Edge-Preserving Multiframe Image Super-Resolution Methods Under Anisotropic Diffusion Framework
SN Computer Science(2022)
摘要
Anisotropic diffusion has demonstrated an outstanding performance in several image processing and computer vision tasks. In image denoising, for instance, anisotropic diffusion simultaneously removes noise and preserves critical image features (edges, texture, and contours). Despite its promising performance, scholars have not evaluated different anisotropic diffusion functionals, especially those derived from robust statistics, to observe their effectiveness in addressing inverse problems. In this work, we propose an anisotropic diffusion functional and demonstrate its effectiveness in addressing the ill-posed nature of the multiframe super-resolution problem. Subjective and objective evaluation metrics show that, compared to the existing functionals, the proposed functional outperforms, giving higher values of peak-signal-to-noise ratio and structural similarity indices. Furthermore, derivations and formulations are given to guide scholars on the design of optimization problems for multiframe super-resolution under the anisotropic diffusion framework.
更多查看译文
关键词
Anisotropic diffusion, Multiframe super-resolution, Observation model, Image denoising, Resolution enhancement
AI 理解论文
溯源树
样例
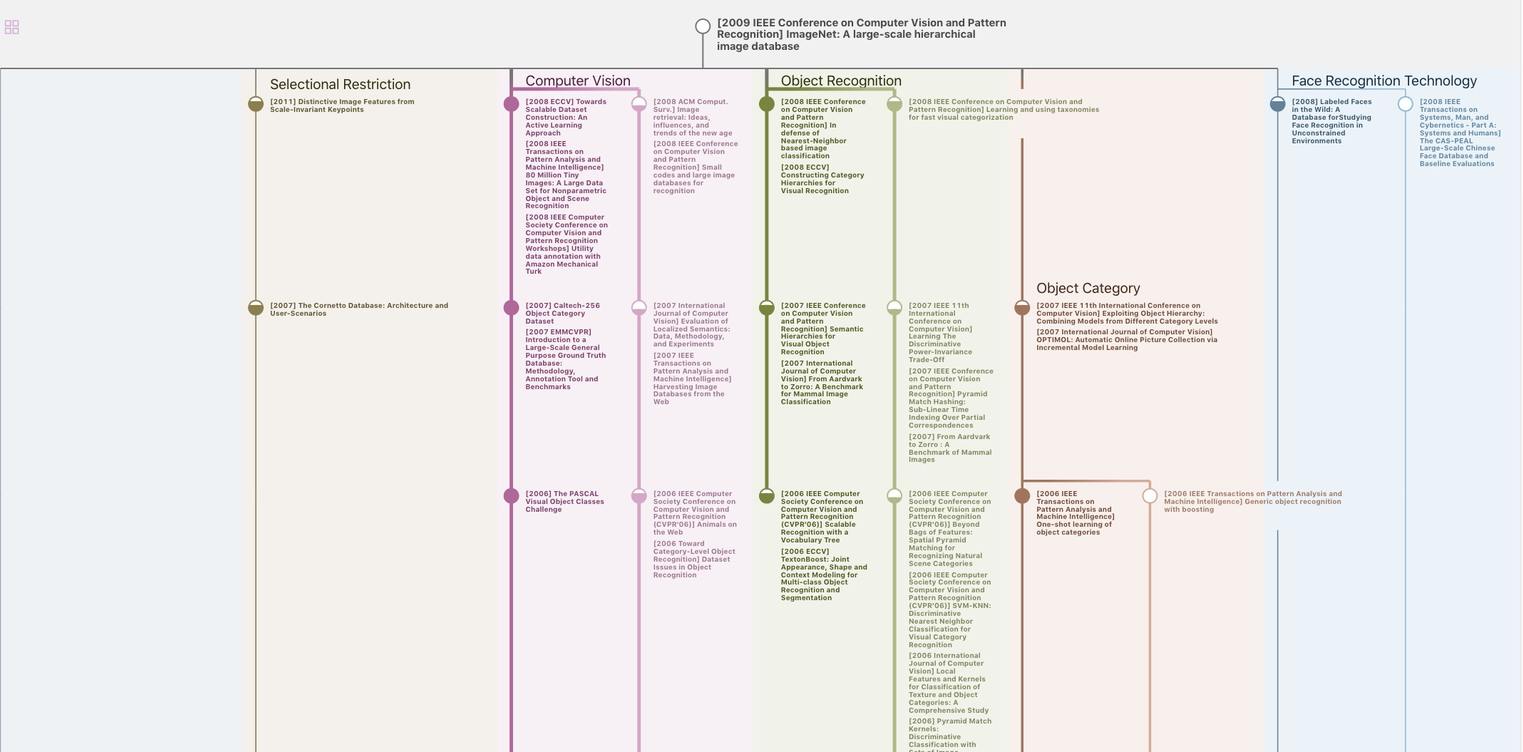
生成溯源树,研究论文发展脉络
Chat Paper
正在生成论文摘要