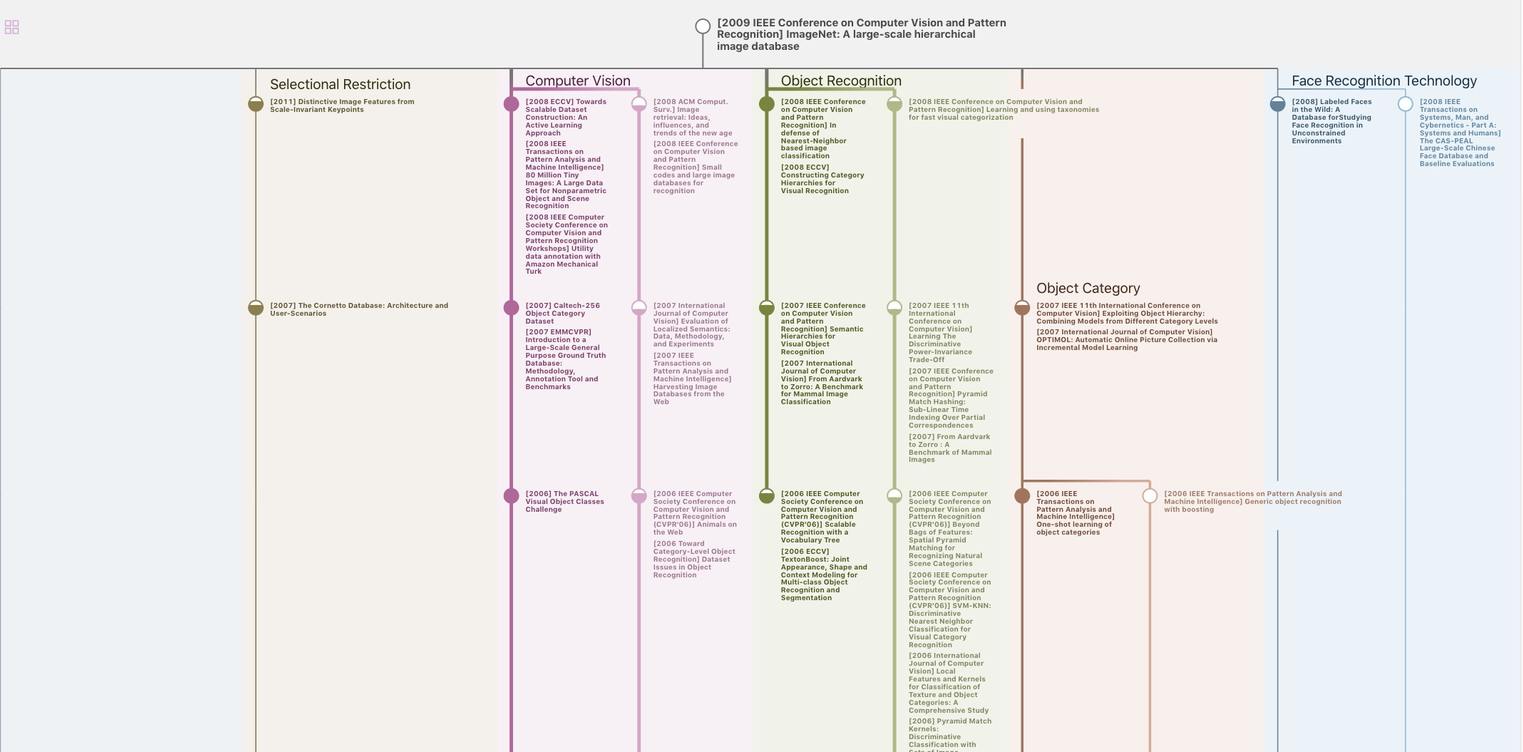
生成溯源树,研究论文发展脉络
Chat Paper
正在生成论文摘要