A Learnable Optimization and Regularization Approach to Massive MIMO CSI Feedback
IEEE TRANSACTIONS ON WIRELESS COMMUNICATIONS(2024)
摘要
Channel state information (CSI) plays a critical role in achieving the potential benefits of massive multiple input multiple output (MIMO) systems. In frequency division duplex (FDD) massive MIMO systems, the base station (BS) relies on sustained and accurate CSI feedback from users. However, due to the large number of antennas and users being served in massive MIMO systems, feedback overhead can become a bottleneck. In this paper, we propose a model-driven deep learning method for CSI feedback, called learnable optimization and regularization algorithm (LORA). Instead of using l(1)-norm as the regularization term, LORA introduces a learnable regularization module that adapts to characteristics of CSI automatically. The conventional Iterative Shrinkage-Thresholding Algorithm (ISTA) is unfolded into a neural network, which can learn both the optimization process and the regularization term by end-to-end training. We show that LORA improves the CSI feedback accuracy and speed. Besides, a novel learnable quantization method and the corresponding training scheme are proposed, and it is shown that LORA can operate successfully at different bit rates, providing flexibility in terms of the CSI feedback overhead. Various realistic scenarios are considered to demonstrate the effectiveness and robustness of LORA through numerical simulations.
更多查看译文
关键词
Massive MIMO,CSI feedback,model-driven,deep learning,regularization learning
AI 理解论文
溯源树
样例
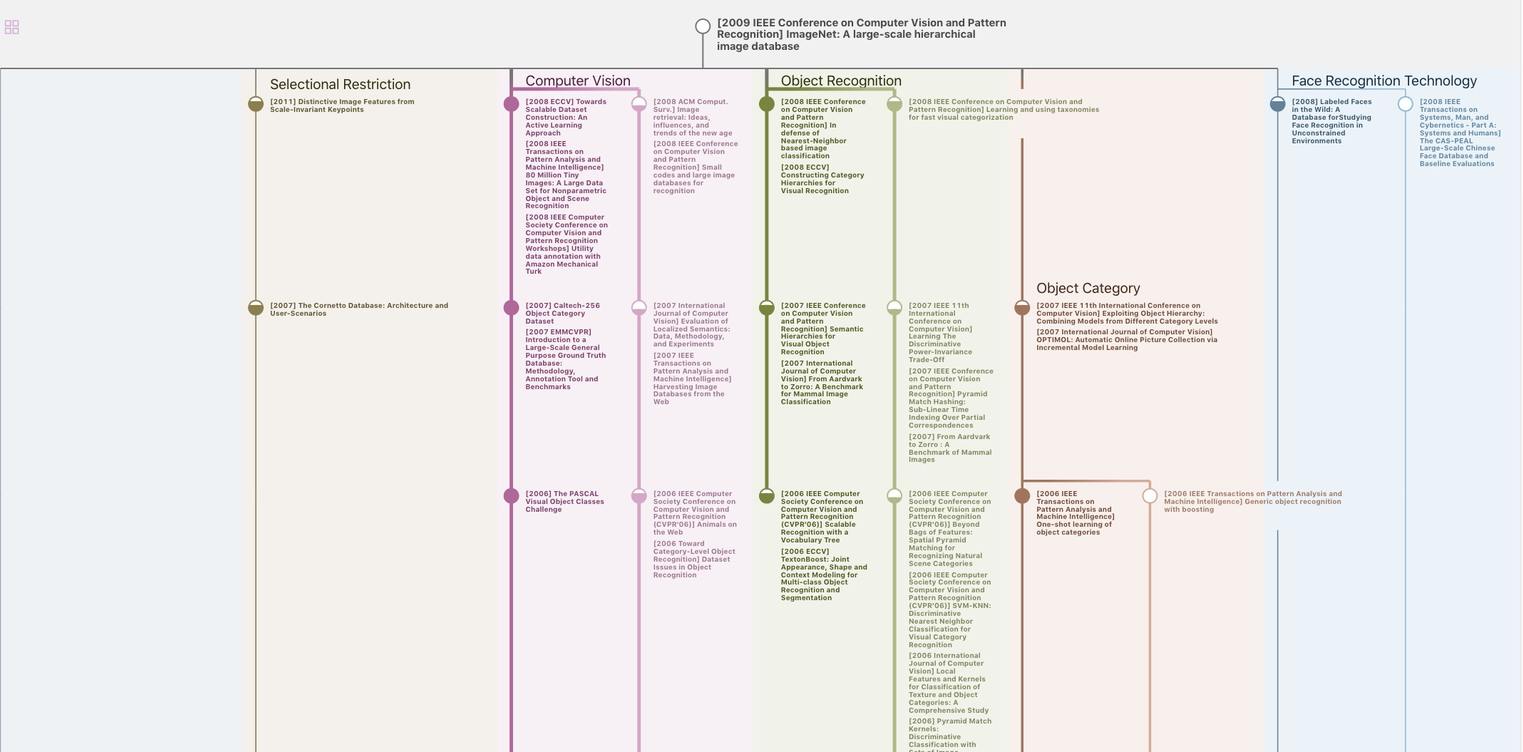
生成溯源树,研究论文发展脉络
Chat Paper
正在生成论文摘要