Evolution is Still Good: Theoretical Analysis of Evolutionary Algorithms on General Cover Problems
arxiv(2022)
摘要
Theoretical studies on evolutionary algorithms have developed vigorously in recent years. Many such algorithms have theoretical guarantees in both running time and approximation ratio. Some approximation mechanism seems to be inherently embedded in many evolutionary algorithms. In this paper, we identify such a relation by proposing a unified analysis framework for a generalized simple multi-objective evolutionary algorithm (GSEMO), and apply it on a minimum weight general cover problem. For a wide range of problems (including the the minimum submodular cover problem in which the submodular function is real-valued, and the minimum connected dominating set problem for which the potential function is non-submodular), GSEMO yields asymptotically tight approximation ratios in expected polynomial time.
更多查看译文
关键词
evolutionary algorithms
AI 理解论文
溯源树
样例
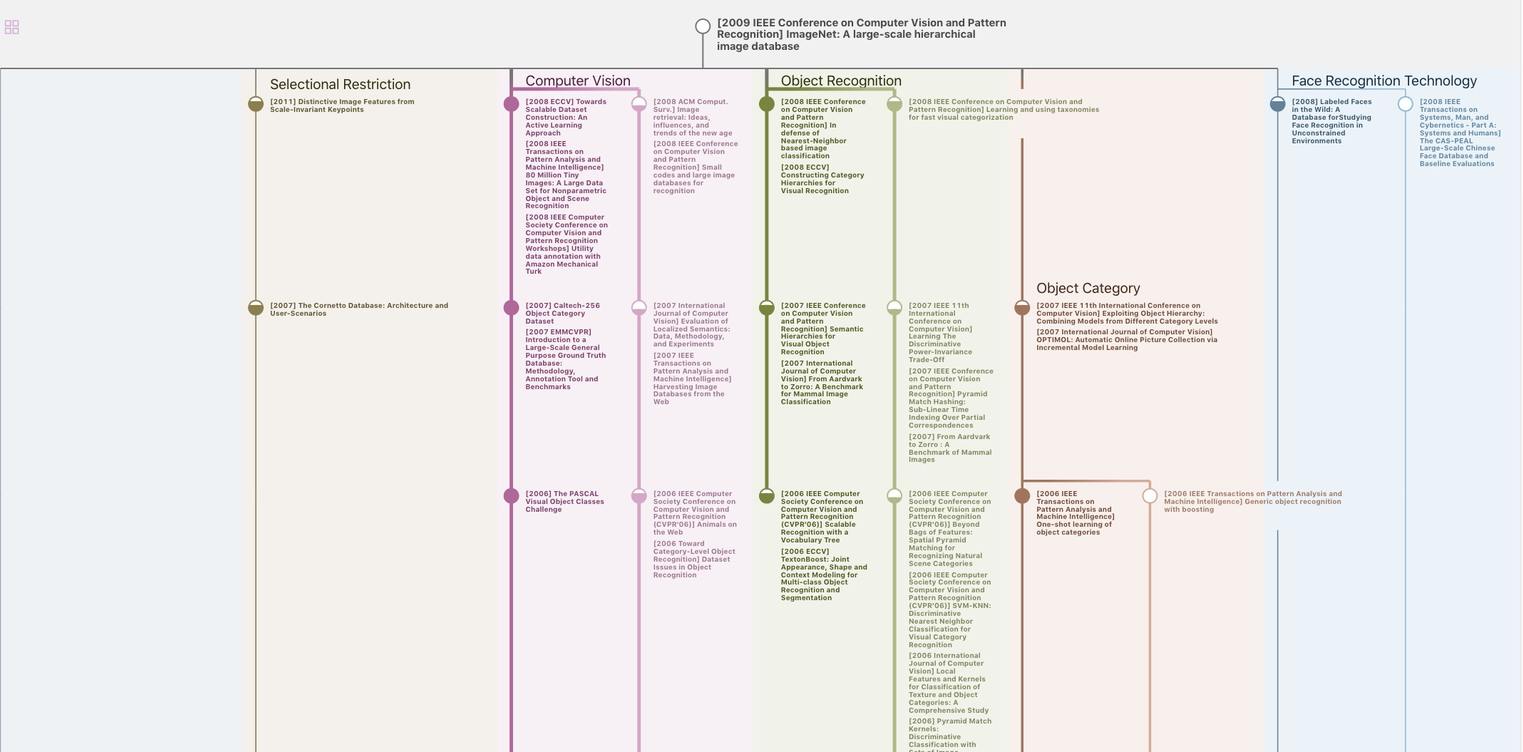
生成溯源树,研究论文发展脉络
Chat Paper
正在生成论文摘要