On the Limitations of Machine Learning (ML) Methodologies in Predicting the Wake Characteristics of Wind Turbines
Renewable Energy Systems in Smart Grid(2022)
摘要
Machine Learning (ML) algorithms have been more prevalent in recent years, and they are being used to tackle complicated issues across a broad range of fields. Wind energy is not an exception, as ML has recently been applied to wind turbine blade design, wake velocity and wake turbulence intensity prediction, and even wind farm optimization. The immense learning ability of ML models enables them to be trained to predict and regress a complex relationship with a high degree of accuracy. However, data for testing ML models often originate from the same rotor simulation used for training, with just slight variations in operating conditions. This research aims to investigate the generalizability of ML-based wake prediction models, i.e., whether ML can correctly predict wake properties using data from a different wind turbine that was not taken for training. This investigation's observation shows that a generalized ML wake model requires training data from multiple turbines with a wide range of operating conditions. In addition, advanced regularization, complex loss functions, and ML methods that focus on capturing the physics (such as Physics Informed Artificial Neural Networks (PINN) and symbolic regression) can be utilized.
更多查看译文
关键词
Machine learning, Wind turbines, Wake velocity, Turbulence intensity
AI 理解论文
溯源树
样例
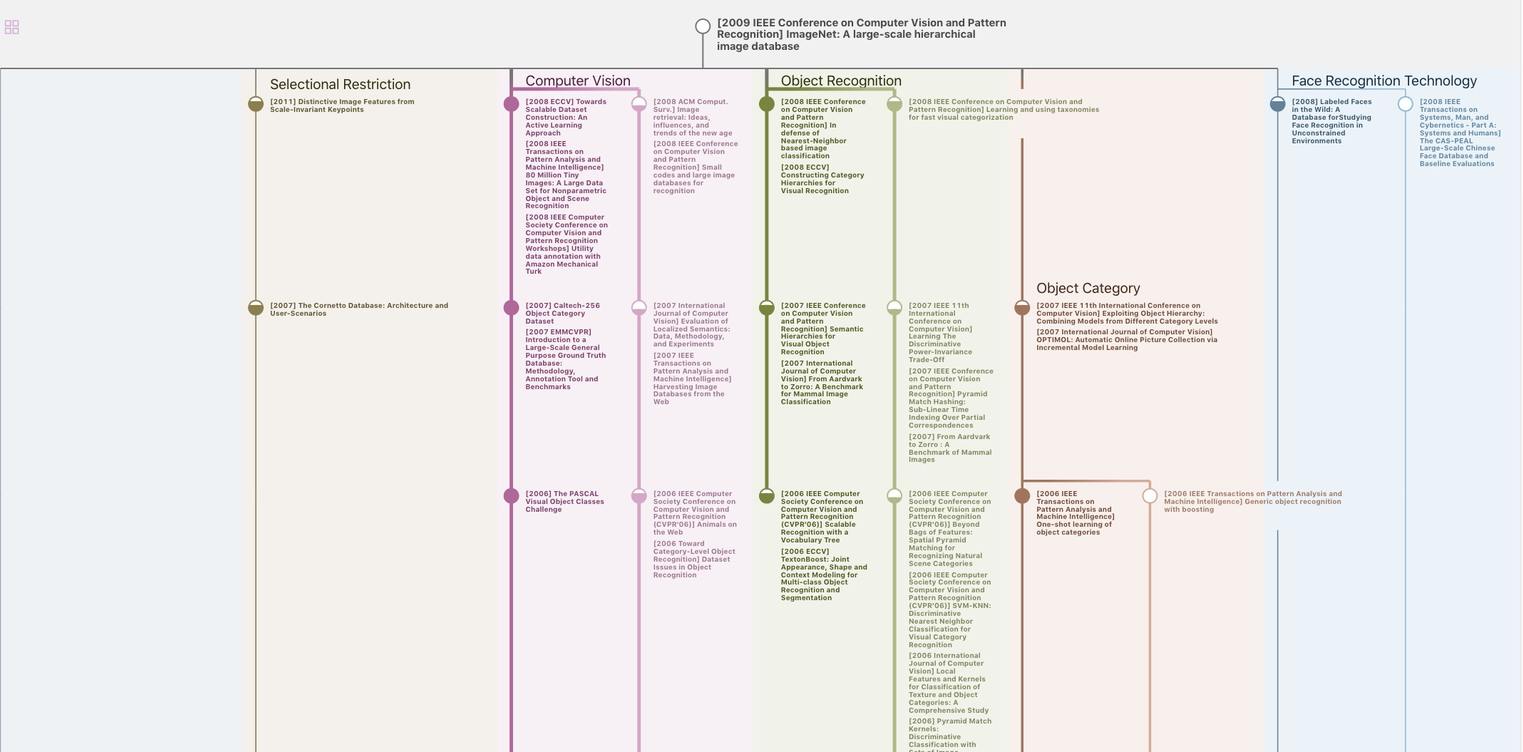
生成溯源树,研究论文发展脉络
Chat Paper
正在生成论文摘要