Transfer Learning Featured Short-Term Combining Forecasting Model for Residential Loads With Small Sample Sets
IEEE Transactions on Industry Applications(2022)
摘要
In recent years, a large variety of short-term load forecasting methodologies have been proposed, but a common drawback of them is that require a large amount of historical data to train the model. However, in reality, the cases in limited historical data would be more frequently occurred, for example, a new house or substation is built. To tackle this issue, a combining forecasting method of deep residual neural network (ResNet)-based transfer learning for residential loads is proposed in this article. To improve the accuracy and reliability of transfer learning, a novel deep ResNet with dual skip connections (ResNet-DSC) is proposed as the base model. Then with sparse training data, a Bayesian probability weighted averaging (approach is proposed, to address the model combination parameters estimation problem. In addition, probability density function forecasting is also delivered by the method. For demonstration, a series of experiments, with actual residential load data, was performed. With the comparison with other transfer learning and nontransfer learning approaches, the effectiveness and improvement of the proposed method have been validated.
更多查看译文
关键词
Few-shot learning,probability density function (PDF) forecasting,ResNet-DSC,short-term residential load forecasting (STRLF),transfer learning
AI 理解论文
溯源树
样例
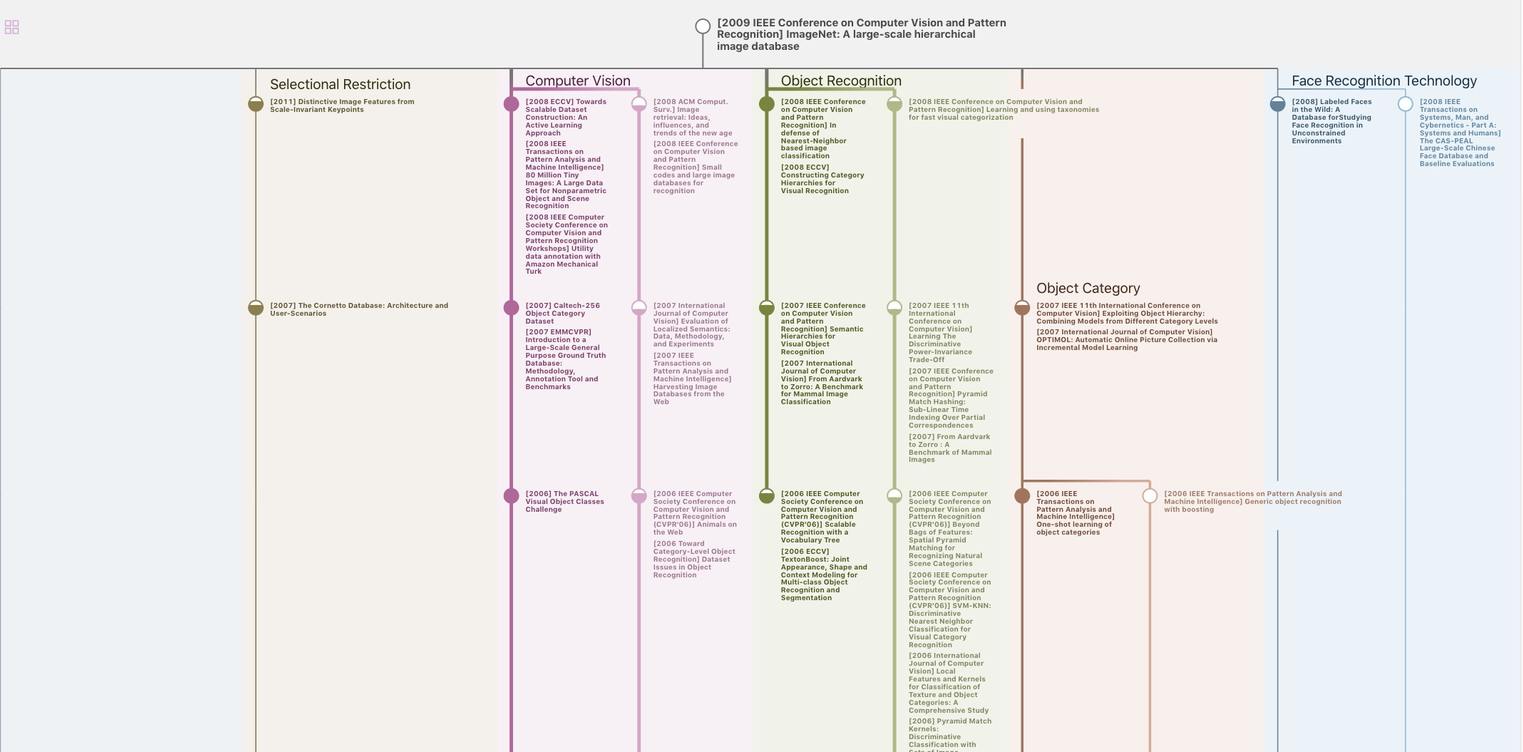
生成溯源树,研究论文发展脉络
Chat Paper
正在生成论文摘要