Deep Learning vs Keypoint: Which Feature Set Is Better for Shell Recognition?
2022 12th International Conference on Pattern Recognition Systems (ICPRS)(2022)
摘要
The shells of aquatic molluscs are ubiquitous in many coastal areas. While shells have been valued for decorative, cultural and economic reasons for millennia, they also have significant utility as ecological indicators of marine and freshwater biodiversity, pollution and water quality. With tens of thousands of species, shells are difficult to identify manually, necessitating automated approaches. Machine learning and computer vision can be employed to solve this issue. Unfortunately, very few studies have investigating classifying shells using these technologies. This paper presents an end-to-end shell species detection pipeline comprising feature extraction and recognition components. We exhaustively investigate various feature extraction methods, including those using domain knowledge, keypoints, and pretrained deep neural networks. Three classifiers are used to validate the discriminative capabilities of all generated features: support vector machine, random forest, and feed-forward neural network. Using a recently proposed shell dataset, we show that our best model - SVM trained on pretrained VGG16 features achieved 96% accuracy and macro-average F1, outperforming existing benchmarks by more than 13 % in both metrics.
更多查看译文
关键词
machine learning,computer vision,shell species recognition,deep learning features,keypoint features,transfer learning
AI 理解论文
溯源树
样例
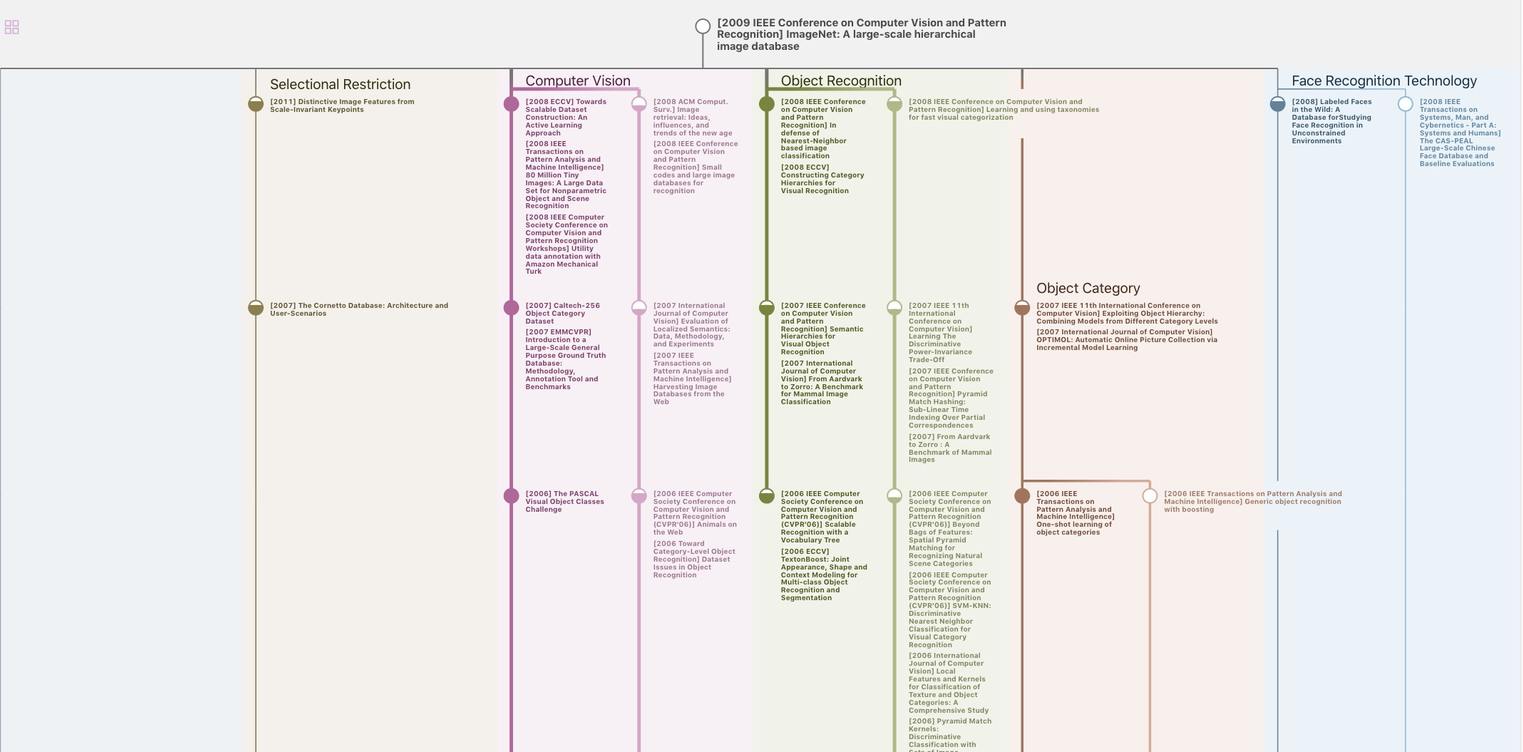
生成溯源树,研究论文发展脉络
Chat Paper
正在生成论文摘要