More efficient fully Bayesian adaptive testing with a revised proposal distribution
Behaviormetrika(2022)
摘要
The present study improved the efficiency of the fully Bayesian algorithm for adaptive testing proposed in van der Linden and Ren (2020) by revising the proposal distribution used in the Metropolis–Hastings sampler. The revised algorithm was found to require considerably fewer iterations for convergence as compared to the original algorithm. Moreover, the ability estimates of the revised algorithm were similar to or more accurate than the original algorithm. Compared with the conventional CAT algorithm that treats the item parameter estimates as fixed and ignores the estimation error, both the revised and original fully Bayesian algorithms led to better estimation accuracy at the lower end of ability scale.
更多查看译文
关键词
CAT, IRT, Bayesian, MCMC algorithm
AI 理解论文
溯源树
样例
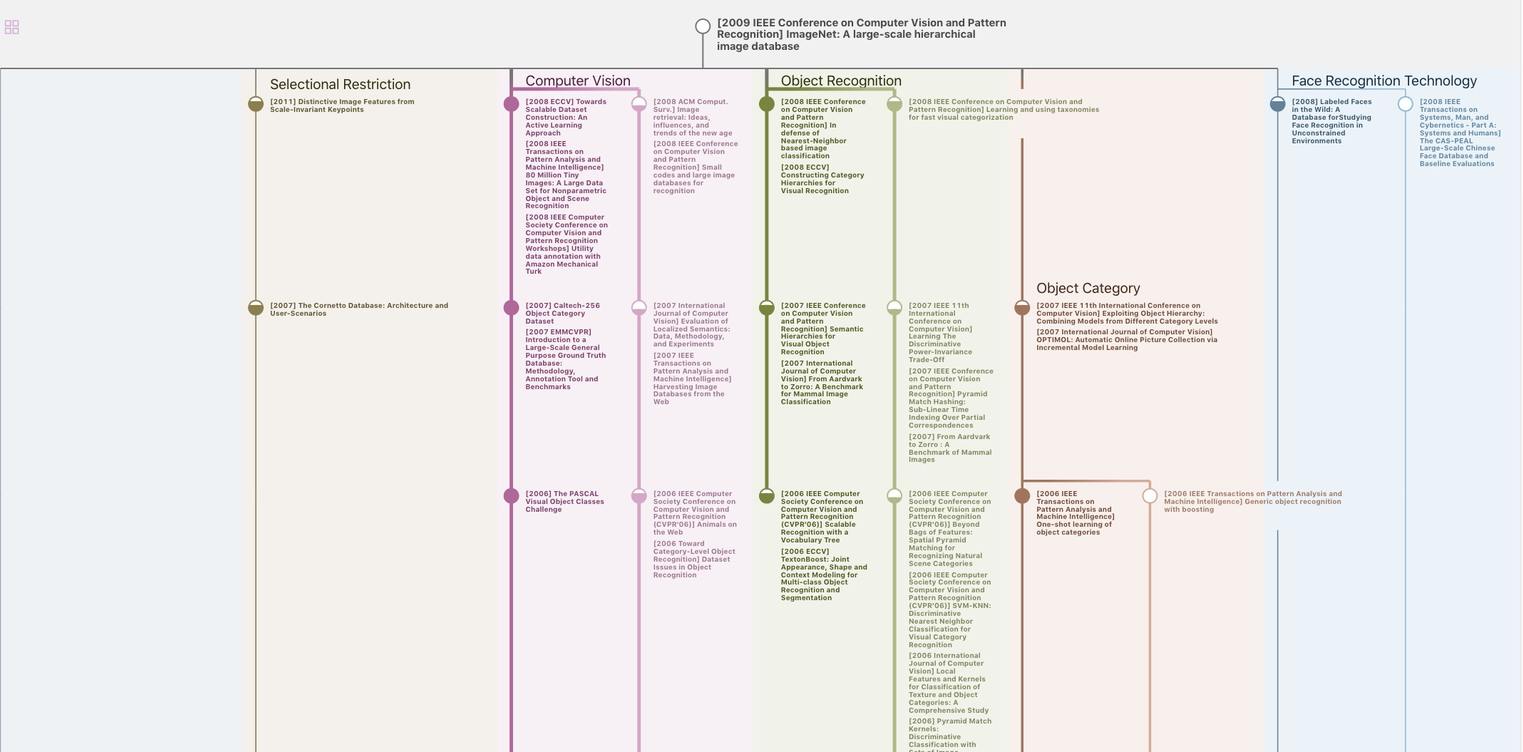
生成溯源树,研究论文发展脉络
Chat Paper
正在生成论文摘要