Private Federated Learning With Misaligned Power Allocation via Over-the-Air Computation
IEEE Communications Letters(2022)
摘要
To further preserve the data privacy of federated learning (FL), we propose a differentially private FL (DPFL) scheme with misaligned power allocation (MPA-DPFL). Unlike most existing over-the-air FL studies, in MPA-DPFL, the gradients are aggregated through over-the-air computation (Aircomp) but do not need to be aligned in the transmission. Therefore, MPA-DPFL can avoid the problem that the signal-to-noise ratio (SNR) of the system is limited by the device with the worst channel condition. We formulate an optimization problem to minimize the optimality gap of MPA-DPFL while guaranteeing a certain degree of privacy protection. Additionally, we demonstrate that the MPA-DPFL is more suitable than the DPFL with aligned power allocation (APA-DPFL) when the channel condition of a device in the system is lower than a threshold. The analytical results are validated through simulation.
更多查看译文
关键词
Data privacy,federated learning,over-the-air computation,power allocation
AI 理解论文
溯源树
样例
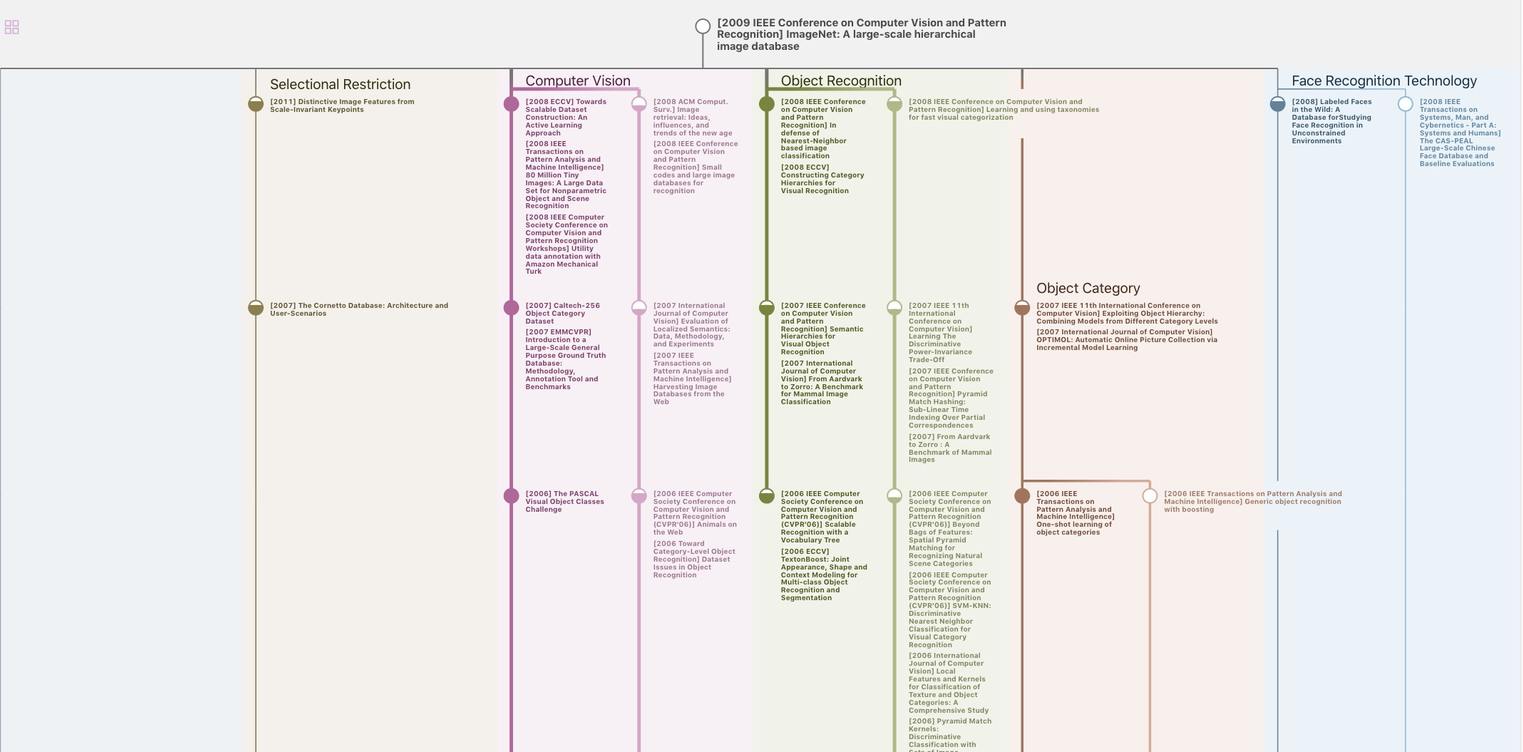
生成溯源树,研究论文发展脉络
Chat Paper
正在生成论文摘要