L-SRR: Local Differential Privacy for Location-Based Services with Staircase Randomized Response.
Conference on Computer and Communications Security (CCS)(2022)
摘要
Location-based services (LBS) have been significantly developed and widely deployed in mobile devices. It is also well-known that LBS applications may result in severe privacy concerns by collecting sensitive locations. A strong privacy model ''local differential privacy'' (LDP) has been recently deployed in many different applications (e.g., Google RAPPOR, iOS, and Microsoft Telemetry) but not effective for LBS applications due to the low utility of existing LDP mechanisms. To address such deficiency, we propose the first LDP framework for a variety of location-based services (namely ''L-SRR''), which privately collects and analyzes user locations with high utility. Specifically, we design a novel randomization mechanism ''Staircase Randomized Response'' (SRR) and extend the empirical estimation to significantly boost the utility for SRR in different LBS applications (e.g., traffic density estimation, and k-nearest neighbors). We have conducted extensive experiments on four real LBS datasets by benchmarking with other LDP schemes in practical applications. The experimental results demonstrate that L-SRR significantly outperforms them.
更多查看译文
AI 理解论文
溯源树
样例
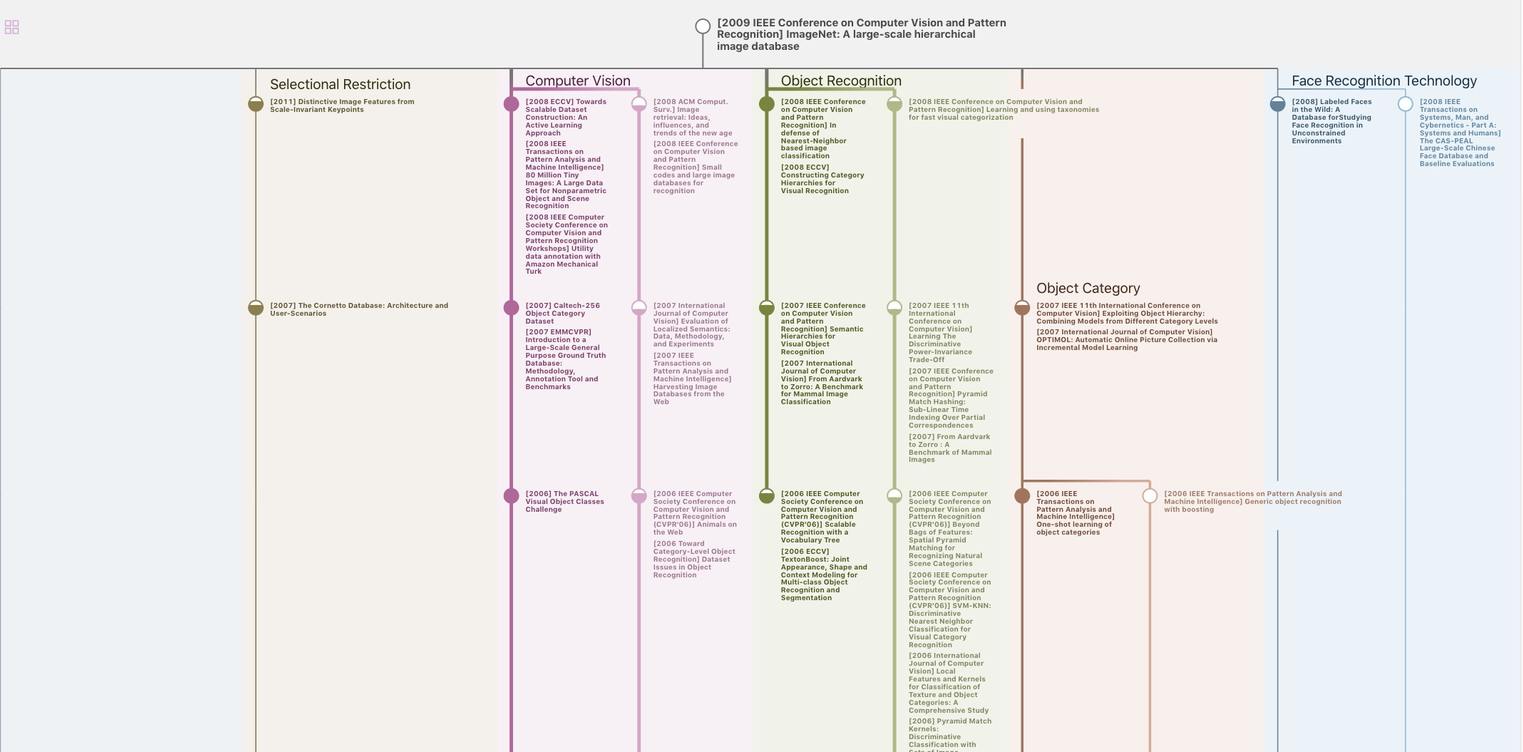
生成溯源树,研究论文发展脉络
Chat Paper
正在生成论文摘要