Self-supervised Multi-modality Image Feature Extraction for the Progression Free Survival Prediction in Head and Neck Cancer
Head and Neck Tumor Segmentation and Outcome Prediction(2022)
摘要
Long-term survival of oropharyngeal squamous cell carcinoma patients (OPSCC) is quite poor. Accurate prediction of Progression Free Survival (PFS) before treatment could make identification of high-risk patients before treatment feasible which makes it possible to intensify or de-intensify treatments for high- or low-risk patients. In this work, we proposed a deep learning based pipeline for PFS prediction. The proposed pipeline consists of three parts. Firstly, we utilize the pyramid autoencoder for image feature extraction from both CT and PET scans. Secondly, the feed forward feature selection method is used to remove the redundant features from the extracted image features as well as clinical features. Finally, we feed all selected features to a DeepSurv model for survival analysis that outputs the risk score on PFS on individual patients. The whole pipeline was trained on 224 OPSCC patients. We have achieved a average C-index of 0.7806 and 0.7967 on the independent validation set for task 2 and task 3. The C-indices achieved on the test set are 0.6445 and 0.6373, respectively. It is demonstrated that our proposed approach has the potential for PFS prediction and possibly for other survival endpoints.
更多查看译文
关键词
Progression free survival prediction, OPSCC, DeepSurv, Autoencoder, PET-scans and CT-scans
AI 理解论文
溯源树
样例
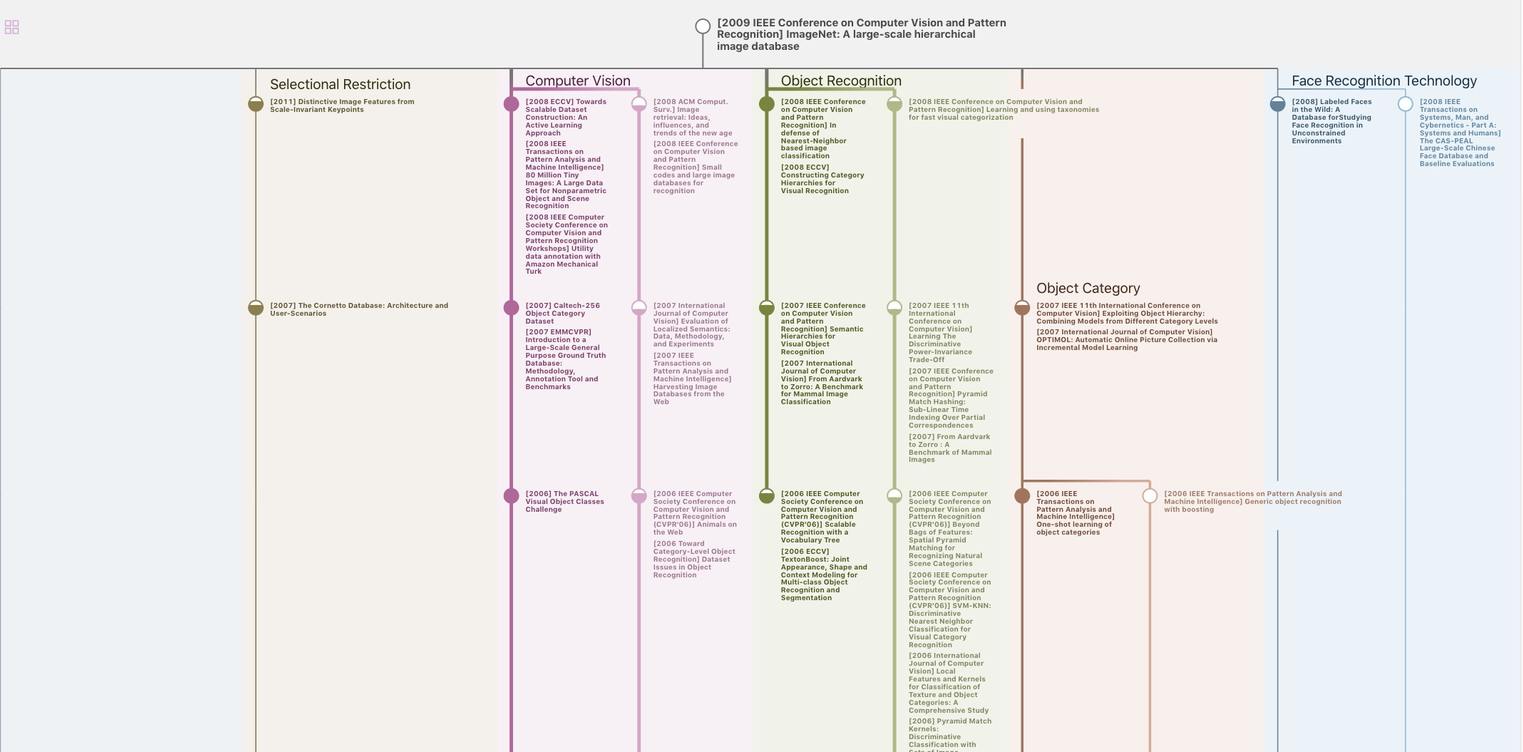
生成溯源树,研究论文发展脉络
Chat Paper
正在生成论文摘要