On Leveraging the Metapath and Entity Aware Subgraphs for Recommendation.
Proceedings of the 1st Workshop on Multimedia Computing towards Fashion Recommendation(2022)
摘要
In graph neural networks (GNNs), message passing iteratively aggregates nodes' information from their direct neighbours while neglecting the sequential nature of multi-hop node connections. Such sequential node connections e.g., metapaths, capture critical insights for downstream tasks. Concretely, in recommender systems (RSs), disregarding a larger neighbourhood and focusing only on the immediate neighbours leads to inadequate distillation of the collaborative signals. In this paper, we employ collaborative subgraphs (CSGs) and metapaths to form metapath-aware subgraphs, which explicitly capture sequential semantics in graph structures. We propose metaPath and Entity-Aware Graph Neural Network (PEAGNN), which trains GNN to perform metapath-aware information aggregation on such subgraphs. The information from different metapaths is then fused using attention mechanism. To leverage the local structure of CSGs, we introduce entity-awareness that acts as a contrastive regularizer on node embedding. Moreover, PEAGNN can be combined with prominent layers such as GAT, GCN and GraphSage. Our empirical evaluation shows that our proposed approach outperforms competitive baselines on three public datasets. Further analysis demonstrates that PEAGNN also learns meaningful metapath combinations from a given set of metapaths.
更多查看译文
AI 理解论文
溯源树
样例
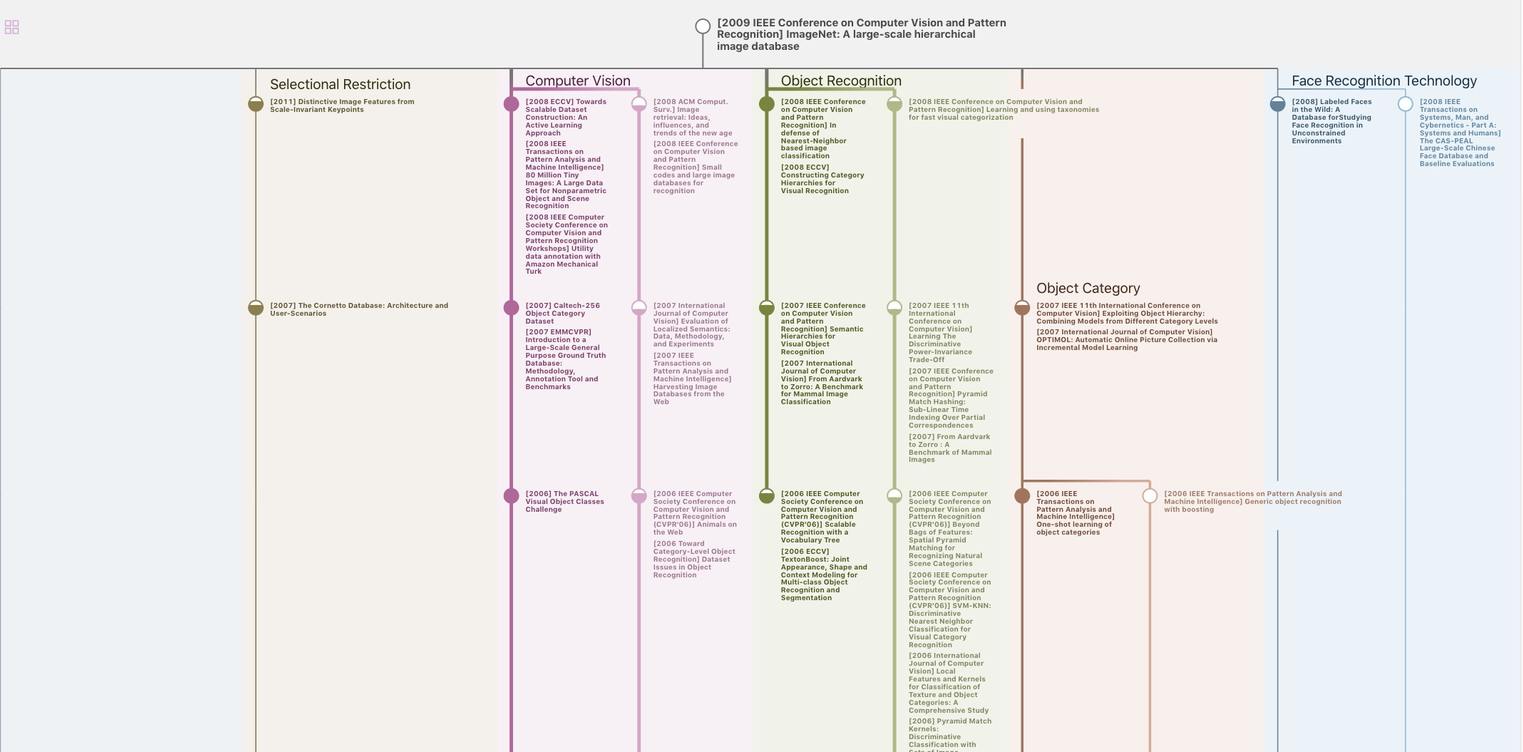
生成溯源树,研究论文发展脉络
Chat Paper
正在生成论文摘要