Many-Body Approximation for Tensors
ICLR 2023(2022)
摘要
We propose a nonnegative tensor decomposition with focusing on the relationship between the modes of tensors. Traditional decomposition methods assume low-rankness in the representation, resulting in difficulties in global optimization and target rank selection. To address these problems, we present an alternative way to decompose tensors, a many-body approximation for tensors, based on an information geometric formulation. A tensor is treated via an energy-based model, where the tensor and its mode correspond to a probability distribution and a random variable, respectively, and many-body approximation is performed on it by taking the interaction between variables into account. Our model can be globally optimized in polynomial time in terms of the KL divergence minimization, which is empirically faster than low-rank approximations keeping comparable reconstruction error. Furthermore, we visualize interactions between modes as tensor networks and reveal a nontrivial relationship between many-body approximation and low-rank approximation.
更多查看译文
关键词
Tensor decomposition,Energy based model,Tensor networks
AI 理解论文
溯源树
样例
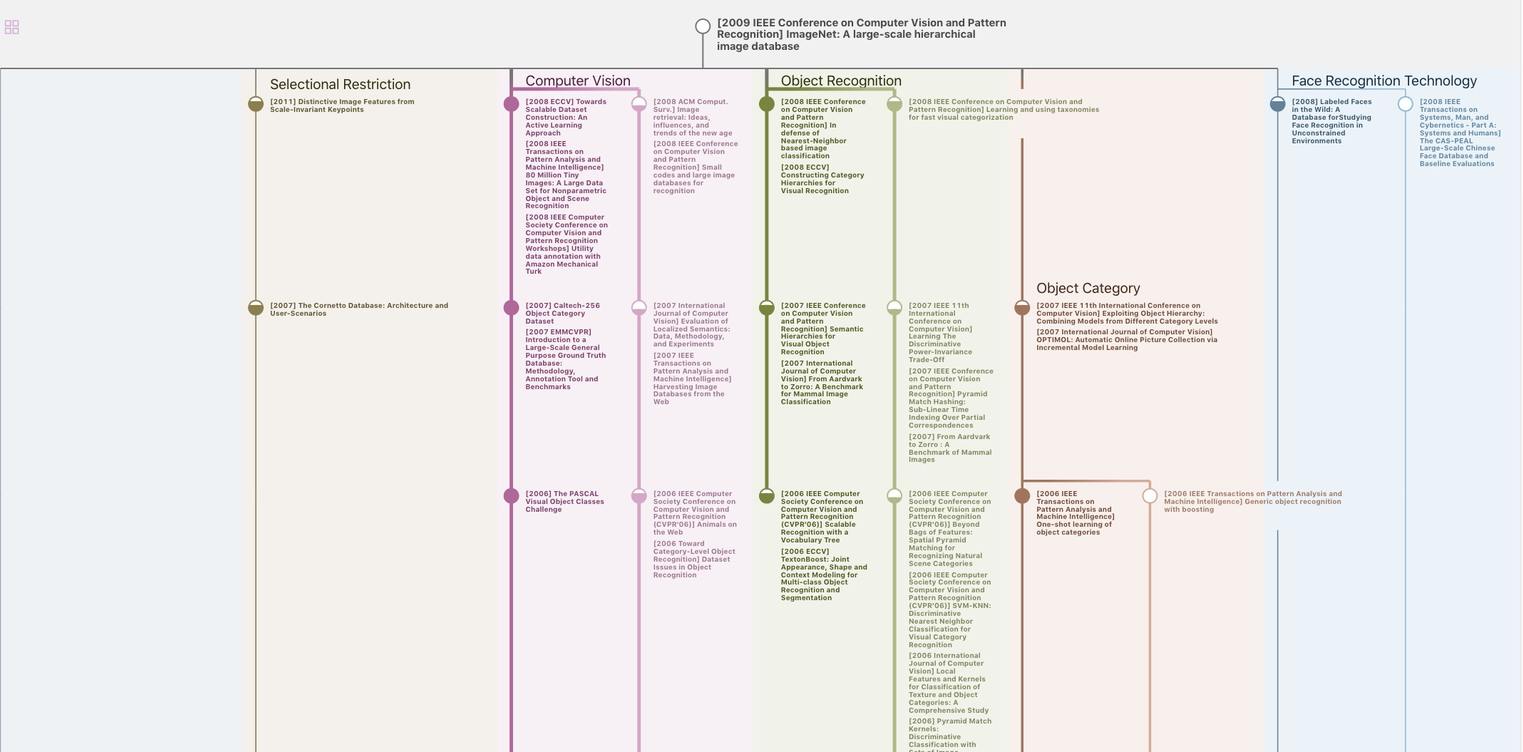
生成溯源树,研究论文发展脉络
Chat Paper
正在生成论文摘要