Small-target weed-detection model based on YOLO-V4 with improved backbone and neck structures
SSRN Electronic Journal(2023)
摘要
In field weed detection tasks, achieving accurate identification of crops and weeds is the primary target. However, since small target weeds among crops are not easily detected, this undoubtedly increases the difficulty of detection. In order to solve this problem, based on the YOLO-V4 network, this paper modifies the residual block of the backbone network into a Res2block residual block with a hierarchical residual mode, and constructs a new backbone network Csp2Darknet53 to enhance fine-grained feature detection; In addition, receptive field enlargement and multi-scale fusion are achieved by using the I-SPP structure with multi-branch structure and dilated convolution; Finally, a depthwise separable convolution block with residual mode (IDSC-X ) is proposed to replace the original 5-time convolution block in the path aggregation network (PANet) to ensure that the original features are not completely lost and reduce the amount of parameters. Compared with FasterR-CNN, SSD, MaskR-CNN, YOLO-V3 and YOLO-V4, the improved network detection accuracy is significantly better than other networks. Compared with YOLO-V4, the AP value of small target weeds increased by 15.1%, the mAP value increased by 4.2%, and the model parameters and training weight file size decreased by 34%. The results show that the method is feasible to improve the accurate detection of small target weeds, and can be extended to weed detection tasks of different crops.
更多查看译文
关键词
Weed detection,YOLO-V4 network,Small target,Different crops
AI 理解论文
溯源树
样例
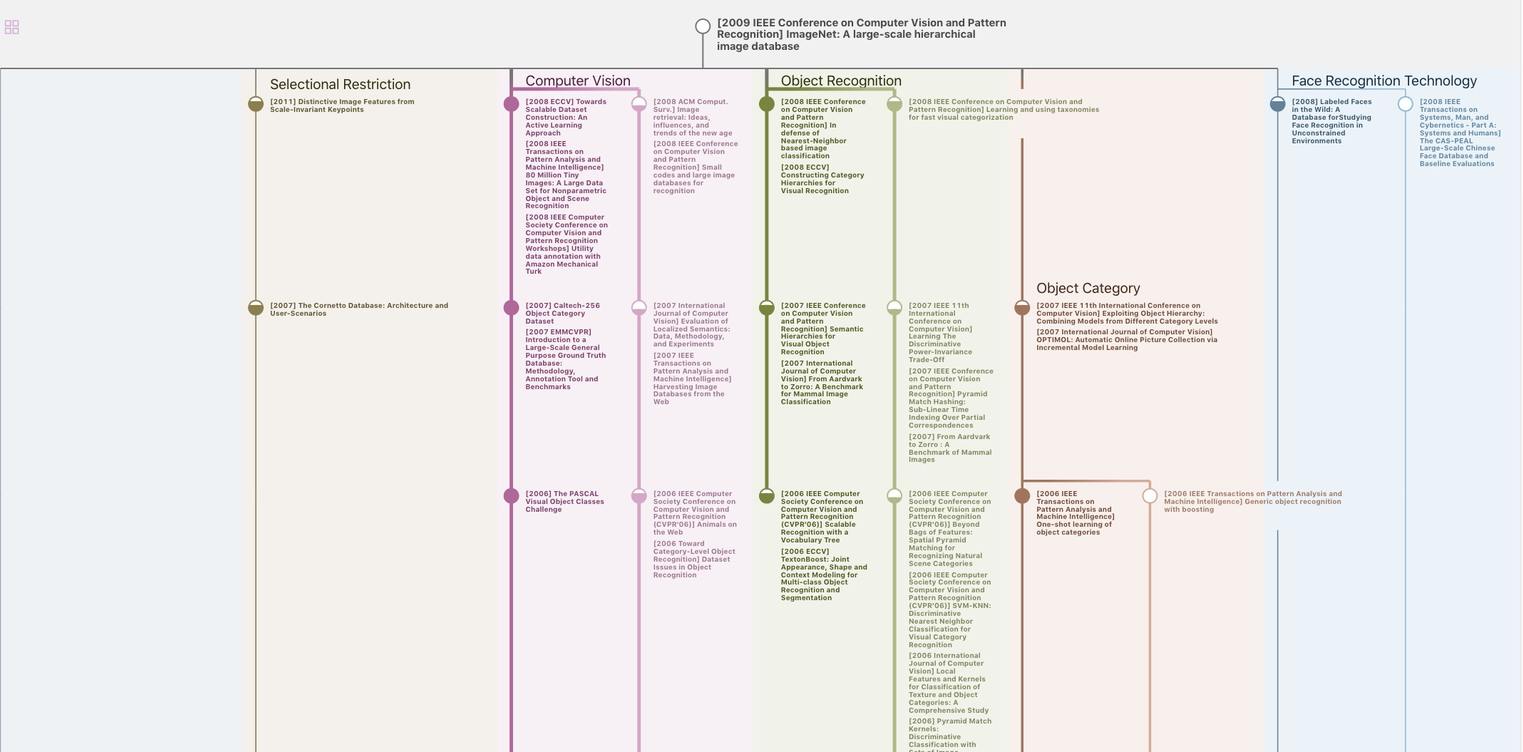
生成溯源树,研究论文发展脉络
Chat Paper
正在生成论文摘要