Global Attention-Based Graph Neural Networks for Node Classification
Neural Processing Letters(2022)
摘要
Graph attention networks (GATs) is an important method for processing graph data. The traditional GAT method can extract features from neighboring nodes, but the abilities of obtaining features from distant nodes and learning the global structure of the graph are insufficient. To tackle this issue, a new model Global Attention-based Graph Neural Networks (GA-GNN) has been proposed, with two improvements aimed at improving the global feature learning ability. Firstly, in order to improve the efficiency of global information acquisition, the basic graph structure is obtained by K -means clustering method, and the training set is re-selected with wider influencing scope according to the obtained structure. Secondly, the relation influential features are designed to represent the influence between node groups, and added to the node feature to improve the global expression ability of nodes. The node classification task performed on three citation network datasets shows that the performance of our proposed method is better than that of other baseline methods.
更多查看译文
关键词
Graph attention network,Global attention,Node classification,Clustering
AI 理解论文
溯源树
样例
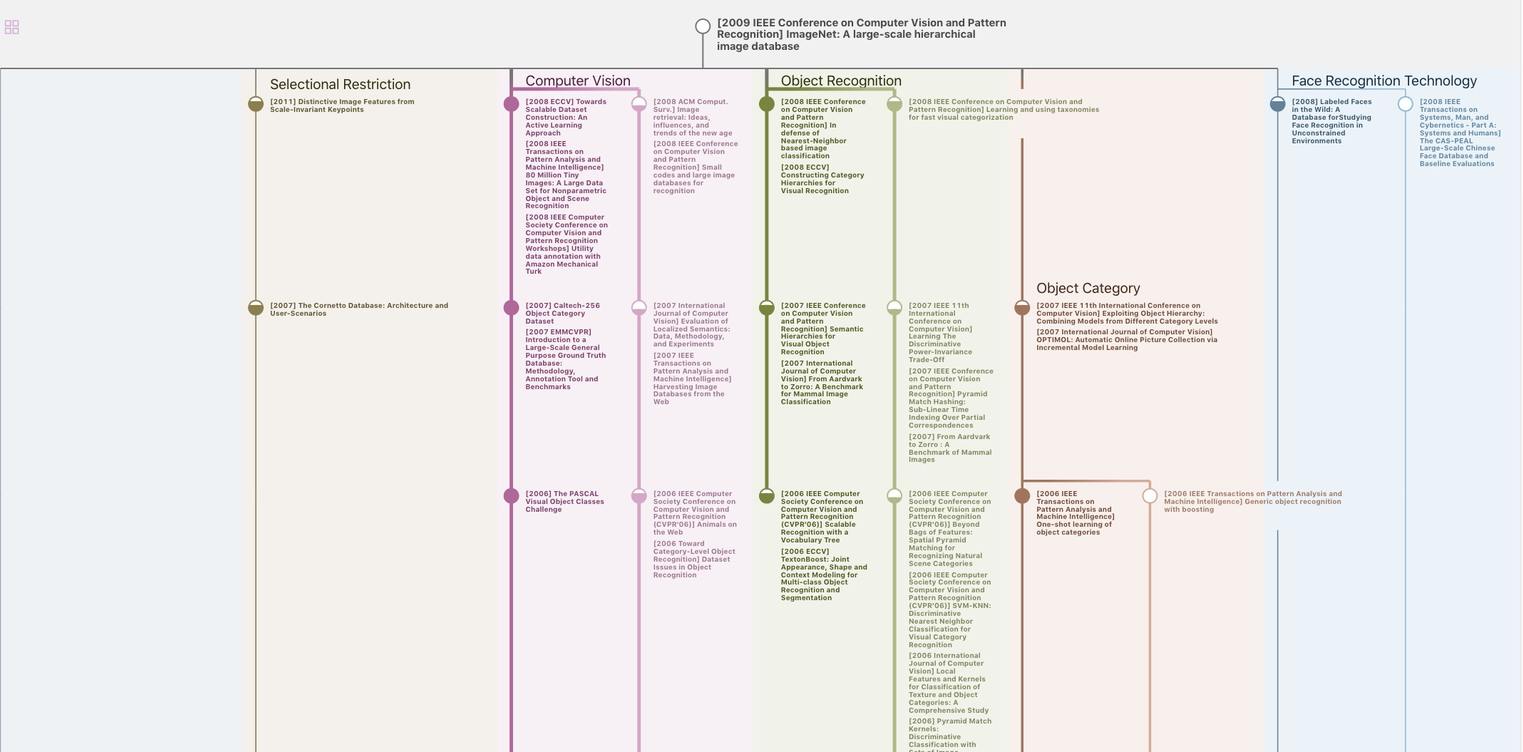
生成溯源树,研究论文发展脉络
Chat Paper
正在生成论文摘要