Robust Variational Autoencoders and Normalizing Flows for Unsupervised Network Anomaly Detection
Advanced Information Networking and Applications(2022)
摘要
In recent years, the integration of connected devices in smart homes has significantly increased, thanks to the advent of the Internet of things (IoT). However, these IoT devices introduce new security challenges, since any anomalous behavior has a serious impact on the whole network. Network anomaly detection has always been of considerable interest for every actor in the network landscape. In this paper, we propose GRAnD, an algorithm for unsupervised anomaly detection. Based on Variational Autoencorders and Normalizing Flows, GRAnD learns from network traffic metadata a normal profile representing the expected nominal behavior of the network. Then, this model is optimized to detect anomalies. Unlike existing anomaly detectors, our method is robust to the hyperparameter selection and outliers contaminating the training data. Extensive experiments and sensitivity analyses on public network traffic benchmark datasets demonstrate the effectiveness of our approach in network anomaly detection.
更多查看译文
关键词
Unsupervised anomaly detection, Robust autoencoders, Dynamic outlier filtering, Network traffic anomaly detection
AI 理解论文
溯源树
样例
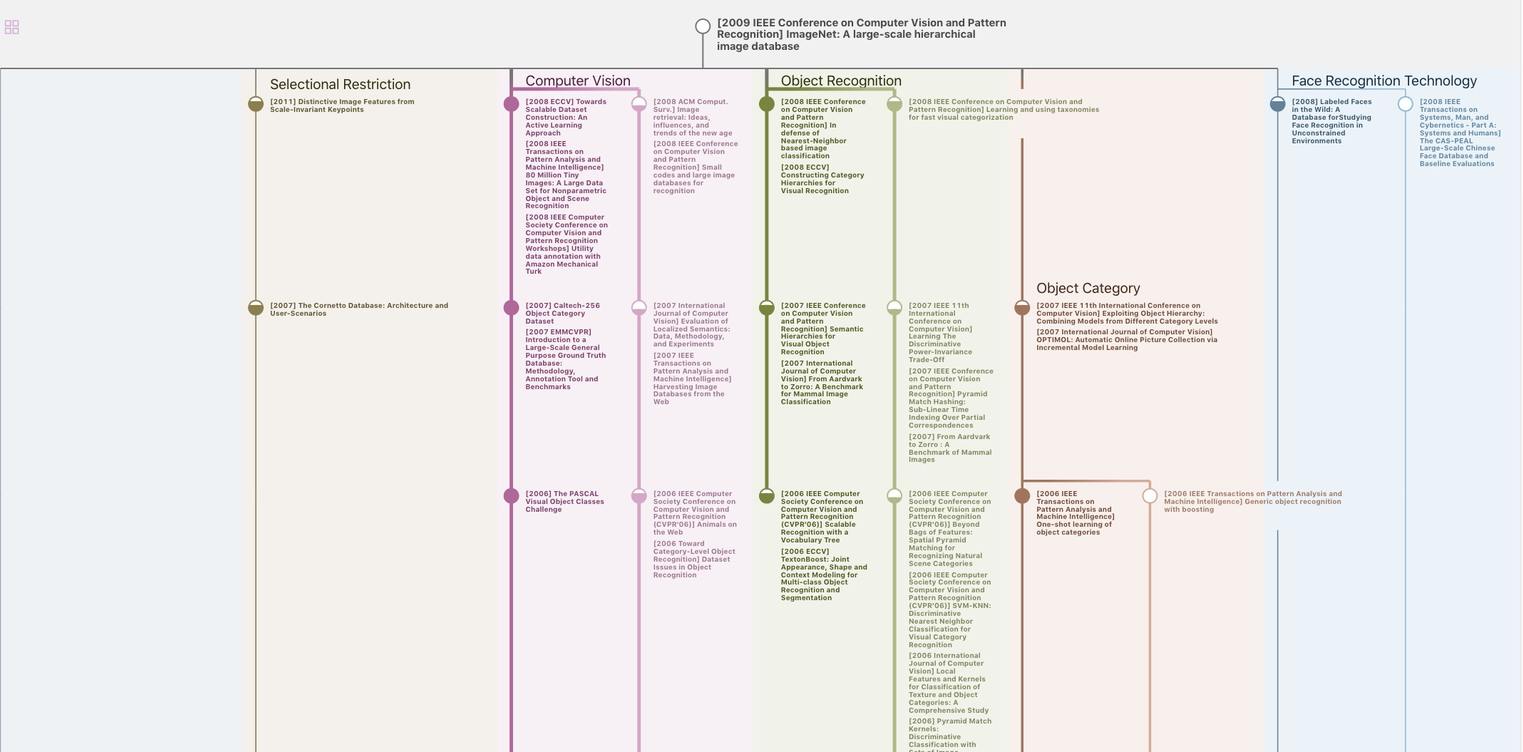
生成溯源树,研究论文发展脉络
Chat Paper
正在生成论文摘要