Did You Get What You Paid For? Rethinking Annotation Cost of Deep Learning Based Computer Aided Detection in Chest Radiographs
MEDICAL IMAGE COMPUTING AND COMPUTER ASSISTED INTERVENTION, MICCAI 2022, PT III(2022)
摘要
As deep networks require large amounts of accurately labeled training data, a strategy to collect sufficiently large and accurate annotations is as important as innovations in recognition methods. This is especially true for building Computer Aided Detection (CAD) systems for chest X-rays where domain expertise of radiologists is required to annotate the presence and location of abnormalities on X-ray images. However, there lacks concrete evidence that provides guidance on how much resource to allocate for data annotation such that the resulting CAD system reaches desired performance. Without this knowledge, practitioners often fall back to the strategy of collecting as much detail as possible on as much data as possible which is cost inefficient. In this work, we investigate how the cost of data annotation ultimately impacts the CAD model performance on classification and segmentation of chest abnormalities in frontal-view X-ray images. We define the cost of annotation with respect to the following three dimensions: quantity, quality and granularity of labels. Throughout this study, we isolate the impact of each dimension on the resulting CAD model performance on detecting 10 chest abnormalities in X-rays. On a large scale training data with over 120K X-ray images with gold-standard annotations, we find that cost-efficient annotations provide great value when collected in large amounts and lead to competitive performance when compared to models trained with only gold-standard annotations. We also find that combining large amounts of cost efficient annotations with only small amounts of expensive labels leads to competitive CAD models at a much lower cost.
更多查看译文
关键词
Annotation cost, Classification, Segmentation, Chest X-ray, Chest abnormality
AI 理解论文
溯源树
样例
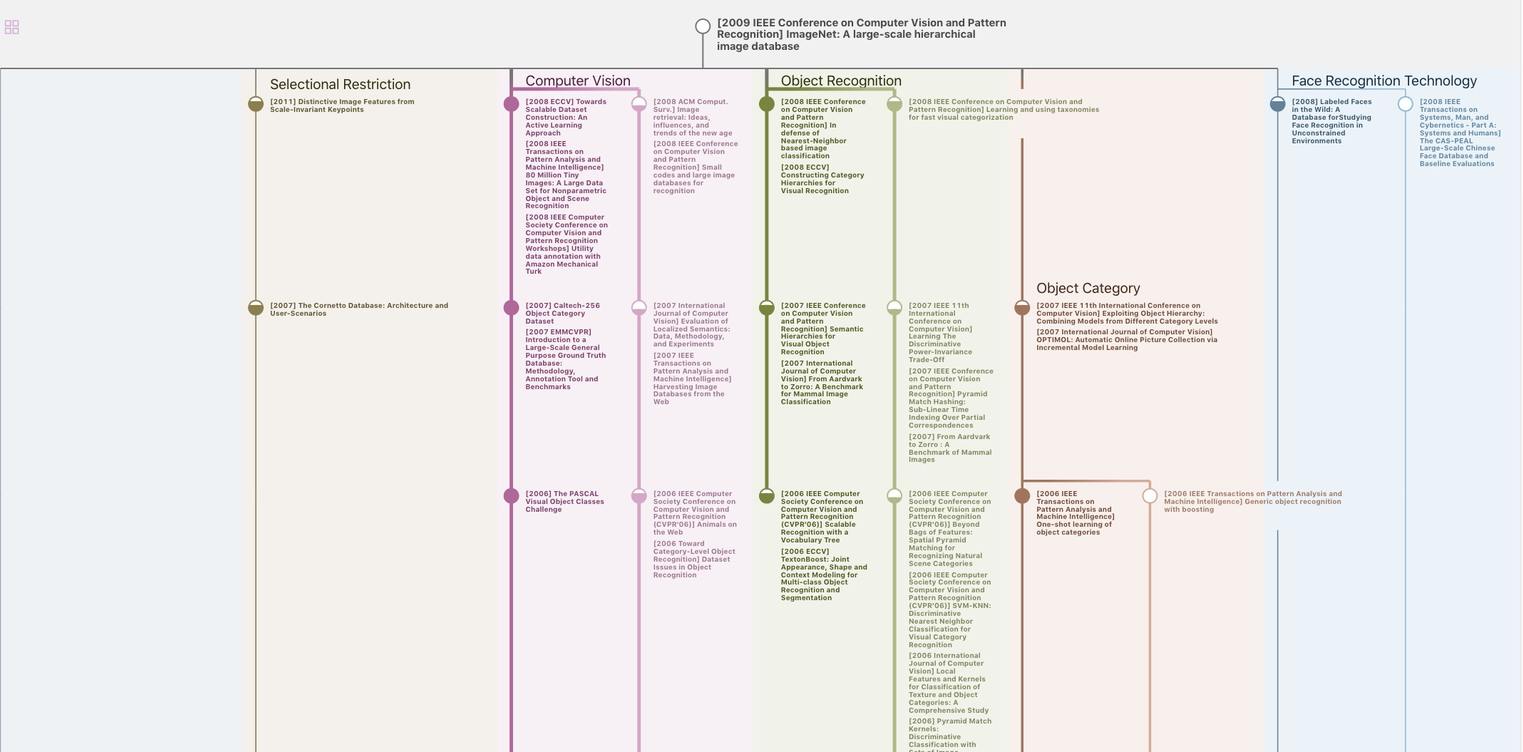
生成溯源树,研究论文发展脉络
Chat Paper
正在生成论文摘要