Learning-Based Optimization for Online Approximate Query Processing
Database Systems for Advanced Applications(2022)
摘要
Approximate query processing (AQP) technique speeds up query execution by reducing the amount of data that needs to be processed, while sacrificing the accuracy of the query result to some extent. AQP is essentially a trade-off between the accuracy of the query result and the query latency. However, the heuristic AQP optimization and error control mechanism used by the existing AQP system fails to meet the accuracy requirements of users. This paper proposes a deep learning-based error prediction model to guide AQP query optimization. By using this model, we can estimate the errors of candidate query plans and select the appropriate plans that can meet the accuracy requirement with high probability. Extensive experiments show that the AQP system proposed in this paper can outperform the state-of-the-art online sampling-based AQP approach.
更多查看译文
关键词
Approximate query processing, OLAP, Error prediction
AI 理解论文
溯源树
样例
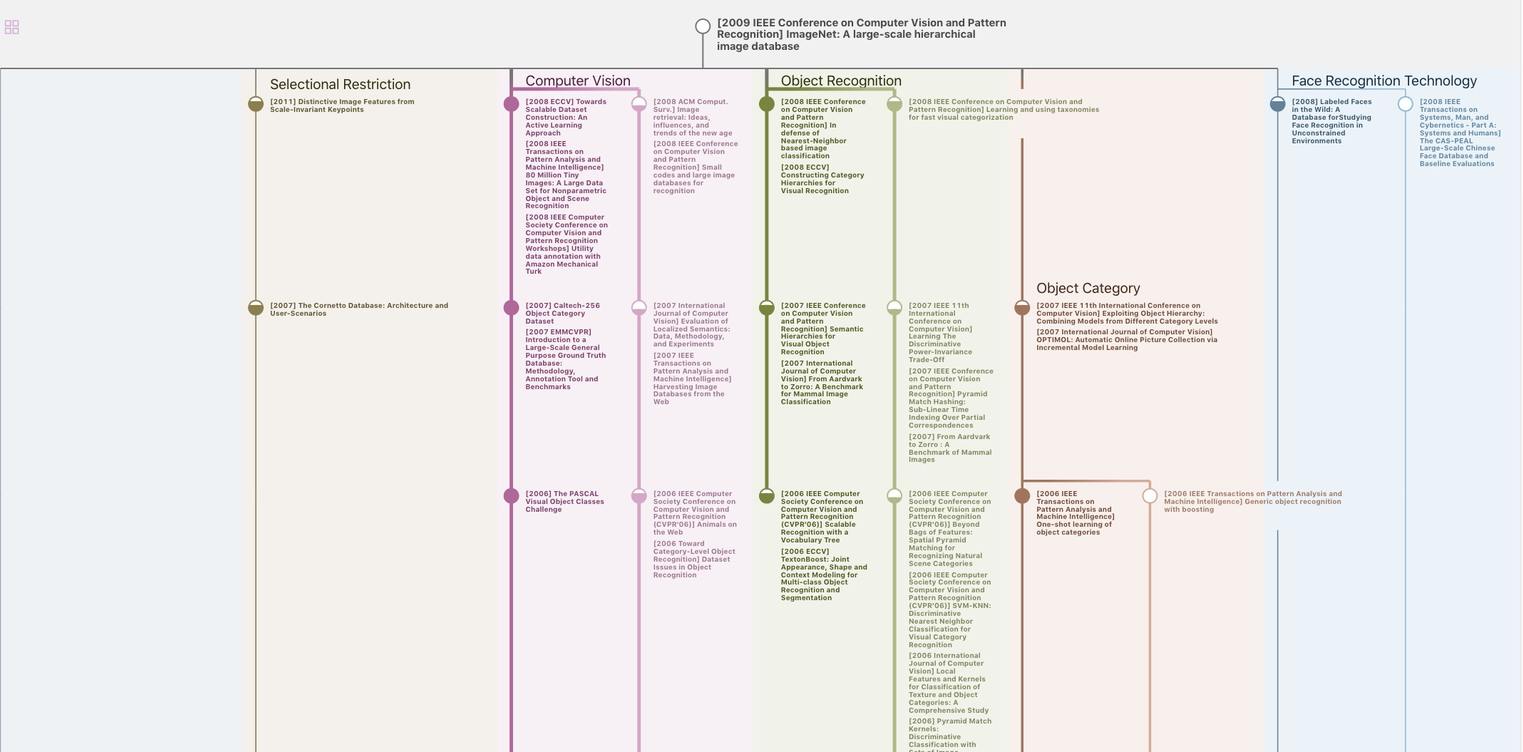
生成溯源树,研究论文发展脉络
Chat Paper
正在生成论文摘要