Early detection of Sepsis in critical patients using Random Forest Classifier
2020 IEEE Region 10 Symposium (TENSYMP)(2020)
摘要
Time plays a pivotal rule in successful control of sepsis-associated infections and mortality in ICU patients. Accurate and confident prediction of sepsis ahead of time is a challenging task owing to the complex nature of the underlying pathophysiological mechanisms. Missing data in the laboratory values which have been reported by medical findings to be important for forecasting sepsis makes the problem worse. To address the problem of missing data, last observation carried forward (LOCF) method has been adopted in this paper. This has been proved to be a simple yet effective idea. The remaining missing features were substituted with zero, which is one type of constant imputation. Out of the 40 available features, 19 were selected by performing the analysis of variance (ANOVA) test. The class imbalance problem was addressed via cost-sensitive learning which gave more weight to the minority class data points based on their proportion. Finally, a random forest classifier was trained upon the data. The model obtained a utility score of approximately 57% and 22% respectively on the two datasets which were taken from Physionet/Computing in Cardiology (CinC) challenge, 2019.
更多查看译文
关键词
Random forest classifier,constant imputation,last observation carried forward(LOCF)
AI 理解论文
溯源树
样例
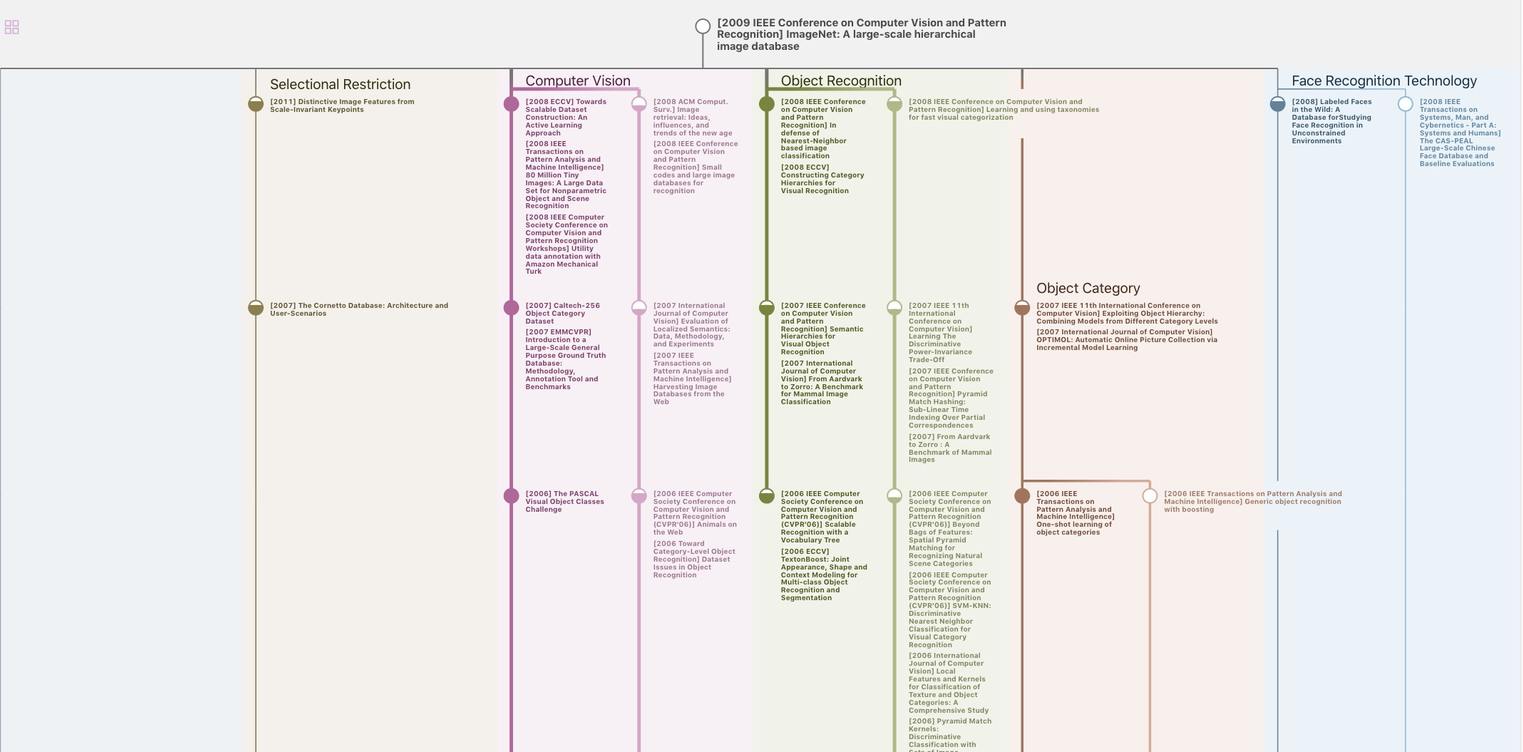
生成溯源树,研究论文发展脉络
Chat Paper
正在生成论文摘要