Fast Hard Clustering Based on Soft Set Multinomial Distribution Function
Recent Advances in Soft Computing and Data Mining(2022)
摘要
Categorical data clustering is still an issue due to difficulties/complexities of measuring the similarity of data. Several approaches have been introduced and recently the centroid-based approaches were introduced to reduce the complexities of the similarity of categorical data. However, those techniques still produce high computational times. In this paper, we proposed a clustering technique based on soft set theory for categorical data via multinomial distribution called Hard Clustering using Soft Set based on Multinomial Distribution Function (HCSS). The data is represented as a multi soft set where every soft set have its probability to be a member of the clusters. Firstly, the corrected proof is shown mathematically. Then, the experiment is conducted to evaluate the processing times, purity and rand index using benchmarks datasets. The experiment results show that the proposed approach have improve the processing times up to 95.03% by not compromising the purity and rand index as compared with baseline techniques.
更多查看译文
关键词
Clustering, Categorical data, Multi soft set, Multinomial distribution function
AI 理解论文
溯源树
样例
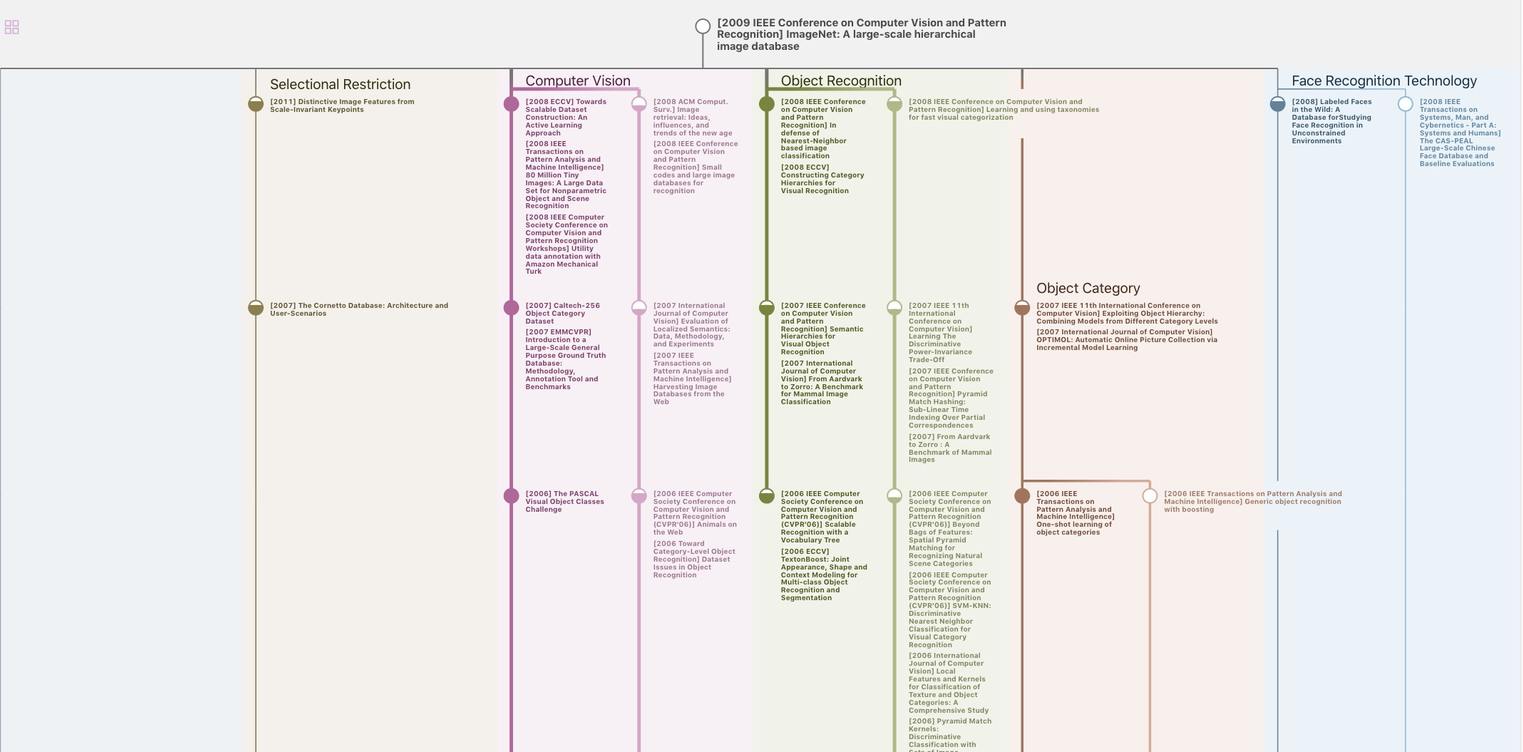
生成溯源树,研究论文发展脉络
Chat Paper
正在生成论文摘要