Stabilize the scale by geometry consistency in self-supervised monocular depth estimation
2022 IEEE Asia-Pacific Conference on Image Processing, Electronics and Computers (IPEC)(2022)
摘要
Limited by the difficulty to gather per-pixel ground truth depth data, self-supervised depth estimation suggests an alternative approach to take advantage of large-scale dataset of autonomous driving scenes. Compared to binocular or multi-view stereo methods, depth estimation on monocular videos appears easy to implement. However, the scale-ambiguity of monocular videos prevents the metric-scale outputs of depth value and causes scale-shift that limits further application such as SLAM. In this paper we attempt to stabilize the scale of depth map by geometry consistency. Experiments on KITTI dataset illustrate that our method gains progress both on the depth and the visual odometry tasks.
更多查看译文
关键词
monocular depth estimation,self-supervised,autonomous driving
AI 理解论文
溯源树
样例
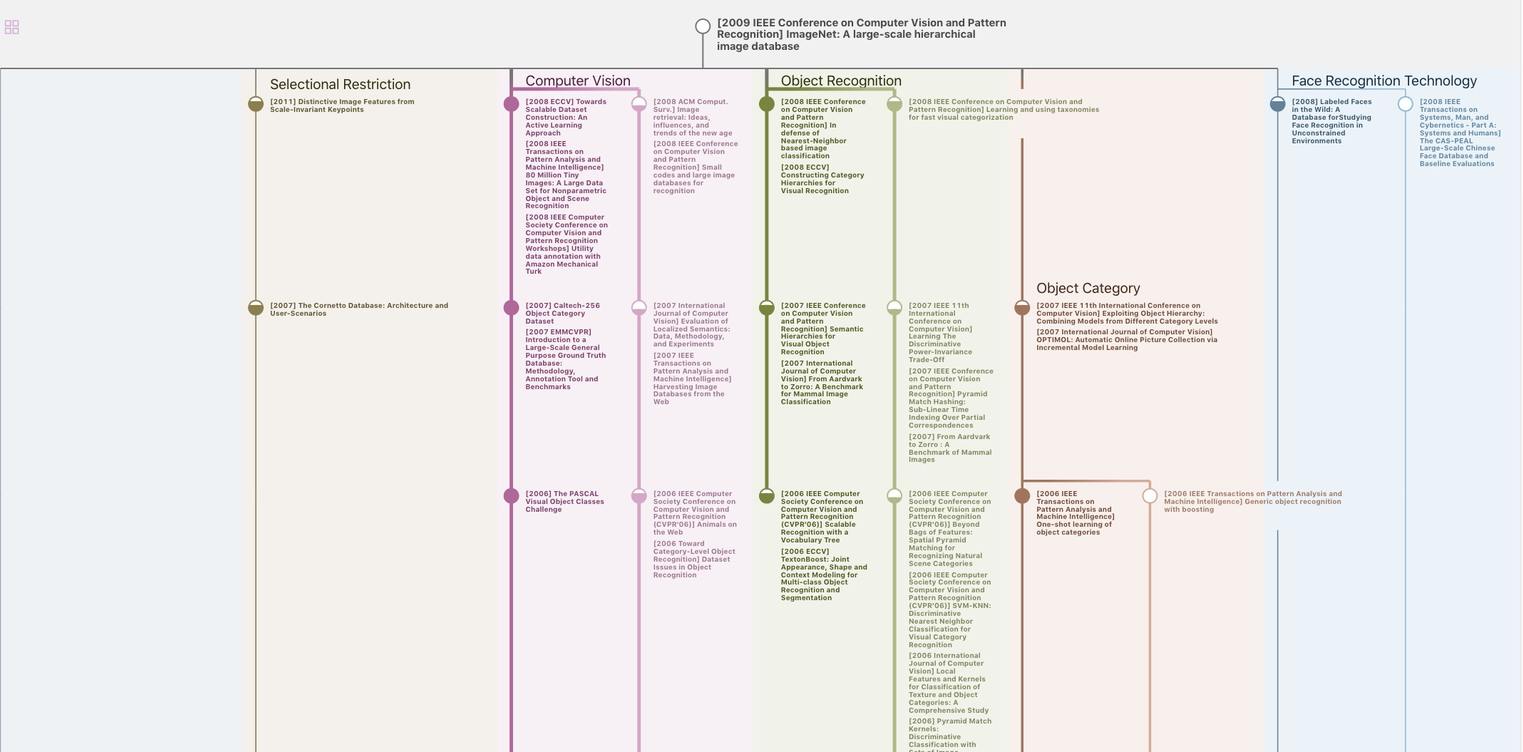
生成溯源树,研究论文发展脉络
Chat Paper
正在生成论文摘要