Efficiently Learning Small Policies for Locomotion and Manipulation
2023 IEEE INTERNATIONAL CONFERENCE ON ROBOTICS AND AUTOMATION, ICRA(2023)
摘要
Neural control of memory-constrained, agile robots requires small, yet highly performant models. We leverage graph hyper networks to learn graph hyper policies trained with off-policy reinforcement learning resulting in networks that are two orders of magnitude smaller than commonly used networks yet encode policies comparable to those encoded by much larger networks trained on the same task. We show that our method can be appended to any off-policy reinforcement learning algorithm, without any change in hyperparameters, by showing results across locomotion and manipulation tasks. Further, we obtain an array of working policies, with differing numbers of parameters, allowing us to pick an optimal network for the memory constraints of a system. Training multiple policies with our method is as sample efficient as training a single policy. Finally, we provide a method to select the best architecture, given a constraint on the number of parameters. Project website: https://sites.google.com/usc.edu/graphhyperpolicy
更多查看译文
关键词
locomotion,small policies,learning
AI 理解论文
溯源树
样例
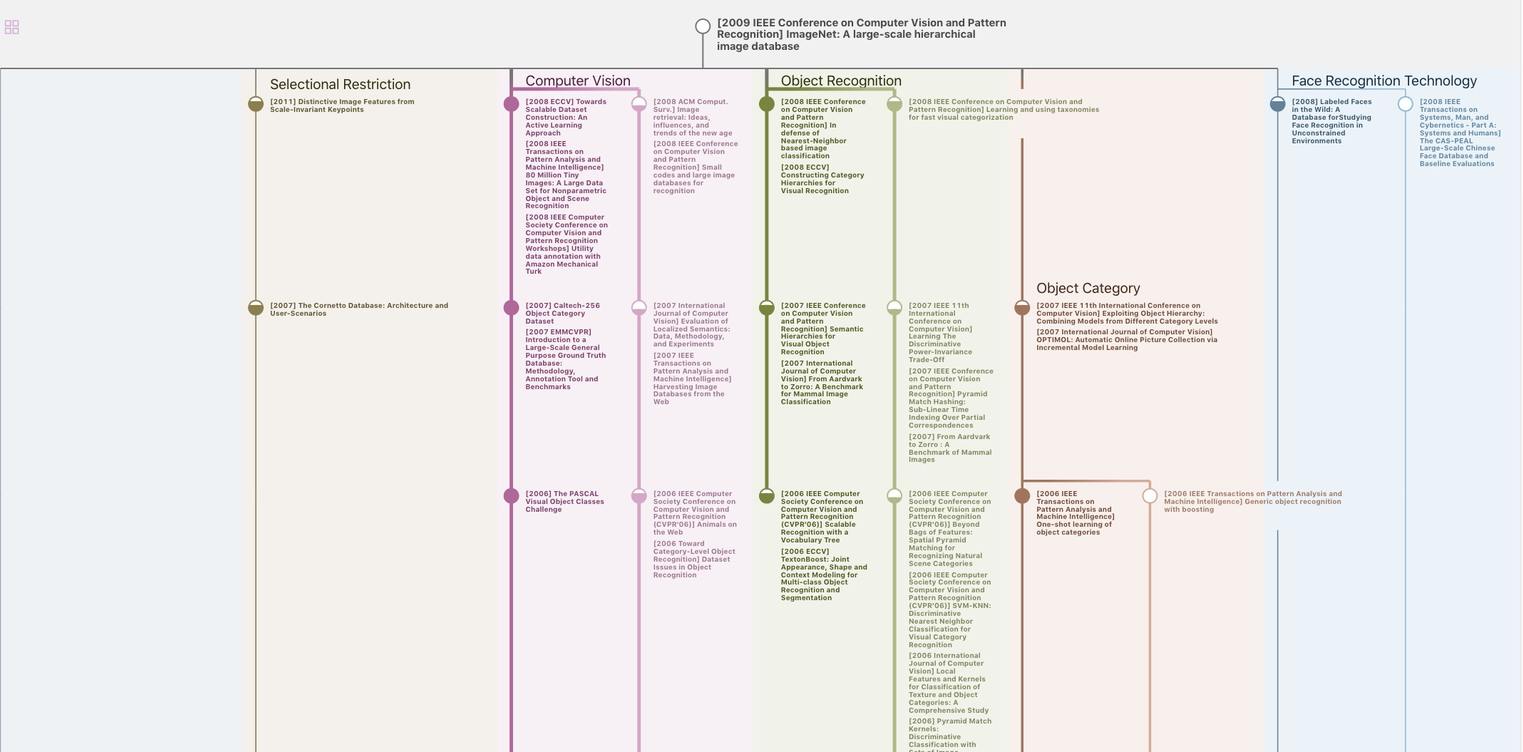
生成溯源树,研究论文发展脉络
Chat Paper
正在生成论文摘要