Image Abstraction Framework as a Pre-processing Technique for Accurate Classification of Archaeological Monuments Using Machine Learning Approaches
SN Computer Science(2021)
摘要
This work extricates the image characteristic features for the classification of archeological monument images. At the pre-processing stage, archeological dataset sample images are treated by using structure safeguarding image abstraction framework, which can deliver the most effective image abstraction output by manipulating the perceptible features in the given low-illuminated and underexposed color image samples. Proposed abstraction-framework effectively boosted the significant image property features like color, edge, sharpness, contrast and suppresses complexity and noise. The image properties were also refined at each phase based on the attained statistical feature disposal information. The work adopted the Harris feature identification technique to identify the most significant image features in the input and enhanced images. The framework also preserves significant features in the foreground of an image by intelligently integrating the series of filters during rigorous experimental work and also diminishes the background content of an input image. The proposed archeological system evaluates every stage of the result with assorted subjective matters and calculates the image quality and properties assessment statistical attributes. By this way prominent features in an image have been recognized. The efficiency of this work has been corroborated by performing the trials on the selected archeological dataset. In addition, user’s visual feedback and the standard image quality assessment techniques were also used to evaluate the proposed pre-processing framework. Based on the obtained abstraction images from the framework, this work extracts the image gray color texture features using GLCM, color texture from CTMs and deep-learning features from AlexNet for the classification of archeological monument classification. This work adopted a support vector machine as a classifier. To corroborate the efficiency of the proposed method, an experiment was conducted on our own data set of Chalukya, Kadamba, Hoysala and new engraving monuments, each domain consisting of 500 archeological data set samples with large intra-class variation, with different environmental lighting condition, low-illumination and different pose. Implementation of this work was carried out in MATLAB-2020 with HPC Nvidia Tesla P100 GPU, and obtained results show that combination of multiple features significantly improves the performance to the extent of 98.10%.
更多查看译文
关键词
Non-photorealistic rendering (NPR), Image abstraction, Denoising convolutional neural network (DnCNN), Weighted gradient sticks filtering (WGSF), Anisotropic diffusion-Kuwahara filtering (ADKF), Gray-level co-occurrence matrix (GLCM), Color texture moments (CTMs)
AI 理解论文
溯源树
样例
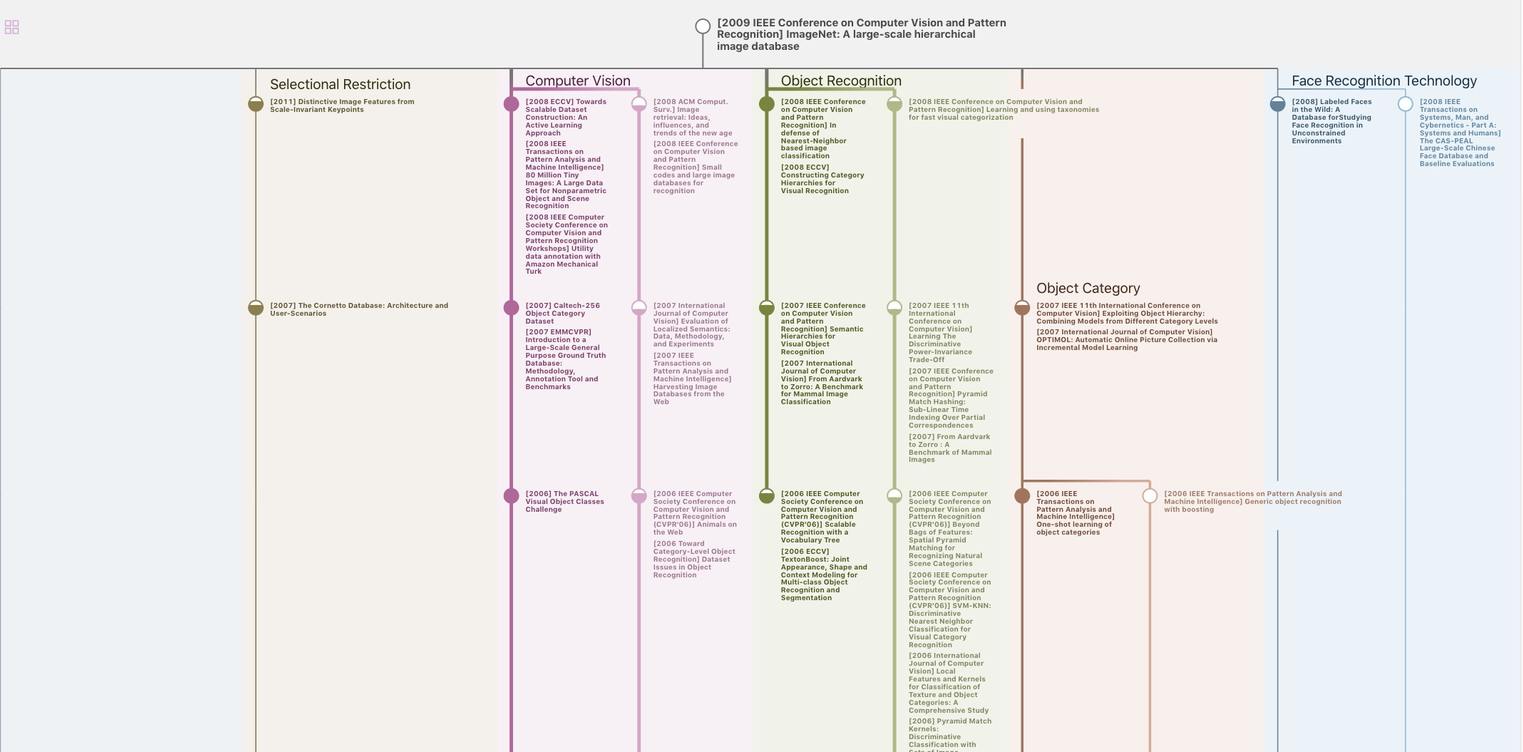
生成溯源树,研究论文发展脉络
Chat Paper
正在生成论文摘要