BERT-based Classifiers for Fake News Detection on Short and Long Texts with Noisy Data: A Comparative Analysis
Text, Speech, and Dialogue(2022)
摘要
Free uncontrolled access to the Internet is the main reason for fake news propagation on the Internet both in social media and in regular Internet publications. In this paper we study the potential of several BERT-based models to detect fake news related to politics. Our contribution to the area consists of testing BERT, RoBERTa and MNLI RoBERTa models with (a) short and long texts; (b) ensembling with the best models; (c) noisy texts. To improve ensembling, we introduce an additional class ‘Doubtful news’. To create noisy data we use cross-translation. For the experiments we consider the well-known FRN (Fake vs. Real News, long texts) and LIAR (short texts) datasets. The results we obtained on the long texts dataset are higher than the results we obtained on the short texts dataset. The proposed approach to ensembling provided significant improvement of the results. The experiments with noisy data demonstrated high noise immunity of the BERT model with long news and the RoBERTa model with short news.
更多查看译文
关键词
Fake News, BERT, RoBERTa, MNLI RoBERTa, Ensembling, Noise Immunity
AI 理解论文
溯源树
样例
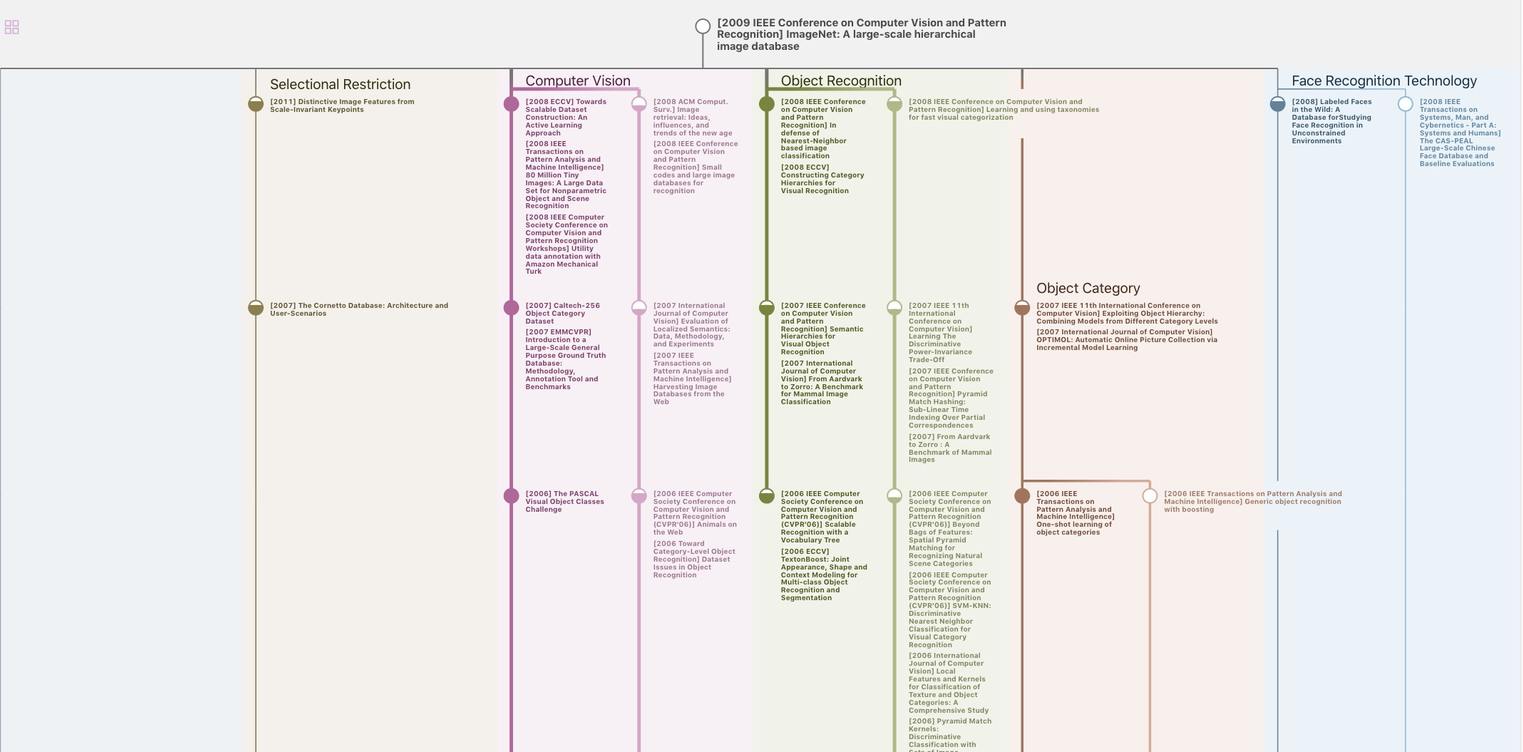
生成溯源树,研究论文发展脉络
Chat Paper
正在生成论文摘要