Residual RNN Models With Pruning for Digital Predistortion of RF Power Amplifiers
IEEE Transactions on Vehicular Technology(2022)
摘要
The paper presents several novel residual recurrent neural network (RNN) models for digital predistortion of radio-frequency power amplifiers. Then, unstructured and structured neural network (NN) pruning algorithms are proposed to reduce the per-sample computational complexity and the required memory, without compromising their modeling performance. The mathematical equations of the complexity and the memory are derived to analyze the hardware cost and design low cost models. An experimental platform is developed to measure the modeling performance in terms of adjacent channel leakage ratio (ACLR), normalized mean square error, error vector magnitude (EVM) and bit error rate. The platform uses an envelope tracking PA excited by 80 MHz IEEE 802.11ac signals. Compared with the state-of-the-art RNN model in (Sun
et al.
, 2019), the proposed models reduce the per-sample complexity and required memory by
$94\hbox{--}99$
%, they also improve the ACLR and EVM by at least 3 dB and 1 dB at 0.45 dB and 3.15 dB gain compression, respectively. Furthermore, an observation path with time alignment is used to remove the transmit latency in the state-of-the-art approach. At 3.15 dB gain compression, the proposed models outperform the state-of-the-art feedforward and convolutional NN models by at least 1.3 dB in EVM, outperform the general memory polynomial model by at least 3.3 dB in EVM. The above evidences indicate that the proposed RNN models can be implemented with relatively low hardware cost and achieve the best EVM in high power efficiency scenario.
更多查看译文
关键词
Power amplifier,digital predistortion,neural network (NN),long short-term memory (LSTM),LSTM projection,gated recurrent unit (GRU),NN pruning,error vector magnitude (EVM),IEEE 802.11
AI 理解论文
溯源树
样例
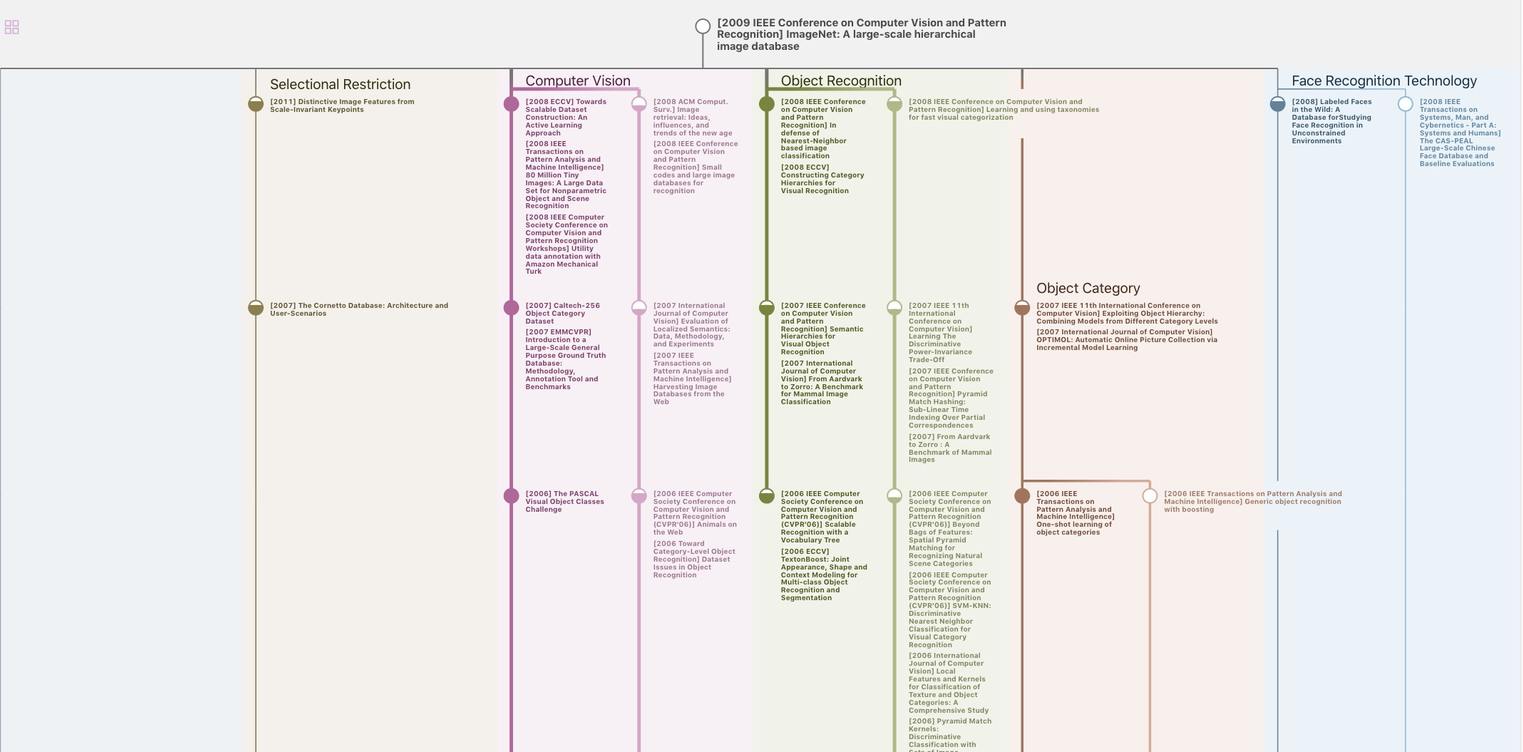
生成溯源树,研究论文发展脉络
Chat Paper
正在生成论文摘要