Generalized Tonic-Clonic Seizure Detection through the Use of Physiological Signals
2022 3rd International Conference on Pattern Recognition and Machine Learning (PRML)(2022)
摘要
Generalized tonic-clonic seizures increase the risk of sudden unexpected death in epilepsy, especially for patients without caregivers around them. There is a need for wearable devices with the ability to detect on-going seizures in an in-home care setting. Video-EEG is the gold standard of seizure detection, but lack of practicability for long-term daily monitoring. Recent years, various studies on wristband that accepted well by patients have achieved high sensitivity but also high false alarm rate (FAR) on seizure detection. To decrease false alarms, we proposed a seizure detection method on multimodal signals related to GTCS, including six-axis accelerometer (ACM), surface electromyography (sEMG) and electrodermal activity (EDA). The method consisted of two classifiers, one for identifying resting state and moving state and the other for identifying seizure state and non-seizure state from segments in moving state, and post-processing consisted of a mean filter and threshold crossing for restraining outliers. In this work, 4 patients were enrolled, and wristband recordings reached 3786 hour with 68 GTCS in total. We achieved a mean sensitivity of 81.69% and FDR of 0.64/24h, and the result was better than using just one signal, ACM, sEMG or EDA. Besides, the FAR of multimodal signals was lower than that of any signal at the same level of sensitivity, which meant that it’s a feasible solution to combine the multimodal signals for purpose of less false alarms on the basis of high sensitivity.
更多查看译文
关键词
seizure detection,generalized tonic-clonic seizure,multimodal,wristband
AI 理解论文
溯源树
样例
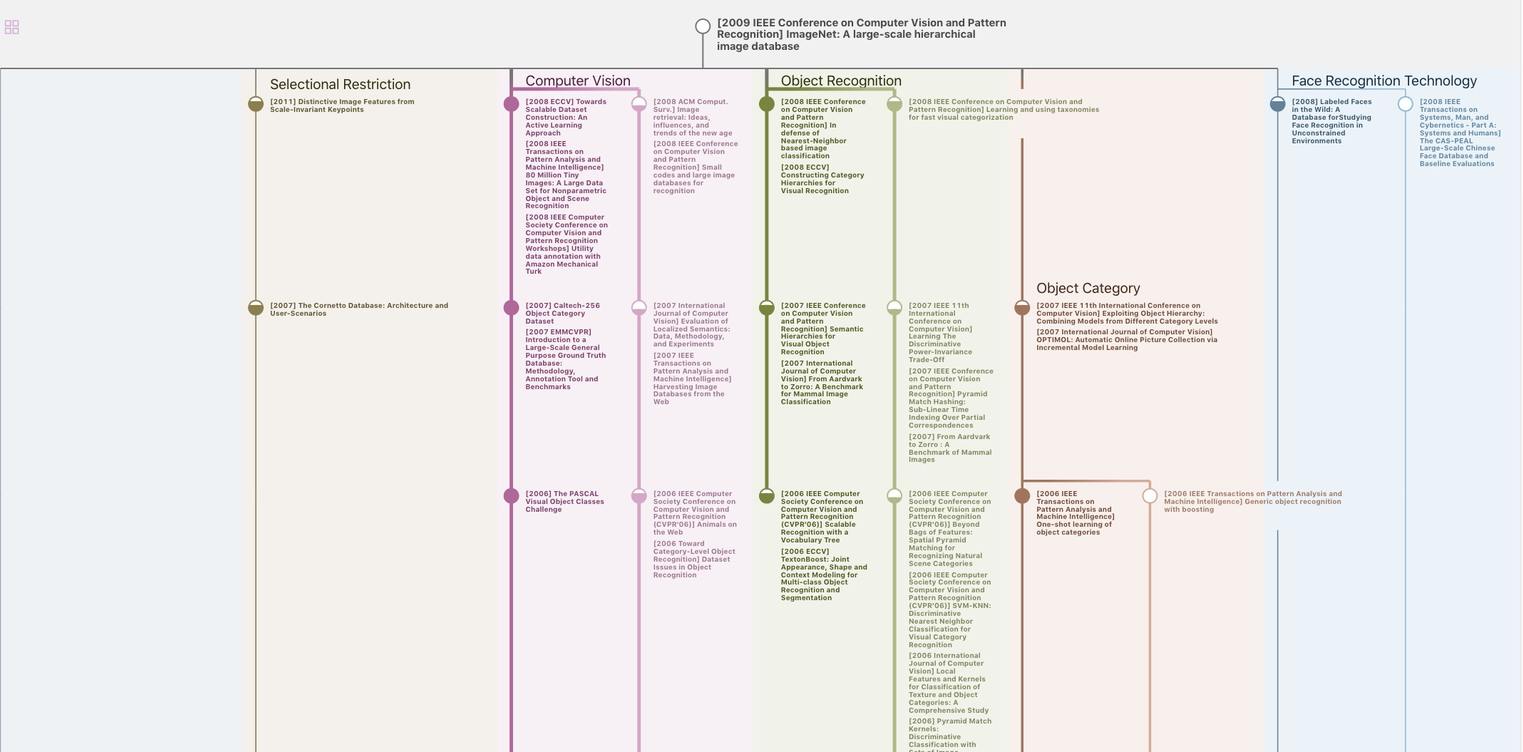
生成溯源树,研究论文发展脉络
Chat Paper
正在生成论文摘要