Information-Theoretic Analysis of Unsupervised Domain Adaptation
ICLR 2023(2022)
摘要
This paper uses information-theoretic tools to analyze the generalization error in unsupervised domain adaptation (UDA). We present novel upper bounds for two notions of generalization errors. The first notion measures the gap between the population risk in the target domain and that in the source domain, and the second measures the gap between the population risk in the target domain and the empirical risk in the source domain. While our bounds for the first kind of error are in line with the traditional analysis and give similar insights, our bounds on the second kind of error are algorithm-dependent, which also provide insights into algorithm designs. Specifically, we present two simple techniques for improving generalization in UDA and validate them experimentally.
更多查看译文
关键词
unsupervised domain adaptation,generalization,information theory,regularization
AI 理解论文
溯源树
样例
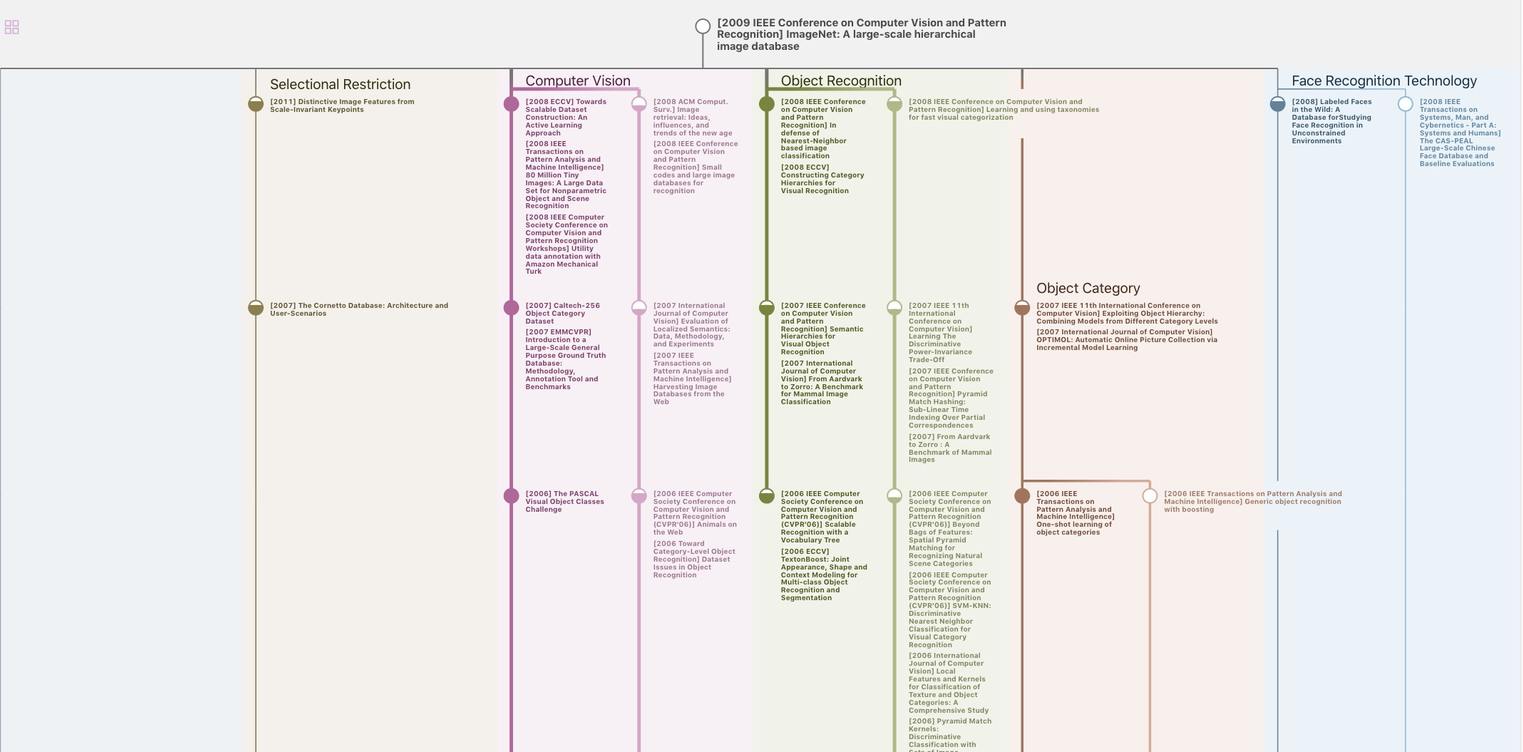
生成溯源树,研究论文发展脉络
Chat Paper
正在生成论文摘要