Enforcing Hard Constraints with Soft Barriers: Safe Reinforcement Learning in Unknown Stochastic Environments
arxiv(2022)
摘要
It is quite challenging to ensure the safety of reinforcement learning (RL) agents in an unknown and stochastic environment under hard constraints that require the system state not to reach certain specified unsafe regions. Many popular safe RL methods such as those based on the Constrained Markov Decision Process (CMDP) paradigm formulate safety violations in a cost function and try to constrain the expectation of cumulative cost under a threshold. However, it is often difficult to effectively capture and enforce hard reachability-based safety constraints indirectly with such constraints on safety violation costs. In this work, we leverage the notion of barrier function to explicitly encode the hard safety constraints, and given that the environment is unknown, relax them to our design of \emph{generative-model-based soft barrier functions}. Based on such soft barriers, we propose a safe RL approach that can jointly learn the environment and optimize the control policy, while effectively avoiding unsafe regions with safety probability optimization. Experiments on a set of examples demonstrate that our approach can effectively enforce hard safety constraints and significantly outperform CMDP-based baseline methods in system safe rate measured via simulations.
更多查看译文
关键词
soft barriers,safe reinforcement
AI 理解论文
溯源树
样例
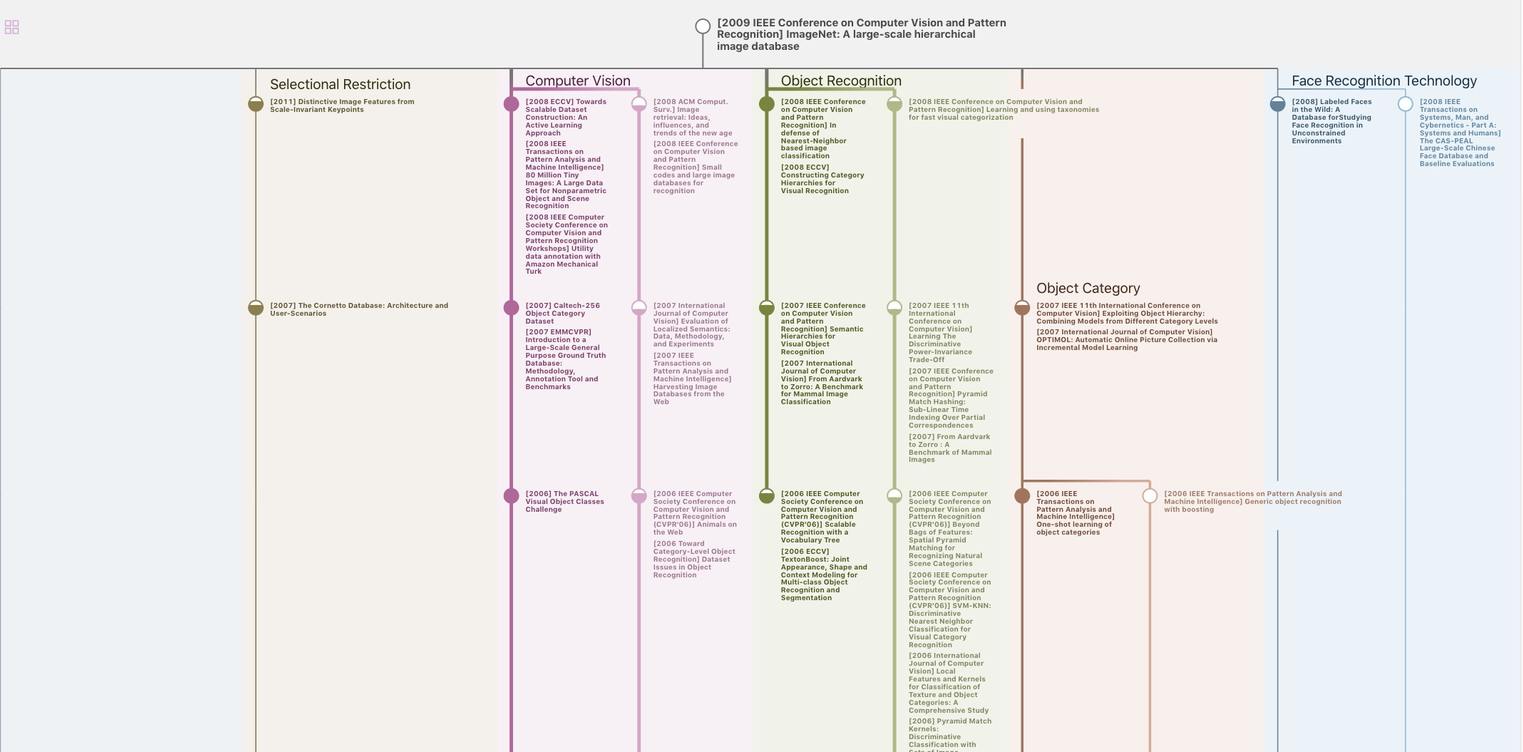
生成溯源树,研究论文发展脉络
Chat Paper
正在生成论文摘要