Learning Shape Distributions from Large Databases of Healthy Organs: Applications to Zero-Shot and Few-Shot Abnormal Pancreas Detection
Medical Image Computing and Computer Assisted Intervention – MICCAI 2022(2022)
摘要
We propose a scalable and data-driven approach to learn shape distributions from large databases of healthy organs. To do so, volumetric segmentation masks are embedded into a common probabilistic shape space that is learned with a variational auto-encoding network. The resulting latent shape representations are leveraged to derive zero-shot and few-shot methods for abnormal shape detection. The proposed distribution learning approach is illustrated on a large database of 1200 healthy pancreas shapes. Downstream qualitative and quantitative experiments are conducted on a separate test set of 224 pancreas from patients with mixed conditions. The abnormal pancreas detection AUC reached up to
$$65.41\%$$
in the zero-shot configuration, and
$$78.97\%$$
in the few-shot configuration with as few as 15 abnormal examples, outperforming a baseline approach based on the sole volume.
更多查看译文
关键词
Shape analysis, Anomaly detection, Pancreas
AI 理解论文
溯源树
样例
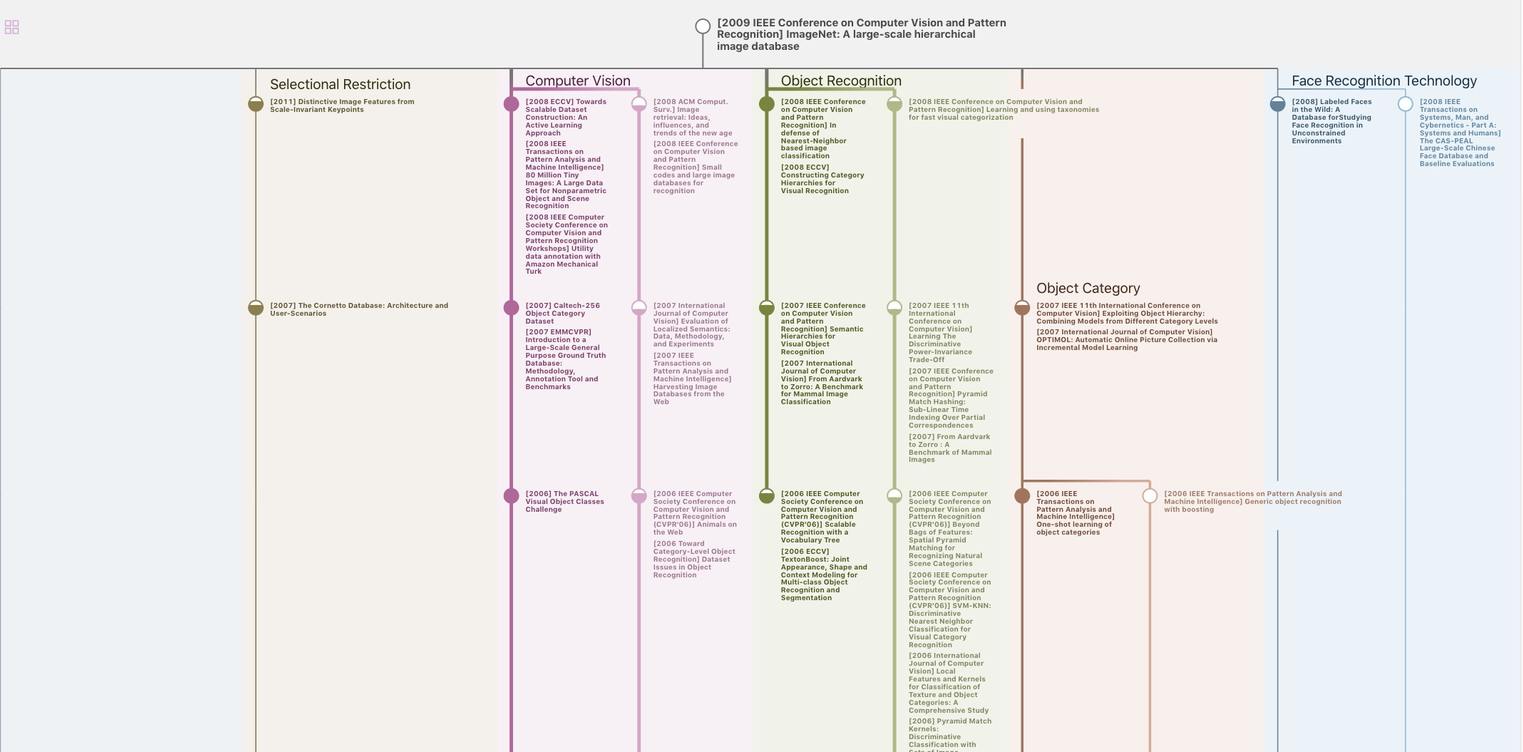
生成溯源树,研究论文发展脉络
Chat Paper
正在生成论文摘要