Cooperative Localization for Human Pose Estimation
Computer Supported Cooperative Work and Social Computing(2022)
摘要
The existing methods for human pose estimation are needed to predict either dense heatmap, which requires more complex model architecture, or to execute long-distance regression, which makes the model unable to achieve good performance. This work proposes an accurate and efficient deep learning framework for human pose estimation called cooperative localization. It uses a sparse heatmap to find the keypoint’s approximate location while two short-distance offsetmaps obtain its precise coordinates. To realize the framework, we construct two types of cooperative localization networks: CLNet-ResNet and CLNet-Hourglass. We evaluate the networks on three benchmark datasets: the Leeds Sports Pose dataset, the MPII Human Pose dataset, and the COCO keypoints detection dataset. Experimental results show that our framework consistently improves the average precision of their plain counterparts. The CLNet-ResNet50 outperforms SimpleBaseline by 1.14% with about 1/2 GFLOPs, and the CLNet-Hourglass outperforms the original stacked-hourglass by 4.45% on COCO.
更多查看译文
关键词
Human pose estimation, Cooperative localization, Deep learning
AI 理解论文
溯源树
样例
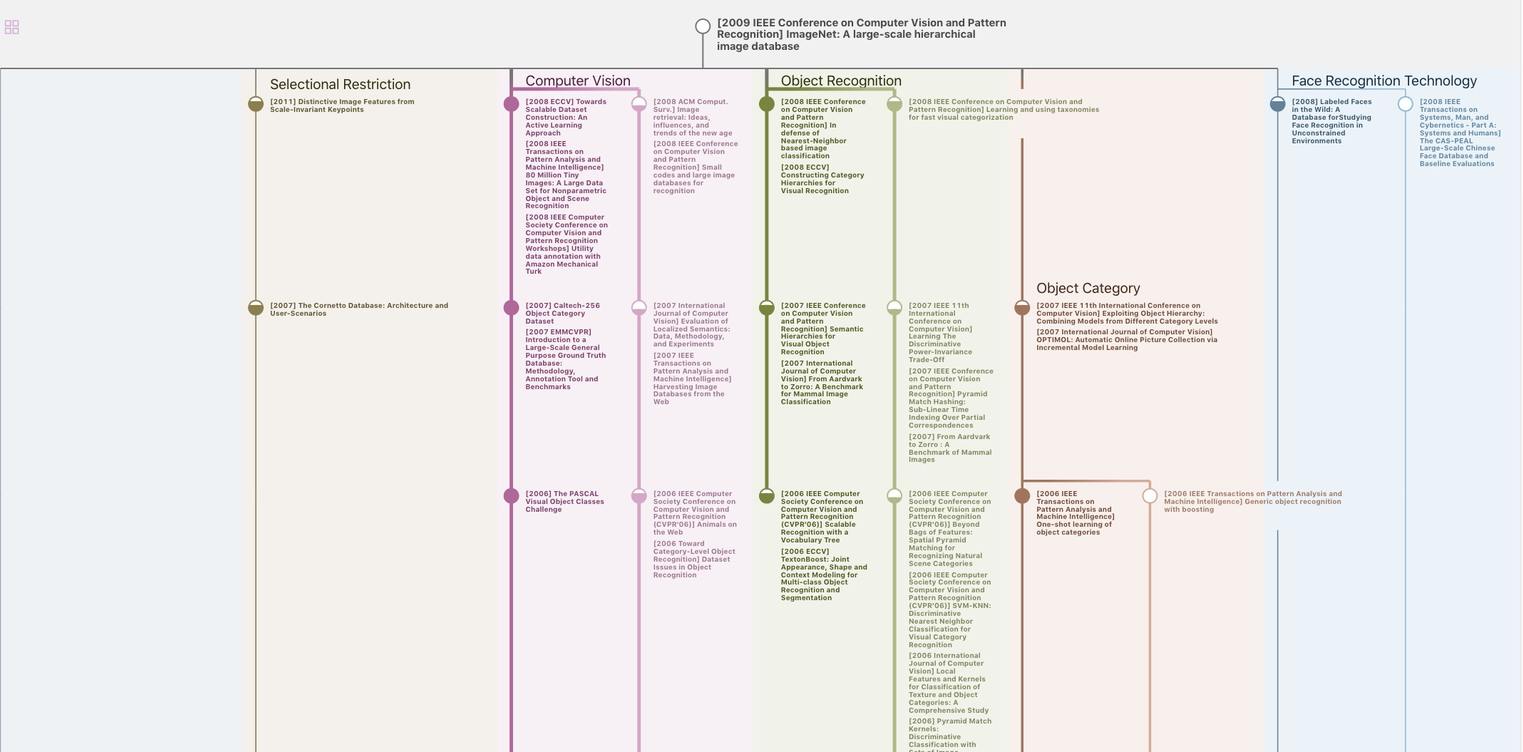
生成溯源树,研究论文发展脉络
Chat Paper
正在生成论文摘要