Scheduling for Urban Air Mobility using Safe Learning.
Workshop on Formal Methods for Autonomous Systems (FMAS)(2022)
摘要
This work considers the scheduling problem for Urban Air Mobility (UAM) vehicles travelling between origin-destination pairs with both hard and soft trip deadlines. Each route is described by a discrete probability distribution over trip completion times (or delay) and over inter-arrival times of requests (or demand) for the route along with a fixed hard or soft deadline. Soft deadlines carry a cost that is incurred when the deadline is missed. An online, safe scheduler is developed that ensures that hard deadlines are never missed, and that average cost of missing soft deadlines is minimized. The system is modelled as a Markov Decision Process (MDP) and safe model-based learning is used to find the probabilistic distributions over route delays and demand. Monte Carlo Tree Search (MCTS) Earliest Deadline First (EDF) is used to safely explore the learned models in an online fashion and develop a near-optimal non-preemptive scheduling policy. These results are compared with Value Iteration (VI) and MCTS (Random) scheduling solutions.
更多查看译文
关键词
urban air mobility,scheduling
AI 理解论文
溯源树
样例
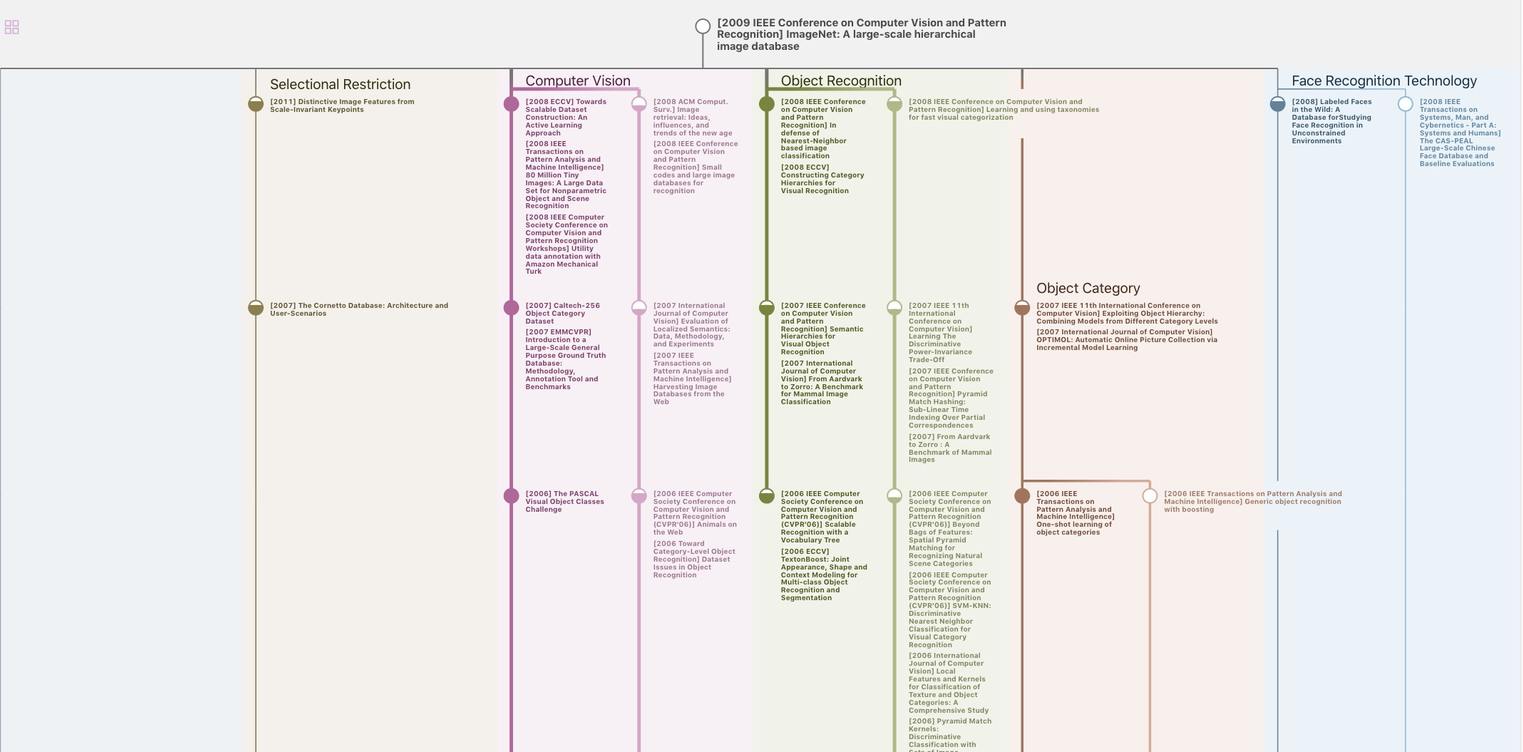
生成溯源树,研究论文发展脉络
Chat Paper
正在生成论文摘要