Comparison of Real-time Control Methods for CSO Reduction with Two Evaluation Indices: Computing Load Rate and Double Baseline Normalized Distance
Water Resources Management(2022)
摘要
Real-time control (RTC) methods, which utilize real-time information to control the existing infrastructures in combined sewer systems, are effective in reducing combined sewer overflows (CSOs). However, it is difficult to compare the performance of RTC systems due to their diverse frameworks and application scenarios. This study provides a comparison of different RTC strategies through two proposed evaluation indices: computing load rate ( CLR ) and double-baseline normalized distance ( DBND ). CLR represents the computing load as a percentage of the control step interval, while DBND indicates the control effect normalized by the lower and upper bounds of the control process. Three different RTC methods, heuristic control (HC), model predictive control (MPC), and reinforcement learning control (RLC), were compared and studied through the two indices. In this study, RLC trains an artificial intelligence agent to regulate sewage pumps during rainfall events for CSO reduction. A combined sewer system in eastern China is taken as a case study. According to the simulation results and indices: (i) CLR is an effective index for the computing cost and efficiency evaluation of diverse RTC systems. (ii) DBND enables the comparison of control effects between RTC systems that differ in rainfall events and the capacities of combined sewer systems. (iii) HC, MPC, and RLC vary in computing time costs and control effects, and among them, RLC is the most cost-effective control method.
更多查看译文
关键词
Combined sewer overflow,Real-time control,Reinforcement learning,Heuristic control,Model predictive control
AI 理解论文
溯源树
样例
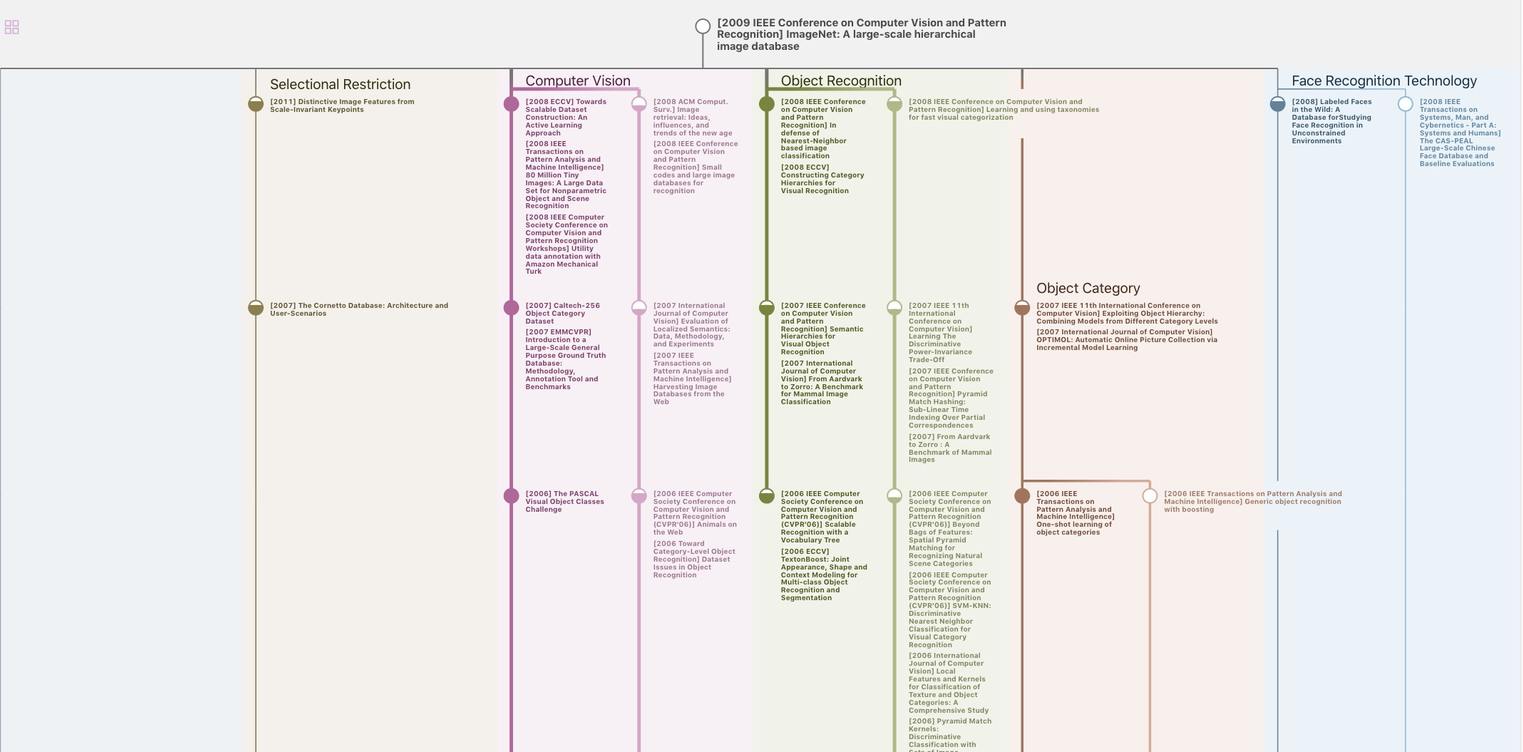
生成溯源树,研究论文发展脉络
Chat Paper
正在生成论文摘要