DualNER: A Trigger-Based Dual Learning Framework for Low-Resource Named Entity Recognition
IEEE Intelligent Systems(2022)
摘要
Most of the existing named entity recognition methods require a large amount of annotated data to obtain good performance. Once the annotated data are insufficient, the performance of these methods will degrade significantly. However, data annotation is time-consuming and laborious work. Therefore, how to achieve better performance with small amounts of annotated data in the named entity recognition task is an urgent task to be solved. In real scenarios, human beings always rely on some other words in the sentence when they recognize named entities, these words are called triggers. Motivated by this, we first verified the effectiveness of entity triggers for named entity recognition, then constructed a dual learning framework for low-resource named entity recognition based on the duality of entity triggers and corresponding entity, termed DualNER. Experiments show that the proposed methods use 20% trigger-entity annotated data and can achieve comparable results with the conventional model, which is trained by 50%–70% conventional annotated data.
更多查看译文
AI 理解论文
溯源树
样例
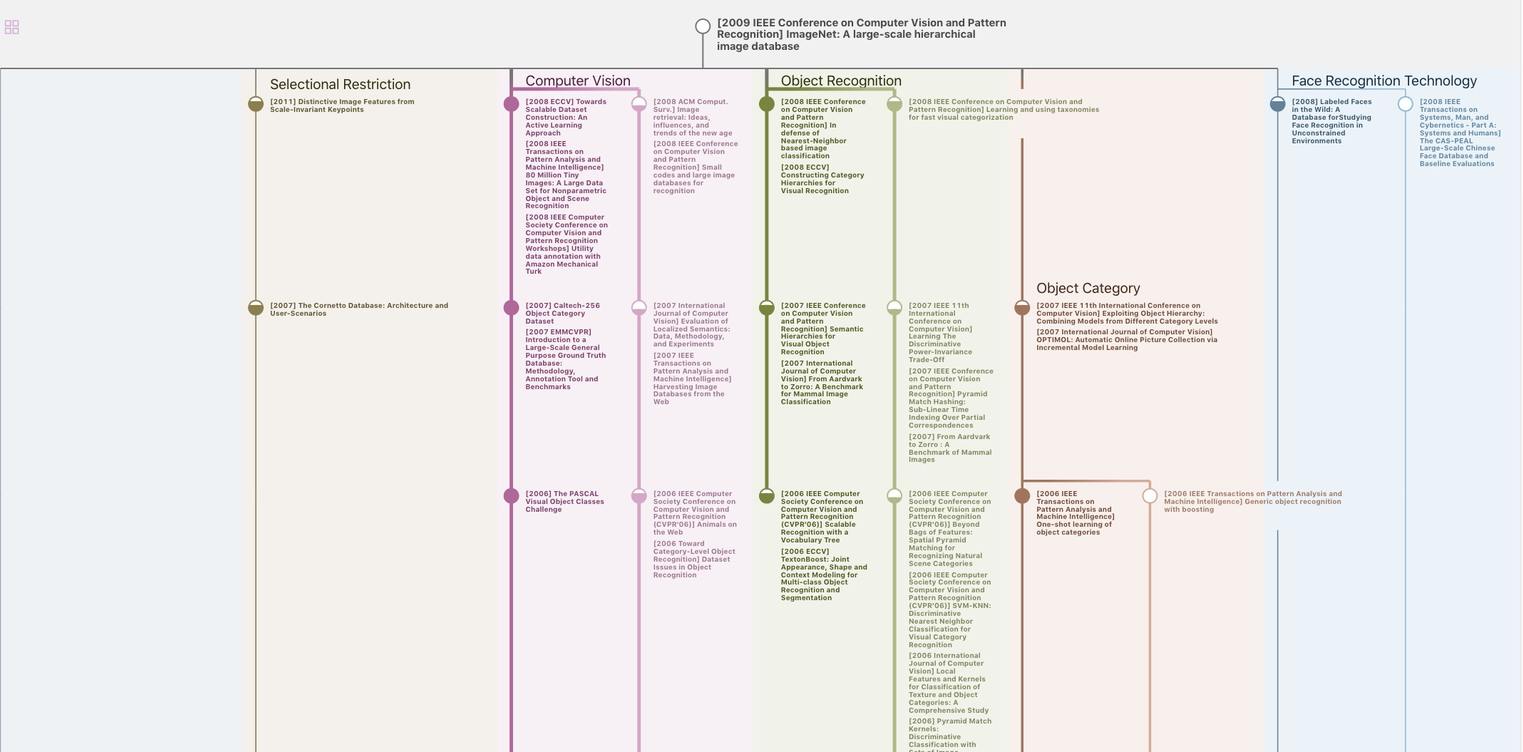
生成溯源树,研究论文发展脉络
Chat Paper
正在生成论文摘要