GROUP: Global RObUst and Privacy Preserved Model for Diabetes Prediction
2022 IEEE Region 10 Symposium (TENSYMP)(2022)
摘要
The tremendous advancements in biomedical health sciences have resulted in a substantial amount of data generation, such as high-throughput genomics information and clinical data. These data are widely used by researchers to build predictive models to aid in the early diagnosis of a disease or disorder. Employing Artificial Intelligence algorithms to extract knowledge from enormous amounts of available health-related data is a technique of prime importance to make decisions. We have reviewed the work of many researchers and found the necessity of a model that could successfully tackle the problems of user data privacy and robustness. In this paper, we present a model that predicts whether a patient is likely or not to be diagnosed with the most common disorder, Diabetes in the near future on the basis of his/her health records. The suggested paradigm employs the notions of Federated learning to preserve privacy and uses Neural Network to make predictions based on the available training set. To demonstrate the efficacy of the model we conducted evaluation tests. Our model approaches an accuracy of 78% and 81.36% for Independent and Identical Distribution (IID) and non-Independent and Identical Distribution (non IID) datasets, respectively.
更多查看译文
关键词
Federated Learning,Diabetes Prediction,User Privacy,Federated Averaging
AI 理解论文
溯源树
样例
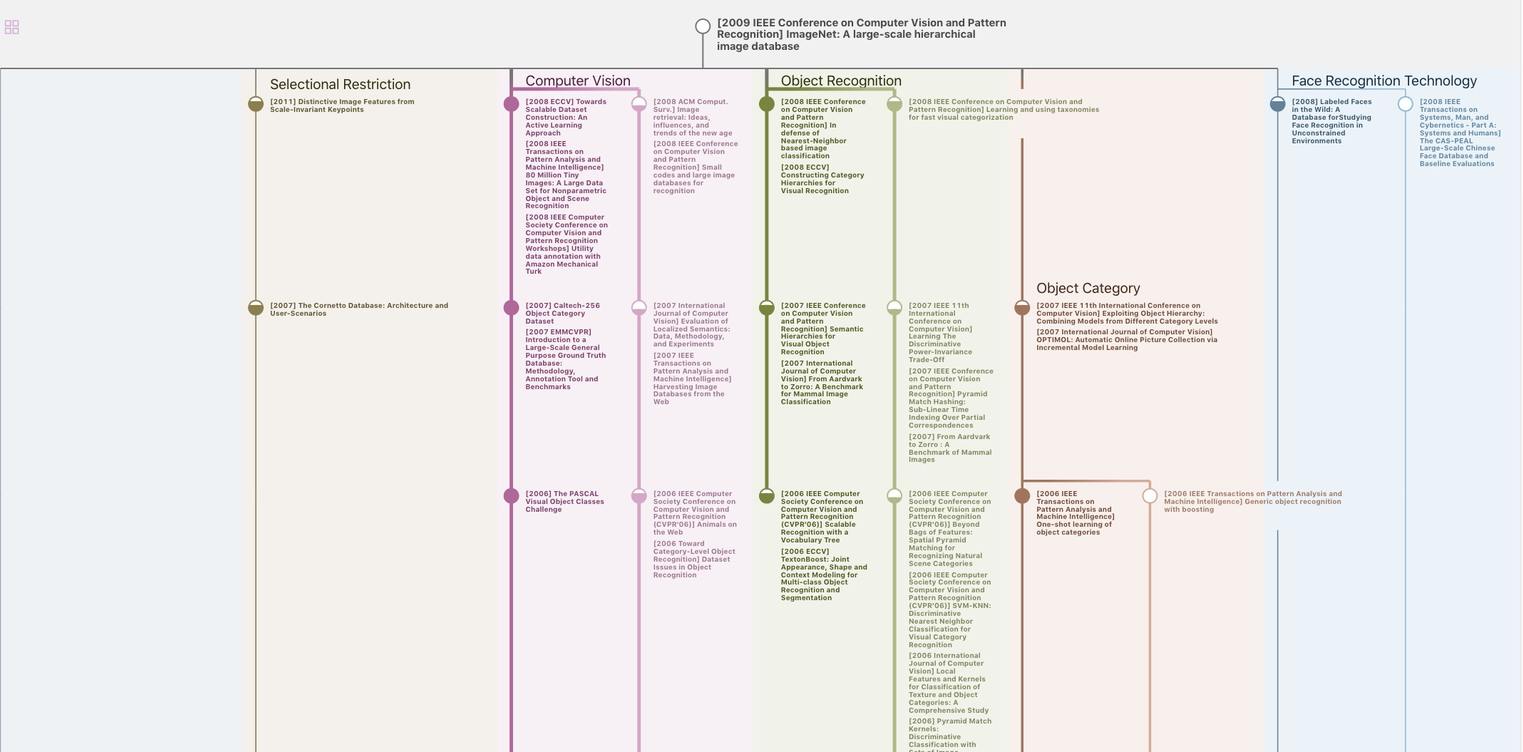
生成溯源树,研究论文发展脉络
Chat Paper
正在生成论文摘要