Enforcing Geometrical Priors in Deep Networks for Semantic Segmentation Applied to Radiotherapy Planning
Journal of Mathematical Imaging and Vision(2022)
摘要
Incorporating prior knowledge into a segmentation process, whether it is geometrical constraints such as volume penalisation, (partial) convexity enforcement, or topological prescriptions to preserve the contextual relations between objects, proves to improve accuracy in medical image segmentation, in particular when addressing the issue of weak boundary definition. Motivated by this observation, the proposed contribution aims to provide a unified variational framework including geometrical constraints in the training of convolutional neural networks in the form of a penalty in the loss function. These geometrical constraints take several forms and encompass level curve alignment through the integration of the weighted total variation, an area penalisation phrased as a hard constraint in the modelling, and an intensity homogeneity criterion based on a combination of the standard Dice loss with the piecewise constant Mumford–Shah model. The mathematical formulation yields a non-smooth non-convex optimisation problem, which rules out conventional smooth optimisation techniques and leads us to adopt a Lagrangian setting. The application falls within the scope of organ-at-risk segmentation in CT (computed tomography) images, in the context of radiotherapy planning. Experiments demonstrate that our method provides significant improvements (i) over existing non-constrained approaches both in terms of quantitative criteria, such as the measure of overlap, and qualitative assessment (spatial regularisation/coherency, fewer outliers), (ii) over in-layer constrained deep convolutional networks, and shows a certain degree of versatility.
更多查看译文
关键词
Image segmentation,Deep learning,Proximal operator,ADMM algorithm,Douglas–Rachford algorithm,Primal–dual algorithm
AI 理解论文
溯源树
样例
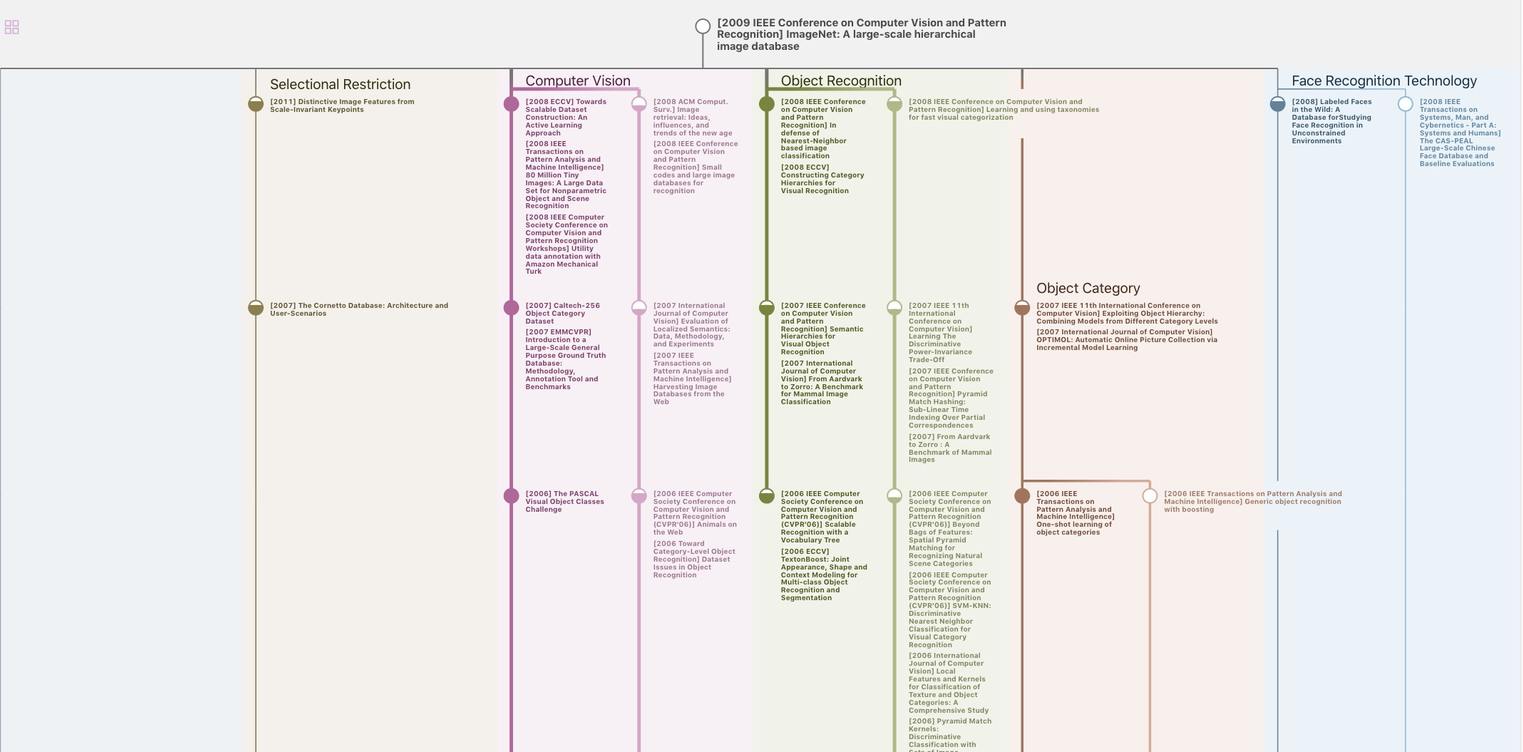
生成溯源树,研究论文发展脉络
Chat Paper
正在生成论文摘要