Multi-Task Option Learning and Discovery for Stochastic Path Planning
ICLR 2023(2022)
摘要
This paper addresses the problem of reliably and efficiently solving broad classes of long-horizon stochastic path planning problems. Starting with a vanilla RL formulation with a stochastic dynamics simulator and an occupancy matrix of the environment, our approach computes useful options with policies as well as high-level paths that compose the discovered options. Our main contributions are (1) data-driven methods for creating abstract states that serve as endpoints for helpful options, (2) methods for computing option policies using auto-generated option guides in the form of dense pseudo-reward functions, and (3) an overarching algorithm for composing the computed options. We show that this approach yields strong guarantees of executability and solvability: under fairly general conditions, the computed option guides lead to composable option policies and consequently ensure downward refinability. Empirical evaluation on a range of robots, environments, and tasks shows that this approach effectively transfers knowledge across related tasks and that it outperforms existing approaches by a significant margin.
更多查看译文
关键词
Option discovery,learning abstractions,planning and learning,reinforcement learning,RL for robotics,hierarchical methods,stochastic path planning
AI 理解论文
溯源树
样例
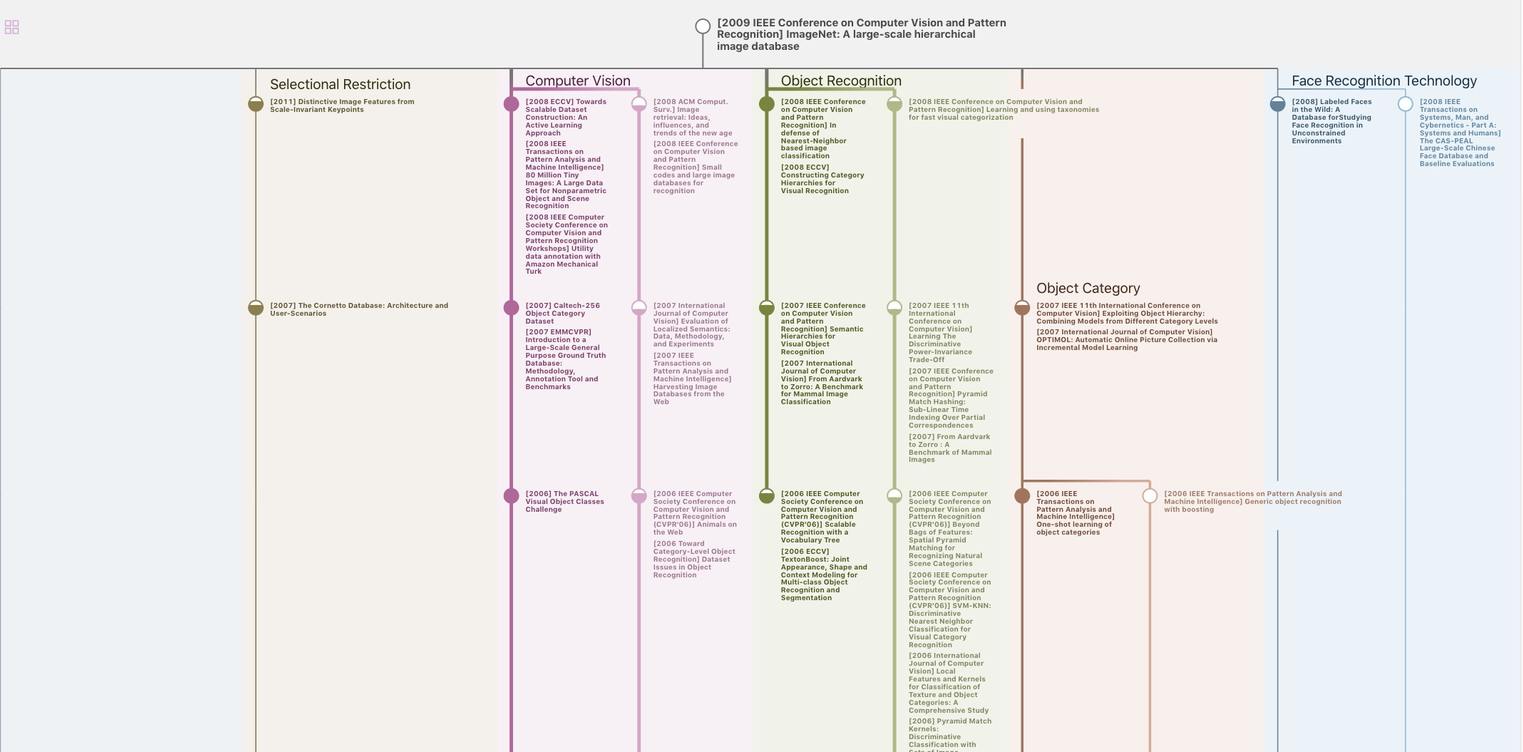
生成溯源树,研究论文发展脉络
Chat Paper
正在生成论文摘要